AI for Pricing: Redefining Pricing Strategies
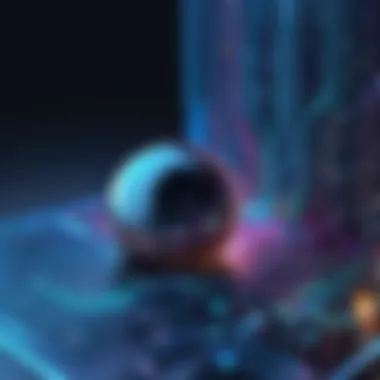
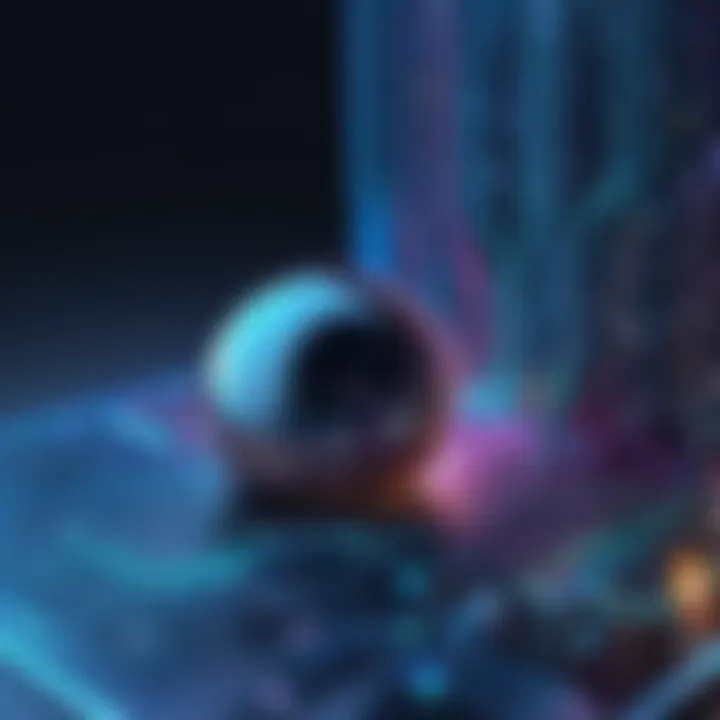
Intro
Artificial intelligence has made significant strides in various fields, but one area where it shines most vividly is in pricing strategies. Pricing is no longer just about setting a number; it's a complex web of data, analytics, and human behavior. The intersection of AI and pricing is creating waves, enabling businesses to tailor their pricing models in real-time, enhance customer experience, and maximize profits.
In today’s market, understanding how to leverage AI for pricing is crucial for any organization aiming to maintain a competitive edge. Consumers expect personalized experiences and dynamic pricing that reflects their preferences and behaviors. As a result, organizations face the challenge of navigating a landscape that's not only influenced by market trends but also by rapidly evolving technology.
This article aims to unpack the essential elements of AI in pricing strategies. From discussing the various tools available to delving into real-world applications and ethical considerations, we will shed light on how artificial intelligence is transforming this crucial aspect of business strategy.
Software Overview
When diving into AI-driven pricing strategies, the software tools that power these solutions come to the forefront. Understanding their capabilities is key to harnessing the full potential of artificial intelligence in pricing.
Key Features
- Dynamic Pricing Algorithms: These algorithms analyze data such as demand fluctuations, competitor prices, and consumer behaviors to adjust prices instantaneously.
- Predictive Analytics: Leveraging historical data, predictive analytics can forecast price trends and consumer behavior patterns, allowing businesses to make proactive pricing decisions.
- Market Basket Analysis: This feature helps identify the relationship between products, enabling businesses to optimize pricing based on item bundles or cross-sell opportunities.
- User-friendly Dashboards: Intuitive visualizations help businesses understand pricing impacts and outcomes, making complex data easy to digest.
- Integration Capabilities with Existing Systems: Seamless integration with CRM, ERP, and inventory systems allows for more cohesive pricing strategies across the organization.
System Requirements
Employing AI-driven pricing solutions typically requires robust hardware and modern software environments. Generally, a few common requirements include:
- Operating System: Windows, Linux, or macOS (latest versions recommended)
- Processor: Multi-core processors such as Intel i5/i7 or AMD Ryzen
- RAM: Minimum 16GB for optimal performance
- Storage: SSD with at least 500 GB for fast data retrieval
- Additional Software: Latest versions of statistical and analytics tools, such as R or Python libraries.
With a firm understanding of the software components, it’s time to delve deeper into how these technologies affect performance, usability, and their most appropriate use cases.
