AI in Debt Collection: Revolutionizing Recovery Strategies
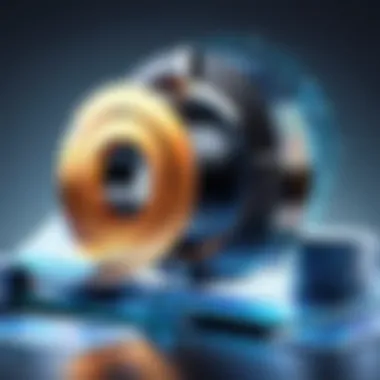
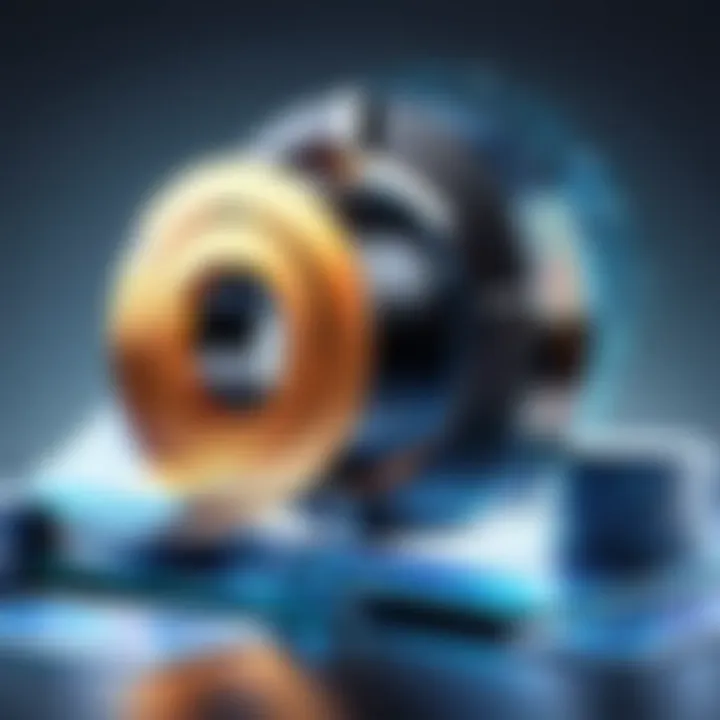
Intro
The evolution of debt collection is increasingly influenced by the rapid advancements in artificial intelligence (AI). As financial technologies evolve, businesses must adapt their recovery processes to leverage the benefits that AI offers. In the competitive landscape of debt recovery, organizations are exploring ways to enhance efficiency and improve customer engagement. This article will delve into various aspects of AI's role in debt collection, including technological integration, benefits, challenges, regulatory considerations, and future trends in the industry.
Understanding these dynamics is crucial for stakeholders aiming to optimize their debt recovery procedures while ensuring compliance and fostering better customer relations.
Software Overview
AI-driven debt collection software has become an essential tool for many organizations. As they implement these solutions, it is important to comprehend the key features and system requirements associated with such software.
Key Features
- Automated Communication: Uses AI chatbots or automated systems for interaction with debtors, ensuring timely responses while reducing human error.
- Data Analytics: Tools analyze vast datasets to identify trends, predict debtor behavior, and optimize recovery strategies.
- Risk Assessment: Employs algorithms to evaluate the likelihood of successful debt recovery, focusing resources more effectively.
- Personalized Approaches: AI enables tailored communication strategies based on individual debtor profiles, enhancing engagement rates.
- Seamless Integration: These systems can often be integrated with existing CRM and ERP software to streamline operations.
System Requirements
To successfully operate AI debt collection software, organizations need to consider several technical specifications. This may include:
- Hardware Requirements: Adequate processing power and memory to handle data processing tasks.
- Software Requirements: Compatible operating systems and any necessary third-party applications for optimal performance.
- Data Security Measures: Strong security protocols to safeguard sensitive debtor information.
- User Access Control: Robust systems to manage user access and maintain data integrity.
In-Depth Analysis
As businesses assess AI tools for debt recovery, a critical analysis of performance and usability becomes essential. This section will explore how these systems function in practice.
Performance and Usability
Many organizations have reported marked improvements in performance since adopting AI-driven debt collection methods.
- Efficiency: Tasks that previously required human intervention can now be automated, leading to a significant reduction in operational time.
- Accuracy: AI systems minimize errors that could occur due to manual processing, ensuring that communications and tracking are accurate.
- Accessibility: Intuitive interfaces make it easier for staff to utilize the software efficiently, thereby fostering its adoption across teams.
Best Use Cases
AI applications within debt collection can greatly vary depending on specific business needs. Some prominent use cases include:
- Small Businesses: Automating follow-ups on overdue invoices while maintaining personalized communication.
- Large Corporations: Analyzing debtor trends to optimize collection strategies and reduce default rates.
- Financial Institutions: Employing AI for risk assessments and improving compliance with regulatory requirements.
The implementation of AI in debt collection simplifies processes, allowing companies to focus on high-priority tasks while improving recovery rates.
Leveraging these insights can help organizations navigate the complexities of debt recovery in a changing technological landscape.
Understanding AI in Debt Collection
The introduction of artificial intelligence into debt collection processes marks a significant development in the financial technology sector. Understanding AI in debt collection means recognizing its potential to improve efficiency, accuracy, and overall effectiveness in recovering debts. This section will delve into the subtle nuances of this relationship, offering insights into how AI reshapes the recovery landscape.
The Concept of Debt Collection
Debt collection is the process of pursuing payments of debts owed by individuals or businesses. This function is vital for maintaining cash flow and financial health for companies in myriad industries. In an era characterized by rising amounts of debt, effective debt collection has become more critical than ever. Traditional methods often included calls and letters that had little success in engaging debtors.
AI technology has changed the way debt collection occurs. Automated systems can analyze data more efficiently than humans ever could. This means better targeting of collections strategies and faster resolution of debt issues. Furthermore, by utilizing technology, the debtor’s experience can be transformed. Understanding how debt collection operates is essential to grasping the importance of AI's role in enhancing these processes.
Defining Artificial Intelligence
Artificial intelligence refers to the simulation of human intelligence by computer systems. This encompasses various capabilities like learning, reasoning, and self-correction. There are several types of AI, including narrow AI, which is designed to perform a narrow task, and general AI, which can understand and reason in a broad sense similar to a human. In debt collection, narrow AI is predominantly utilized. It provides automated responses, analyzes payment patterns, and predicts behaviour of debtors. By comprehending what constitutes AI, stakeholders can better understand its application in specific areas such as debt collection.
Linking AI with Debt Collection
The link between AI and debt collection manifests through various applications. AI can automate routine tasks, such as sending reminders and notices. This frees up human agents to handle more complex interactions that require emotional intelligence. Additionally, AI tools can analyze vast datasets to forecast payment behaviours and identify the most efficient strategies for each debtor demographic.
AI-driven analytics tools utilize machine learning to refine their algorithms continually. This adaptation enhances their effectiveness over time. The combination of AI with debt collection processes can ultimately lead to higher recovery rates and reduced operational costs. Therefore, understanding this connection is crucial for businesses looking to optimize their debt collection practices.
"The integration of AI in debt collection enables businesses to operate not only more efficiently but also with greater empathy towards the debtor's experience."