Prologue to AI in Pricing
Artificial Intelligence (AI) has become a game changer when it comes to pricing strategies in various industries. With businesses facing tight margins and the pressure to adapt to rapidly shifting consumer preferences, AI offers a way to navigate through these turbulent waters. The integration of AI in pricing isn’t just a new trend; it's fundamentally changing how companies approach pricing decisions, allowing for greater precision and responsiveness.
Understanding AI in pricing involves looking at how algorithms utilize data to inform pricing strategies. This is not merely about setting prices; it encompasses a range of methodologies that analyze consumer behavior, competitor pricing, market trends, and much more. For example, AI can help determine the optimal price for a product based on real-time market conditions, often leading to increased revenue and improved profit margins.
Benefits of AI in Pricing
- Enhanced Decision-Making: AI provides valuable insights that enable companies to make data-driven decisions, minimizing guesswork.
- Dynamic Adjustments: Prices can be adjusted rapidly to reflect changes in consumer demand or competitor pricing, allowing businesses to stay ahead.
- Personalization: AI applications can tailor specific offers to different customer segments based on their purchasing history and preferences, thereby increasing conversion rates.
This section introduces key considerations when embracing AI in pricing strategies. The importance of having quality data cannot be overstated; poor data quality can lead to misguided decisions. Similarly, businesses must consider the need for transparency in pricing algorithms to build consumer trust.
"AI in pricing not only optimizes being cost-effective but also enhances the customer experience by ensuring patrons perceive value in what they pay."
As we delve deeper into AI’s role in pricing strategies, we’ll investigate its practical applications, limitations, and implications for the future. The interconnectedness of AI technology and pricing strategies indicates this is just the beginning of a significant transformation in how businesses operate in competitive markets.
Definition of AI in Pricing
AI in pricing refers to the application of advanced computational methods and algorithms to analyze vast amounts of data for the purpose of determining optimal pricing structures. This involves using techniques from machine learning and data analytics to predict how consumers will respond to various price points, thereby influencing demand and profitability.
For instance, many retail giants utilize AI tools to set prices based on customer interactions on their websites or through previous purchase behaviors. This is not just about discounting; it's about strategically positioning products in a way that maximizes revenue while ensuring customer satisfaction. By leveraging AI, companies can create pricing strategies that adapt in real-time, thus responding to both market fluctuations and customer behaviors.
Historical Context
The application of technology in pricing is not new. However, the integration of AI intensifies this evolution. Historically, pricing strategies often relied on intuition or static models based on historical data. Businesses adjusted their prices periodically, sometimes at the expense of missing fleeting market opportunities or failing to address shifts in consumer sentiment.
In the late 20th Century, advanced analytics began to play a role, and progressively more businesses adopted data analytics for pricing. It was around the early 2000s that the emergence of machine learning began shifting the landscape. Algorithms, capable of processing far more data than a human analyst, started to provide predictive insights based on past consumer behavior and market dynamics. Companies like Amazon and Walmart were early adopters of these technologies, using sophisticated algorithms to adjust prices instantaneously based on a multitude of factors, including inventory levels and competitor pricing.
Today, AI stands at the forefront, with tools and platforms specifically designed for price optimization becoming available to businesses of all sizes. The use of AI in pricing has transitioned from a competitive advantage of the few to a necessity for survival in many markets. As a result, companies are forced to continuously innovate and adapt, shaping the future landscape of pricing strategies.
The Role of AI in Modern Pricing Strategies
Artificial Intelligence has become increasingly prominent in contemporary pricing strategies, fundamentally altering the way businesses determine their prices. The infusion of AI into pricing practices brings about a host of advantages, enabling companies to respond more adeptly to market fluctuations and consumer behavior. This is especially vital in an era characterized by rapid change; businesses can no longer afford to base their pricing decisions solely on historical data or simplistic heuristics. By leveraging AI, organizations can harness data analytics to develop smarter, more agile pricing frameworks.
A key benefit of utilizing AI in pricing strategies is its ability to adopt a data-driven approach. With advanced algorithms and machine learning capabilities, AI analyzes vast amounts of data from various sources in real-time, turning insight into action. For instance, retailers can monitor competitor pricing, track inventory levels, and assess consumer demand all at once. Consequently, they can identify trends and optimize prices accordingly, ensuring they avoid losing out on potential revenue.
Moreover, implementing AI in pricing strategies allows businesses to personalize offers for their customers. It enables organizations to tailor pricing based on individual consumer preferences, shopping habits, and demographic information. This not only enhances customer satisfaction but also drives loyalty. In highly competitive markets, where every dollar counts, the ability to provide personalized pricing is a game changer.
"AI helps businesses to not just set prices – it allows them to rethink their pricing strategies entirely, aligning closely with market dynamics and consumer expectations."