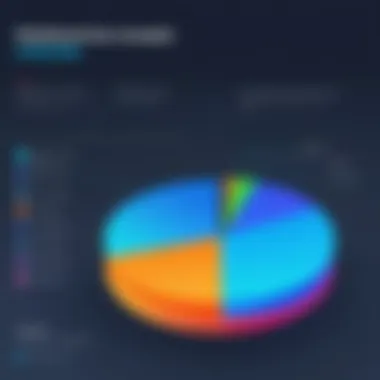
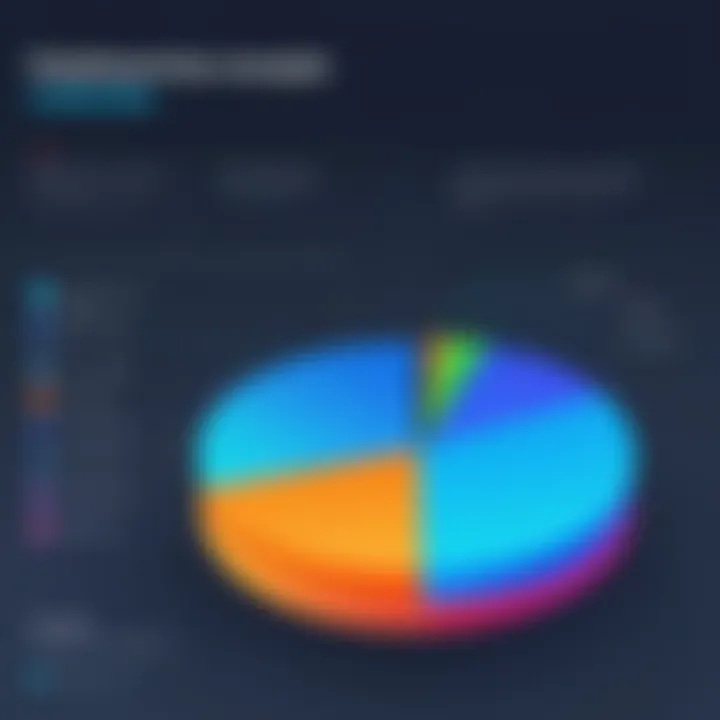
Applications of AI in Debt Collection
The application of artificial intelligence in debt collection has emerged as a transformative phase in modern financial recovery practices. Leveraging AI can streamline processes, enhance decision-making, and ultimately improve outcomes for businesses and their clients. For organizations in the debt collection sector, understanding the various applications of AI is essential for optimizing their strategies and staying competitive. This section will delve into specific applications and their impact on operational efficiency.
Automated Communication Systems
Automated communication systems represent a significant advancement in how debt collectors interact with clients. With AI-driven tools like chatbots and automated messaging, companies can maintain continuous engagement with debtors. These systems can manage responses, answer frequently asked questions, and provide timely updates to clients regarding their accounts. This increases efficiency, allowing human agents to focus on more complex cases that require personal attention.
Implementing these systems can reduce operational costs and guarantee that communication occurs consistently. By utilizing Natural Language Processing (NLP), automated systems can understand and respond to inquiries in a manner that feels conversational, reducing frustration for the debtor.
In many scenarios, the automation of communication not only eases the burden on collections professionals but also enhances customer satisfaction by offering immediate responses. As a result, faster resolutions potentially lead to better recovery rates.
Predictive Analytics for Recovery Rates
Predictive analytics is another pivotal application of AI in debt collection. By analyzing historical data, such as payment patterns and demographic information, AI algorithms can effectively forecast the likelihood of successful debt recovery from certain clients. Organizations can use this information to prioritize their efforts, focusing resources on accounts that are more likely to yield returns.
This approach optimizes the loan collection strategy. Companies can tailor their communication strategies, adapting messages and outreach based on predictive outcomes. For example, if predictive models indicate that a segment of clients tends to respond favorably to email reminders rather than phone calls, resources can be allocated accordingly to maximize recovery rates.
Moreover, implementing predictive analytics supports better risk management by helping businesses develop informed strategies. This proactive approach can significantly improve efficiency and effectiveness in recovery operations.
Enhanced Payment Processing Techniques
Artificial intelligence also plays a critical role in enhancing payment processing techniques. By automating functions such as account verification, payment authentication, and transaction tracking, organizations can reduce human error and speed up the payment cycle.
AI systems can analyze various payment methods and develop insights into which methods are most reliable and preferred by customers. This can streamline options and improve compliance with regulations concerning secure payment processing.
For instance, intelligent systems might flag suspicious or potentially fraudulent transactions, minimizing losses for businesses. By utilizing machine learning algorithms, payment processing can become more adaptable to trends, ensuring that businesses stay ahead in the evolving landscape of digital finance.
In summary, the applications of AI in debt collection are vast and varied, ranging from enhancing communication to optimizing recovery strategies. As organizations adopt these advanced techniques, they not only improve operational efficiency but also ensure a more satisfactory experience for both businesses and clients.
Benefits of AI in Debt Collection
The integration of artificial intelligence in debt collection offers several significant benefits that markedly improve traditional recovery processes. As the landscape of finance evolves, understanding these advantages is essential for any organization looking to enhance their operations. The core benefits include improved efficiency and speed, cost-effectiveness in operations, and higher recovery ratios. Each of these elements plays a vital role in optimizing the debt collection process, ensuring both compliance and better customer interactions.
Improved Efficiency and Speed
Improving efficiency is critical in any business, especially in the collection of debts. AI technologies streamline the entire recovery process. With tools like chatbots and automated communication systems, organizations can respond to client inquiries and reminders much faster than human teams. This quick response time not only saves operational time but also enhances the overall customer experience.
Data analysis further boosts efficiency. AI systems can analyze vast amounts of customer data swiftly, pinpointing debts that are most likely to be recovered. This capability allows debt collectors to prioritize their efforts on high-potential accounts, which increases overall recovery rates. Additionally, the ability to segment audiences based on behavior or payment history means tailored strategies can be applied effectively. Therefore, businesses can expect a noticeable reduction in the time frames typically associated with debt recovery.
Cost-Effectiveness in Operations
Cost considerations are always at the forefront of business decisions. AI contributes to cost-effectiveness in debt collection through minimized operational expenses. Automating repetitive tasks lessens the need for a large workforce, which can lead to significant cost savings.
For instance, using AI-driven tools for generating payment reminders eliminates the need for manual intervention. This not only cuts down on labor costs but also reduces error rates associated with human involvement. Furthermore, AI allows companies to manage multiple customer interactions simultaneously. In contrast, a human team would struggle to handle a similar volume without sacrificing quality. Overall, implementing AI translates into lower costs and higher productivity, making it possible to allocate resources to other important areas of the business.
Higher Recovery Ratios
The ultimate goal of debt collection is to recover as much debt as possible, and this is where AI shines. With its predictive analytics capabilities, AI can forecast the likelihood of recovery against various factors, helping businesses make informed decisions. Enhanced data analysis leads to identifying optimal collection strategies tailored to specific customer segments, thereby increasing the chance of recovery.
By utilizing machine learning algorithms, AI continually improves its accuracy in predicting recovery outcomes. This approach has shown that firms using AI technologies report significantly higher recovery ratios. A study indicated that companies that deployed AI solutions saw improvements in collections as much as 30%. As businesses look to maximize their recovery efforts, AI presents a compelling case for its role in enhancing ratios and driving better outcomes.
"AI can positively affect the efficiency and effectiveness of debt collections, allowing quicker resolutions and reduced costs."
In summary, the benefits of AI in debt collection reveal its transformative potential. By focusing on improving efficiency and speed, cost-effectiveness, and recovery ratios, organizations can redefine their approach to debt recovery. These advantages not only impact the bottom line but also enhance customer relationships, which are equally important in today’s competitive marketplace.
Challenges of Implementing AI in Debt Collection
Integrating artificial intelligence into debt collection might seem beneficial, yet it comes with challenges. Understanding these challenges is crucial for stakeholders. They must navigate pitfalls to effectively implement AI solutions. The potential risks can hinder productivity and lead to legal issues if overlooked. Exploring these specific challenges leads to better preparedness in effectively leveraging AI.