In sum, AI is transforming the landscape of pricing strategies. By enabling dynamic responses to market changes and facilitating customized pricing, AI stands to deliver substantial benefits to businesses looking to thrive in today's fast-paced environment. The next crucial step explores dynamic pricing models, a pivotal application of AI in modern pricing.
Dynamic Pricing Models
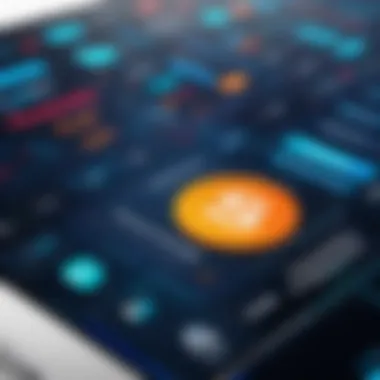
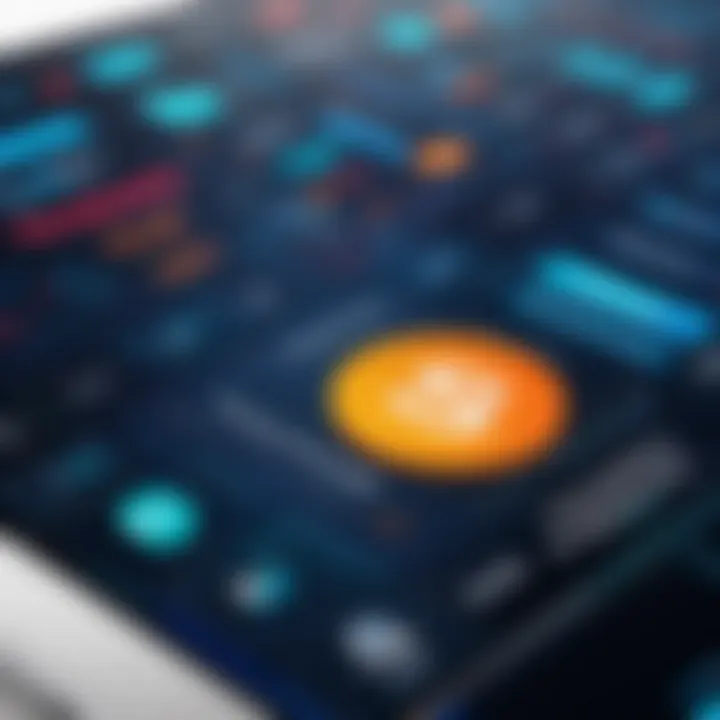
Dynamic pricing models are at the forefront of AI-driven pricing strategies. This method involves adjusting prices in real-time based on market conditions, competitor actions, and other key variables. For example, airlines often utilize dynamic pricing. When a flight's demand spikes, the ticket prices increase correspondingly. Conversely, if the demand lags, prices might drop to fill seats.
Price Optimization Techniques
Another essential aspect of AI in pricing is price optimization techniques. These methods are designed to find the best possible price point for a product, maximizing both sales and profits. Here, businesses account for various factors like production costs, competitive pricing, and consumer purchasing behavior.
To implement price optimization effectively, organizations may use A/B testing. This involves setting two different prices for the same product to identify which generates higher sales. Advanced analytics algorithms can assess performance metrics to ensure that the pricing strategy aligns with the overarching business goals.
Additionally, AI can vary pricing based on customer segments. For instance, data shows that young consumers may be more inclined to purchase a product at a lower price compared to older demographics. Targeting specific segments with appropriate pricing can increase conversion rates significantly.
Machine Learning and Its Applications in Pricing
Machine learning has become a pivotal element in modern pricing strategies. Its ability to analyze vast datasets and uncover patterns that humans might overlook provides companies with a critical edge. The incorporation of machine learning not only enhances the accuracy of pricing but also aids businesses in adapting to rapidly changing market conditions. By utilizing complex algorithms, organizations can forecast demand more effectively, optimize pricing in real-time, and ultimately drive profitability.
Supervised Learning Approaches
When we talk about supervised learning in the pricing context, it often involves algorithms that learn from historical data. Essentially, these algorithms require a labeled dataset where the input features are paired with the correct outputs. For instance, a retailer might use past sales data, product features, and market conditions to train a model that predicts the optimal price for a new product launch.
The benefits of supervised learning in pricing are manifold:
- Predictive Accuracy: Models can forecast future sales volumes based on price changes and other external factors, allowing for data-backed decisions.
- Dynamic Adjustments: With continuous learning, prices can be adjusted automatically as new data comes in, ensuring competitiveness without manual intervention.
- Customer Segmentation: By analyzing past purchasing behavior, businesses can tailor prices to different consumer segments, fostering increased sales through personalized offers.