Data Privacy Concerns
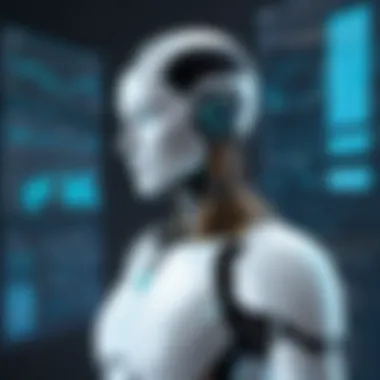
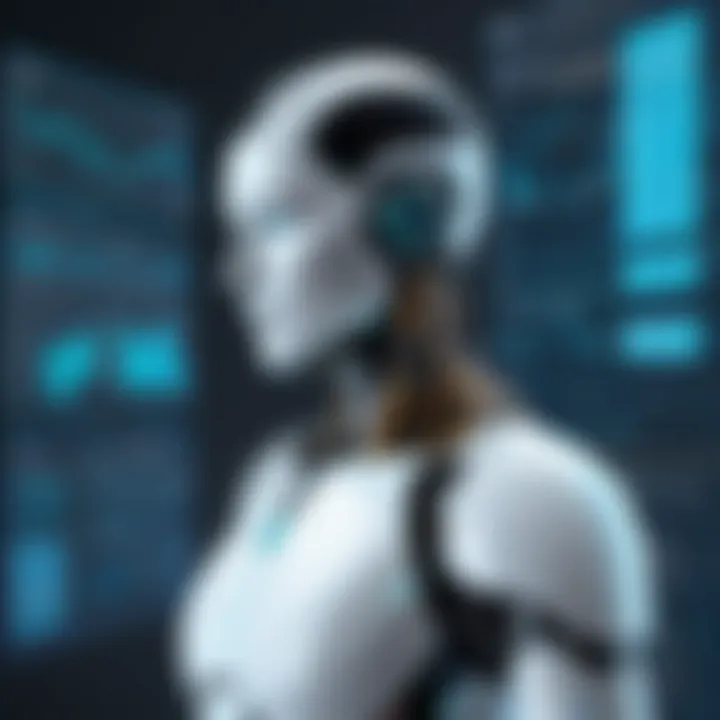
Data privacy forms a significant barrier when deploying AI in debt collection. Organizations must manage sensitive financial information cautiously. Under regulations such as GDPR, failing to protect collected data can lead to severe penalties. Stakeholders must develop strategies for secure data handling. This requires robust encryption methods and frequent audits of data access. The trade-off between efficient AI use and privacy concerns necessitates clear guidelines.
Moreover, customers expect transparency regarding their information use. Maintaining trust hinges on clear communication about data handling practices. Organizations failing to address these concerns risk losing customer confidence, negatively impacting recovery rates.
Technological Compatibility Issues
Adopting AI tools often exposes compatibility issues with existing systems. Organizations may have legacy systems that are not designed for modern technology. Integrating these systems with AI can be complex and costly. Investing in new technology often becomes necessary, leading to budget strains.
It is crucial for organizations to assess existing infrastructures before implementing AI. Understanding integration requirements is essential to avoid disruptions. Seamless compatibility extends productivity as operations remain efficient.
Potential Bias in AI Algorithms
Bias in AI algorithms is another significant obstacle. AI learns from historical data, which may contain biases. Ignoring these biases can lead to unfair treatment of customers. For instance, an automated system may classify certain demographics as higher risk based on biased historical data.
Awareness of this potential bias is vital. Continuous monitoring and evaluation of AI models help reveal and mitigate these biases. Organizations must prioritize fairness in their AI systems to build trust among customers. Making informed decisions based on unbiased data elevates recovery rates and maintains compliance obligations.
"Addressing challenges in AI debt collection is not a choice; it is a necessity for success."
Regulatory Framework Surrounding AI and Debt Collection
The regulatory framework for AI in debt collection is crucial. As businesses increasingly rely on artificial intelligence, compliance with legal standards becomes paramount. Understanding these regulations helps companies navigate potential legal pitfalls. This section breaks down the key elements surrounding compliance and the implications of various laws on AI practices in debt collection.
Understanding Compliance Requirements
First, compliance requirements are essential for technology implementation. This includes adhering to data protection laws, which ensure that consumer data is collected, stored, and used responsibly. For instance, firms must comply with the Fair Debt Collection Practices Act (FDCPA) in the United States. This act mandates transparency and fairness in debt collection efforts. Failing to meet these criteria can lead to legal penalties and damage to a company’s reputation.