Nevertheless, supervised learning isn't without its hurdles. The quality of the outcome is heavily reliant on the quality of the data fed into the models. If the historical data is of poor quality or biased, the predictions can lead a business astray.
Unsupervised Learning Applications
Now, shifting our focus to unsupervised learning, this approach differs primarily in that it works with data that is not labeled. This method is particularly useful when businesses seek to uncover hidden patterns or groupings in their pricing strategy without a clear directive.
Examples of unsupervised learning applications in pricing include:
- Clustering Techniques: Businesses can identify distinct groups of customers through clustering, determining how diverse pricing strategies might benefit different segments.
- Market Basket Analysis: By analyzing purchase patterns, companies can recognize which products are commonly bought together. This knowledge can inform Bundling strategies or promotional pricing.
- Anomaly Detection: Unsupervised algorithms can flag unusual pricing trends or consumer behavior that deviate from the norm, allowing businesses to investigate potential issues quickly.
Despite the advantages, companies must be cautious of the potential pitfalls. Since there are no labeled outcomes, interpreting the results of unsupervised learning often requires careful analysis to ensure the insights gained are actionable and relevant.
"The future of pricing is no longer about setting prices based on gut feelings but leveraging deep insights from data."
Data Sources for AI-Driven Pricing
To harness the full potential of AI in pricing, the sources of data become pivotal. Data sources for AI-driven pricing strategies can distinctly shape decisions and outcomes. Pricing is not merely a number on a tag; it’s a dynamic component shaped by various inputs. By analyzing the right data, businesses can set prices that not only attract customers but also maximize profit margins. The conversation around data sources focuses on two core areas: internal data utilization and external data integration.
Internal Data Utilization
When discussing internal data utilization, it refers to the pool of information that organizations generate during regular operations. This can include sales data, customer interactions, inventory levels, and even historical pricing information.
Access to this data is akin to having a treasure trove — it reveals patterns that can inform future pricing decisions. For instance, tracking seasonal sales data can help businesses forecast demand for specific products during peak times. If a online retailer notices fluctuations in sales correlating with promotional events, they might consider adjusting their pricing strategies during future campaigns. Here are some specific points worth noting about internal data:
- Sales Performance: Analyzing which products sell well and which do not provides insight into consumer preferences.
- Customer Purchase Behavior: Understanding how often and why customers buy can lead to effective pricing strategies that cater to purchasing habits.
- Inventory Management: Keeping track of stock levels helps in deciding when to reduce prices or run sales to clear out old inventory.
By leveraging internal data effectively, companies can create a robust, responsive pricing strategy that reflects their unique selling environment.
External Data Integration
On the flip side, external data integration pulls information from outside an organization. This data comes from various sources, like market trends, competitor pricing, and consumer sentiment executed through social media channels or forums. Relying solely on internal data might leave a business blind to market shifts.
"What’s going on outside the window can change what’s happening inside the room."
Enhancing pricing strategies with external data ensures responsiveness and agility. Consider the elements of external data integration:
- Competitor Pricing: Regularly analyzing competitor prices helps in positioning your offerings competitively.
- Market Trends: Keeping an eye on economic indicators and seasonal trends ensures prices align with market demand.
- Consumer Feedback: Social media platforms and review sites provide insight into consumer perception, which is vital for adjusting pricing based on public sentiment.
Understanding how to weave together both internal and external data creates a richer tapestry for pricing strategy. Companies who effectively employ these sources navigate the competitive landscape with finesse, ensuring their prices meet both consumer expectations and business goals. Thus, the integration and analysis of relevant data sources are not just beneficial but paramount to succeed in today’s fast-paced market.
Consumer Behavior Insights Through AI
Understanding consumer behavior through artificial intelligence (AI) has become a cornerstone of modern pricing strategies. In an era where data is the new oil, leveraging AI allows businesses to tap into deep-rooted consumer preferences and tendencies with unparalleled precision. As markets grow increasingly competitive and consumer expectations evolve, insights gained from AI can guide companies in crafting more effective pricing strategies.
Understanding Price Sensitivity
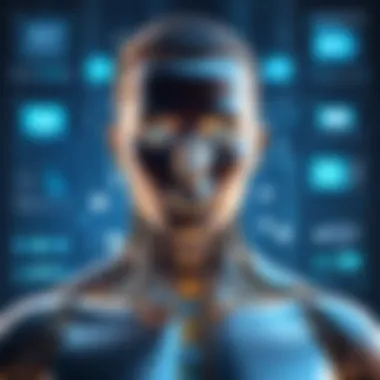
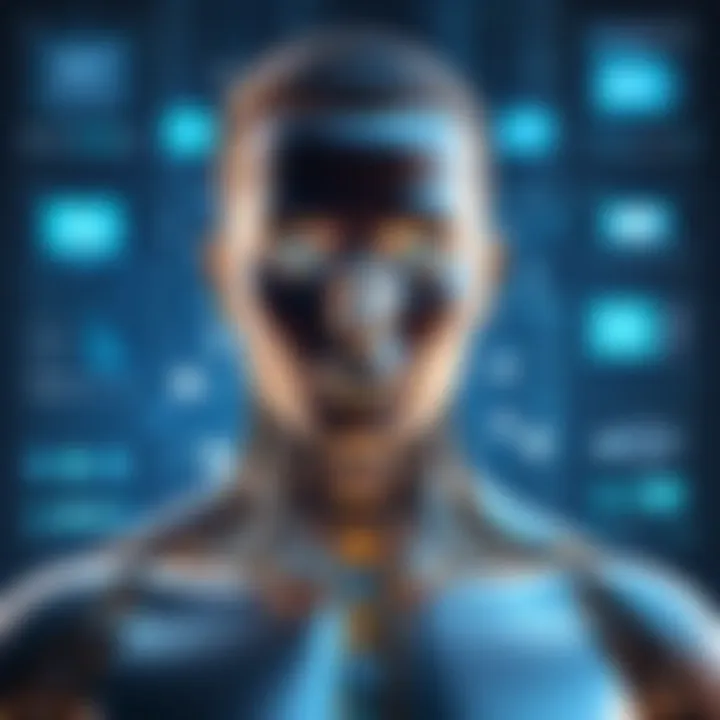
Price sensitivity refers to how the demand for a product or service changes as its price varies. It’s a critical element for businesses to consider, as it directly influences sales and profitability. AI significantly enhances the ability to gauge price sensitivity by processing vast amounts of data quickly and accurately.
- Predictive Analysis: AI tools can analyze historical sales data, considering factors like seasonality, market trends, and economic indicators. By recognizing patterns, they can predict how a potential price change might affect sales volumes.
- Segmented Insights: Different consumer groups often respond differently to price changes. An AI system can identify these segments and tailor pricing strategies accordingly, offering personalized pricing that resonates with specific demographics.
- Real-time Feedback: AI allows companies to monitor consumer reactions to price changes in real-time, enabling them to adjust strategies quickly. This responsiveness helps businesses stay ahead of the curve and maintain competitive pricing.
This continuous feedback loop ensures that businesses remain aligned with consumer expectations and economic conditions.
Behavioral Analysis
Behavioral analysis, powered by AI, goes beyond just understanding what consumers buy; it delves into why they make certain purchasing decisions. This introspective view of consumer actions helps businesses refine their marketing and pricing strategies.
- Purchase Triggers: Understanding what prompts consumers to buy at particular prices is invaluable. AI can uncover hidden drivers, such as marketing campaigns or social media trends, and how they correlate with purchasing behavior.
- Loyalty Factors: AI models can examine factors contributing to brand loyalty, such as consistent pricing, perceived value, and customer service. Identifying these elements helps businesses enhance customer retention strategies.
- Sentiment Analysis: By analyzing social media interactions and customer reviews, AI can gauge public sentiment towards a product or pricing strategy, allowing businesses to pivot when necessary to maintain consumer trust and engagement.
"Behavioural insights gained from AI aren’t just numbers; they reflect the complex tapestry of consumer desires and motivations, paving the way for more meaningful connections between businesses and their customers."
Challenges in AI Pricing Implementation
The integration of AI in pricing strategies is no walk in the park. While automation and advanced algorithms can boost efficiency and accuracy, organizations face significant hurdles that can impede their success. Understanding these challenges is crucial for businesses aiming to navigate the complexities of AI pricing.
Data Privacy Concerns
Data privacy stands out as one of the foremost challenges in AI pricing implementation. As companies gather vast amounts of consumer data to refine pricing algorithms, they tread a fine line between gathering valuable insights and respecting user privacy.
In recent years, there have been numerous regulations, like the GDPR in Europe and CCPA in California, which safeguard consumer data privacy. These laws impose strict guidelines on how organizations collect, store, and utilize personal information. Failing to comply with these laws not only risks hefty fines but also can damage an organization's reputation.
Furthermore, the general public is becoming increasingly aware of data privacy issues. Trust is a currency that cannot be bought and, in the age of social media, any misstep can result in a PR nightmare. Businesses must prioritize transparency in their data collection methods, ensuring they are upfront with customers about how their data will be used. To build and maintain trust, many companies are adopting privacy-first approaches and using anonymized data sets to train their algorithms.
- In summary:
- Prioritizing data privacy helps maintain consumer trust.
- Compliance with regulations is essential to avoid penalties.