In addition, companies should regularly review their procedures to align with evolving regulations. Regular audits can help maintain compliance. It's also beneficial to train staff on these requirements, fostering a culture of adherence within the organization. Good practices can mitigate risks associated with AI use in debt collection.
Impact of GDPR on AI Practices
The General Data Protection Regulation (GDPR) has substantial influence on how AI is applied in debt collection, particularly in Europe. The GDPR sets strict guidelines for data handling, focusing on privacy and consumer rights. Companies need explicit consent before processing personal data, which affects how debt collectors utilize AI to analyze consumer behavior.
Under GDPR, individuals have the right to access their data and request deletion. This means that AI systems must be designed to allow such processes. Implementing GDPR-compliant AI practices can be a competitive advantage, as consumers are increasingly aware of their data rights.
Future Regulatory Trends
As technology evolves, so does the regulatory landscape. Future trends may include more stringent regulations based on advancements in AI technologies. Policymakers will likely focus on protecting consumer rights amid the rapid adoption of AI. This could result in specific guidelines aimed at algorithmic transparency, ensuring that AI decision-making processes are understandable and accountable.
In addition, cross-border data flows may attract more regulations as companies expand their operations internationally. Understanding these potential shifts is vital for organizations using AI in debt collection.
"Adapting to regulatory changes is not just about compliance; it's about building trust and credibility with consumers."
As the landscape shifts, companies must remain proactive in understanding and adapting to the regulatory framework surrounding AI and debt collection. Continual education and adaptation will be key to thriving in this rapidly evolving space.
Integration of AI with Existing Debt Collection Software
Integrating artificial intelligence with existing debt collection software is an essential step for optimizing the recovery process. As businesses seek to enhance their operations, the role of AI becomes increasingly significant. AI technologies can analyze data more effectively than traditional methods, allowing for improved decision-making and faster response times. This integration not only streamlines processes but also enhances customer interactions.
Key Benefits of Integration
- Increased Efficiency: AI can manage large volumes of data quickly, allowing debt collectors to focus on more complex cases. By automating routine tasks, time can be reallocated to strategic decision-making.
- Enhanced Accuracy: AI tools can analyze past data trends, reducing human errors in debt collection. This leads to more accurate predictions regarding payment behaviors and anomalies.
- Better Customer Engagement: AI can help tailor communication strategies based on customer behavior. This results in more personalized outreach that can improve response rates and relationships with clients.
However, the integration involves careful planning and consideration of different factors. Organizations need to evaluate their current systems and identify what specific AI tools will enhance their operations.
Choosing the Right AI Tools
Selecting the appropriate AI tools is critical for any debt collection agency looking to integrate AI into their existing software. Not all tools may fit every organization’s needs. It is vital to assess the type of data you collect and how AI can analyze it.
Here are some points to consider:
- Functionality: Choose tools that offer features aligned with your operational goals. Look for capabilities such as predictive analytics or automated communication.
- Scalability: The selected tools should be able to grow with your business. Flexibility is essential for adapting to future demands.
- User-Friendliness: The tools must be easy for staff to use. Training is important, but usability is highly beneficial for quick adoption.