- Transparency in data handling fosters a positive brand image.
Algorithm Transparency
Algorithm transparency is another sticking point when it comes to AI implementation in pricing strategies. While AI has the potential to enhance pricing models significantly, the opacity surrounding algorithmic decision-making can lead to skepticism among stakeholders, including consumers and regulators.
When algorithms operate without clear explanations, it raises various ethical concerns. For instance, if a consumer feels that prices fluctuate unfairly owing to hidden algorithms, they may view the brand as manipulative or untrustworthy. This perception can profoundly affect customer loyalty.
Additionally, many organizations struggle with elucidating how their models work. For software developers and data science professionals, it’s pivotal to ensure that pricing algorithms are interpretable. This means making an effort to clarify how different variables impact pricing decisions. Providing visualizations and reports that break down these processes can be instrumental in building trust.
"In a world of algorithms, a little transparency goes a long way in retaining customer loyalty."
- Key Considerations:
- Organizations need to actively work on demystifying their algorithms.
- Clear communication strategies can mitigate skepticism.
- Investing in tools that visualize algorithm relationships will enhance interpretability.
By addressing data privacy and algorithm transparency, companies can not only overcome significant challenges in AI pricing implementation but also carve a path toward a more trustworthy and efficient pricing strategy.
Ethical Considerations in AI Pricing
In today’s hyper-competitive marketplace, the integration of artificial intelligence into pricing strategies is proving to be a game-changer. However, amid the promising potential lies a web of ethical considerations that cannot be overlooked. These elements are crucial not just for maintaining a company's reputation but also for fostering a sustainable business environment. A deeper understanding of ethics in AI pricing positions stakeholders to act responsibly while leveraging technology.
When discussing ethical considerations, fairness in pricing models takes center stage. It is vital to ensure that algorithms do not inadvertently discriminate against any demographic, as doing so can lead to severe repercussions. Tailored pricing strategies based on data analysis must strive to avoid biases, enabling equal opportunities for all consumers. The challenge is crafting models that balance profitability with equitable treatment.
Fairness in Pricing Models
The question of fairness revolves around the algorithms that dictate pricing strategies. The lack of transparency is a prominent concern. When prices vary significantly based on consumers' online behavior or socioeconomic status, it creates an imbalance and a perception of exploitation. For instance, if a homeowner in a wealthy neighborhood consistently receives higher quoted prices for similar services compared to an individual in a less affluent area, it raises eyebrows. This kind of pricing discrimination can alienate customers and diminish trust in the brand.
Moreover, one can argue about the ethical implications of price optimization based on individual willingness to pay. A dynamic pricing model may adjust prices based on what the software anticipates a customer is willing to spend, which raises ethical challenges. Although such practices might boost profits, they could lead to feelings of inequity among consumers.
Key takeaways about fairness in pricing models include:
- Transparency: Providing clear communication about how prices are determined can build trust.
- Inclusivity: Ensuring pricing models consider all demographics helps mitigate bias.
- Responsibility: Companies should take accountability for how their algorithms perceive and treat various consumer groups.
Consumer Trust Issues
Trust is the bedrock of any customer-business relationship. With AI’s evolving role in pricing strategies, maintaining consumer trust becomes more challenging yet necessary. If customers think they are being manipulated through sophisticated algorithms, they naturally become suspicious. Trust is particularly fragile when pricing fluctuates based on perceived data. Consumers may feel like they are in a game, always guessing the correct time to buy, which can lead to frustration.
Furthermore, brands must navigate the thin line between personalization and intrusion. While tailored prices might seem beneficial, they can also feel invasive. For example, a customer may appreciate personalized discounts but may bounce if they sense being constantly watched or analyzed. This is where ethical considerations play a crucial role in creating a balance between targeted engagement and privacy.