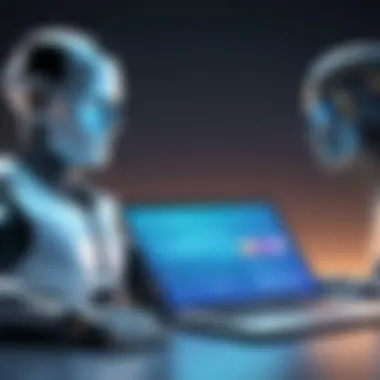
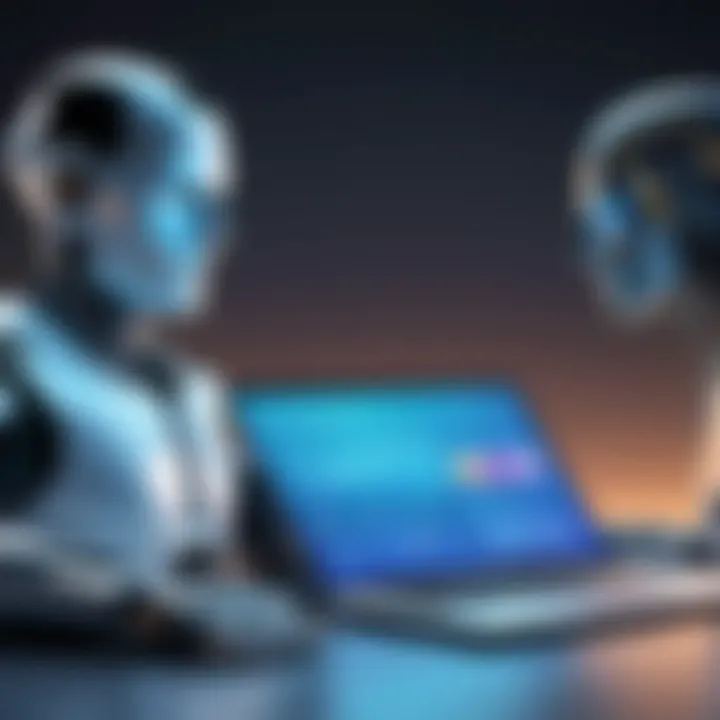
Seamless Integration Techniques
To ensure that AI tools work effectively, seamless integration techniques must be employed. A fragmented approach can lead to inefficiencies and poor data management. Here are some effective techniques:
- API Connectivity: Utilize application programming interfaces (APIs) for smooth data exchange between AI tools and existing software. APIs help maintain data integrity.
- Testing and Iteration: Before full implementation, conduct pilot tests to identify any issues. Iterative testing allows for adjustments before full-scale deployment.
- User Training: Proper training for staff is paramount. They should understand both the functionality of the new tools and how they fit into existing workflows.
Evaluating Performance Post-Integration
After integrating AI with debt collection software, evaluation of its performance is crucial. Organizations should track specific metrics to assess effectiveness.
- Debt Recovery Rates: Analyze if there has been an increase in recovery rates post-integration. Improved rates often signal that the integration has been successful.
- Time Efficiency: Measure how much time is saved with the new system. Time efficiency leads directly to cost savings.
- Customer Feedback: Collect feedback from customers regarding their experience with personalized communications. Positive feedback indicates successful engagement.
"The integration of AI in debt collection is not just about technology; it’s about reshaping the relationship between collectors and clients."
Case Studies: Successful AI Implementations in Debt Collection
In the rapidly changing landscape of debt collection, the adoption of artificial intelligence has emerged as a game changer. Case studies illustrating successful AI implementations help to solidify the understanding of its practical benefits and potential pitfalls in the debt recovery process. They showcase real-world examples where AI has enhanced collection strategies, optimized resource allocation, and fostered a more personalized customer experience. Learning from these case studies is essential for industry professionals seeking to harness AI effectively.
Examining different sectors can highlight specific applications of AI technology. Solutions that work for one industry might provide insights or inspiration for another. Thus, such studies foster cross-sector collaborations and broaden the horizons for AI utilization in debt recovery.
Industry-Specific Examples
Across industries, there are notable examples that illustrate the successful implementation of AI in debt collection. For instance, a leading telecommunications company integrated AI solutions to manage overdue accounts. They automated communication processes, making it easier to reach out to customers with personalized messages. As a result, they reduced their overdue debts by 30% within the first year.
Similarly, a major financial services firm utilized predictive analytics to assess the likelihood of a customer's ability to repay debts based on their previous behavior. This approach helped them prioritize their outreach efforts and tailor repayment plans efficiently, resulting in a 25% increase in the recovery rate compared to traditional methods.
The healthcare industry also benefits from AI-driven debt collection. Hospitals that adopted AI chatbots for interactions with patients for bill collection noted a significant increase in engagement rates. Patients were more likely to respond to AI-generated reminders and inquiries than to human calls, thus leading to more timely payments.