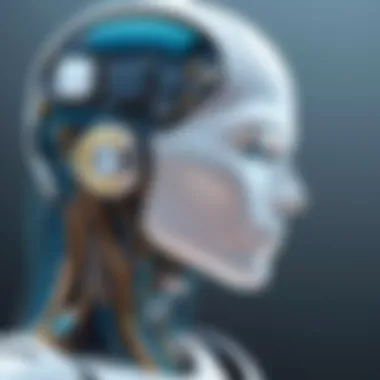
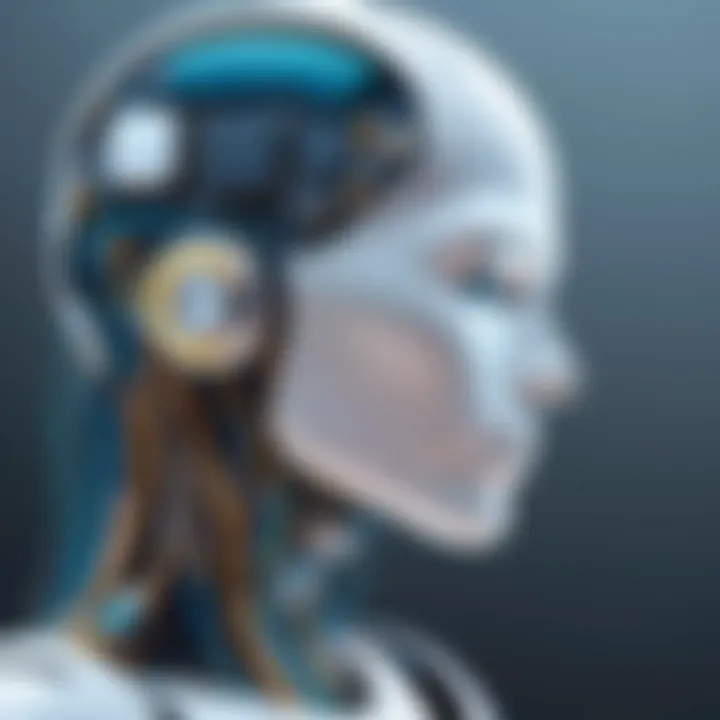
To foster consumer trust, firms may consider the following steps:
- Transparency in Data Usage: Clearly explain how customer data influences pricing decisions.
- Guaranteeing Fair Practices: Brands must commit to employing ethical AI practices that uphold fairness.
- Encouraging Two-Way Communication: Engaging consumers in dialogue around pricing algorithms can help foster a sense of community and trust.
In summary, as AI continues to shape pricing strategies, the ethical considerations surrounding fairness in pricing models and consumer trust issues must lead the conversation. Brands shifting toward AI-driven pricing must take the time to implement responsible practices, or risk not only losing consumer trust but also potentially facing legal scrutiny. Companies that prioritize ethics will likely outperform those that view pricing as merely a numbers game.
Case Studies of AI in Pricing
The examination of real-world applications of artificial intelligence in pricing strategies offers profound insights into its transformative effects. Case studies allow us to analyze how diverse sectors are leveraging AI to fine-tune their pricing models, thereby enhancing competitiveness and operational efficiency. By looking at specific examples, we can understand the practical benefits and challenges these organizations encounter. This section isn't just about successes, but also about the iterative nature of technology adoption, where companies learn and evolve through trial and error.
E-commerce Platforms
E-commerce platforms stand at the forefront of AI-driven pricing. Companies like Amazon have carved out a niche by employing sophisticated algorithms that adjust prices in real-time based on various factors such as demand fluctuations, competitor pricing, and inventory levels. This is not merely a gimmick; it serves a vital role in ensuring that products remain competitively priced while maximizing profit margins.
For instance, a study of Amazon’s pricing strategy revealed how their dynamic pricing model can lead to significant revenue increases. By analyzing consumer behavior and competitor actions, Amazon's algorithms make adjustments every few minutes, often resulting in prices that could change as frequently as 2.5 million times in one day. By doing this, they strike a delicate balance between attracting new customers and retaining existing ones.
Moreover, platforms like eBay utilize AI analytics to access insights about buyer trends and preferences. They often use machine learning models to assess how certain categories perform over time. This information aids sellers in strategically pricing their items to reflect current market conditions while also considering seasonality trends.
"In today’s fast-paced digital marketplace, a minor tweak in pricing can make or break an online seller. E-commerce platforms that harness AI not only save time but also gain a competitive edge."
Retail Examples
In the brick-and-mortar retail world, companies such as Walmart and Target are also deploying AI for pricing strategies. They harness data from various sources, including customer shopping habits and stock levels, to adjust prices dynamically. A notable example can be seen in how Target experiments with price changes during holidays or campaigns, analyzing how slight alterations can influence foot traffic and purchase volume.
Walmart’s utilization of AI extends into their pricing practices as well. By implementing predictive analytics, they can forecast demand for products based on historical trends, social media sentiment, and local events. This level of sophistication helps them set prices that are not only competitive but are also tailored to the unique characteristics of each store's target audience and market conditions.
Additionally, Sephora has made strides with its AI applications by pricing its products based on personalization tactics. Using customer profile data, they customize pricing messages and promotional offers to appeal to specific demographics. Through these methods, they engage customers more effectively while fostering loyal relationships by acknowledging individual preferences.
By learning from these case studies of e-commerce and retail, businesses can draw lessons on the profound impact AI has on pricing strategies. It is clear that as technology progresses, integrating AI into pricing is becoming not just beneficial but essential for survival in a competitive market.