Measurable Outcomes and Success Metrics
Evaluating the impact of AI in debt collection requires clear success metrics. A thorough analysis of these implementations has revealed substantial measurable outcomes.
- Increased Recovery Rates: Companies reported recovery rate increases ranging from 20% to 40% after introducing AI solutions. This significant improvement highlights AI's ability to target debtors effectively and maintain engagement.
- Reduced Operational Costs: Businesses have observed up to a 50% reduction in operational costs directly related to debt collection activities. Automating routine tasks reduces the need for extensive call teams, allowing firms to allocate resources elsewhere.
- Improved Customer Satisfaction: AI implementations have resulted in higher customer satisfaction scores. By providing timely, relevant communication and offering personalized repayment options, companies have improved their brand image and customer loyalty.
- Shortened Collection Cycles: Case studies indicate that companies leveraging AI technologies have shortened their collection cycles by up to 30%. This faster turnaround not only enhances cash flow but also reduces the time accounts remain in arrears.
In summary, case studies of successful AI implementations in debt collection are invaluable resources. They offer insights into how tailored AI solutions can enhance recovery processes. By adopting proven strategies and learning from others, organizations can position themselves favorably in the competitive debt collection landscape.
The Future of AI in Debt Collection
As industries evolve with the emergence of new technologies, the debt collection sector faces significant transformations through the integration of artificial intelligence. The future of AI in debt collection is not merely a continuation of existing practices; it represents a fundamental shift in how organizations manage recovery processes. There is a growing recognition of the need to adapt to these changes to stay competitive while meeting the demands of consumers.
The incorporation of AI technologies holds immense potential for improving the efficiency of debt recovery. Benefits like enhanced customer interactions and streamlined operations can discriminate proactive firms from those struggling with traditional methods. Given the competitive nature of the industry, understanding the future dynamics is vital for businesses that aim to thrive.
While the prospects are promising, organizations must also navigate the complexities and considerations that come with AI implementation. Factors such as technology cost, training, and compliance must be addressed to extract maximum value from AI systems.
Emerging Technologies and Trends
Several emerging technologies are paving the way for advancements in debt collection. One notable trend is the integration of chatbots and virtual assistants. These tools are capable of handling routine inquiries, offering customers a swift means of communicating without burdening human agents. This efficiency reduces overhead costs and enhances the consumer experience, driving higher customer satisfaction rates.
Moreover, the adoption of blockchain technology can revolutionize record-keeping and transactions within debt collection. Secure, transparent ledgers can minimize disputes, which can often lead to delays in the recovery process. It offers a potential solution to enhance trust and reliability between debtors and creditors.
Key Trends to Watch:
- Automation: Continuous improvements in robotic process automation (RPA), leading to more tasks being performed by AI.
- Data Analytics: Enhanced predictive analysis tools for better decision-making regarding recovery strategies.
- Omni-channel Communication: Creating seamless communication paths through various platforms to optimize customer engagement.
The Role of Machine Learning
Machine learning is a pivotal component of AI's future in debt collection. This technology allows systems to analyze vast amounts of data to predict outcomes more precisely than traditional methods. AI algorithms can assess a debtor's payment history, behavior patterns, and various other factors to make informed decisions about when and how to initiate contact or establish repayment plans.
As a result, companies can personalize their outreach strategies. Tailoring methods to individual cases can lead to improved collection rates. Furthermore, AI's capability to learn from past interactions means it can continually improve its strategies over time, making organizations smarter in their collection efforts.
Potential Ethical Considerations
As AI integrates more deeply into debt collection operations, ethical considerations must be a priority. Issues surrounding data privacy and consent are paramount. Organizations must ensure they comply with regulations such as GDPR when collecting and processing user data. Misuse of data can lead to significant reputational damage and legal repercussions.
Another ethical issue is ensuring that AI systems do not lead to biased outcomes, potentially impacting vulnerable customers unfairly. AI models are only as good as the data they are trained on. If not carefully managed, discrepancies in data can perpetuate discrimination.
"Responsible AI use must be a priority, not an afterthought, to maintain integrity and fairness in debt collection."