Future Trends in AI for Pricing
As we venture into the future, it becomes increasingly clear that AI will continue to reshape the pricing landscape in profound ways. The incorporation of AI technology into pricing strategies not only provides efficiency but also enhances decision-making capabilities. This section will delve into the advancements that will mark the next phase of AI in pricing and the growing trend towards personalized pricing strategies that can cater specifically to consumer preferences.
Advancements in AI Technology
The rapid evolution of AI technologies is paving the way for more intricate and effective pricing models. One of the most significant advancements is in the area of predictive analytics. By leveraging vast amounts of data, AI algorithms can more accurately forecast market trends and customer behavior, allowing businesses to set prices that maximize profitability while remaining competitive.
- Machine Learning: This has become a cornerstone of pricing strategy, moving beyond traditional pricing methods. Machine learning approaches can continuously learn from new data, adapt to changing market conditions, and fine-tune pricing strategies on the fly.
- Natural Language Processing (NLP): Companies can now analyze social media sentiment to gauge public perception of their products, allowing them to adjust prices accordingly. For example, if sentiment toward a product declines, businesses can respond by adjusting prices to incentivize sales.
"The future transformation of pricing will depend on how effectively businesses leverage advancements in AI technology to meet the expectations of an evolving market."
Furthermore, technologies like Reinforcement Learning enable algorithms to experiment with different pricing strategies and learn from the outcomes. This adaptive capability can significantly enhance the effectiveness of pricing approaches. In short, as AI tools grow more sophisticated, so do the strategies companies can employ to remain relevant.
Increasing Personalization in Pricing
Personalization in pricing is not just a passing trend; it's becoming an expectation among consumers. People are more informed than ever before, and they seek offers that align closely with their individual needs. AI plays a crucial role in making this a reality.
- Dynamic Pricing: By analyzing user data, such as buying habits or online behavior, companies can create personalized price points that cater to individual customers. For instance, an online retailer might offer tailored discounts to loyal customers based on their shopping history.
- Behavioral Targeting: AI can help businesses understand and predict customer behaviors, allowing them to craft pricing strategies that feel more like bespoke offers than generic sales. This can effectively increase customer loyalty and boost conversion rates.
Moreover, the development of AI tools enables businesses to create real-time segmentation of their customer base, tailoring not only prices but also marketing strategies to various segments. High-value customers might receive exclusive pricing while more price-sensitive shoppers could see different incentives, thus ensuring that everyone feels valued while also optimizing revenue.
In essence, as AI technologies advance, the transition toward a hyper-personalized pricing model is not just probable; it’s inevitable. Understanding these trends will be key for businesses as they navigate this intricate landscape of AI-driven pricing strategies.
Culmination
As we stitch together the intricate tapestry of AI in pricing strategies, it’s clear that this topic is not just a fleeting trend but a significant evolution in the business landscape. The dynamic of pricing is at the heart of revenue generation, and understanding how AI influences this can’t be overstated.
Summary of Key Insights
Throughout the article, we've highlighted several critical insights regarding the integration of artificial intelligence into pricing strategies:
- Transformative Impact: AI is fundamentally reshaping how businesses approach pricing through data-driven insights and dynamic models, allowing for real-time adjustments based on market conditions.
- Consumer Behavior Understanding: With the help of AI analytics, companies can decipher complex consumer behaviors and sensitivities, tailoring prices to maximize their appeal.
- Challenges and Considerations: Despite its advantages, implementing AI in pricing is not without its challenges, including data privacy issues and the need for transparency in algorithms.
- Ethics and Trust: Establishing fairness and maintaining consumer trust are crucial to leveraging AI effectively in pricing strategies. The balance between profit and ethical considerations sets the tone for sustainable practices.
- Future Trends: Looking ahead, advancements in technology and increasing personalization in pricing will further embed AI in pricing strategies, making adaptability a key factor for success in competitive markets.
In a nutshell, this comprehensive exploration underscores AI's role as a catalyst for innovative pricing strategies, providing a scaffold for businesses to build upon.
Call to Action for Businesses
It's not just about keeping up; it's about getting ahead. Businesses should:
- Invest in AI Tools: Implement advanced AI tools and technologies to leverage data effectively for informed pricing decisions.
- Understand Consumer Insights: Use AI analytics to gain deeper insights into consumer behavior and adapt strategies accordingly.
- Foster Ethical Practices: Ensure that pricing models are not only profitable but also fair and transparent, thereby fostering trust with consumers.
- Stay Agile: The marketplace is ever-changing; businesses must remain agile and ready to adapt their pricing strategies in real-time.
In a rapidly evolving digital world, harnessing the power of AI for pricing is more than just an option—it's a necessity for survival and growth. The opportunity is ripe; now is the time to act.