Understanding Azure Stream Analytics Pricing Structure
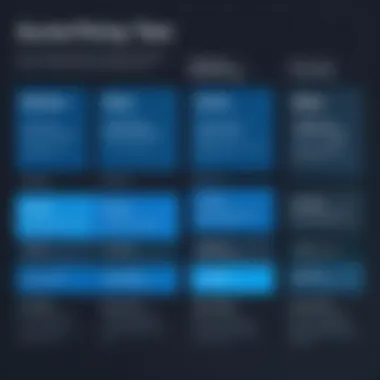
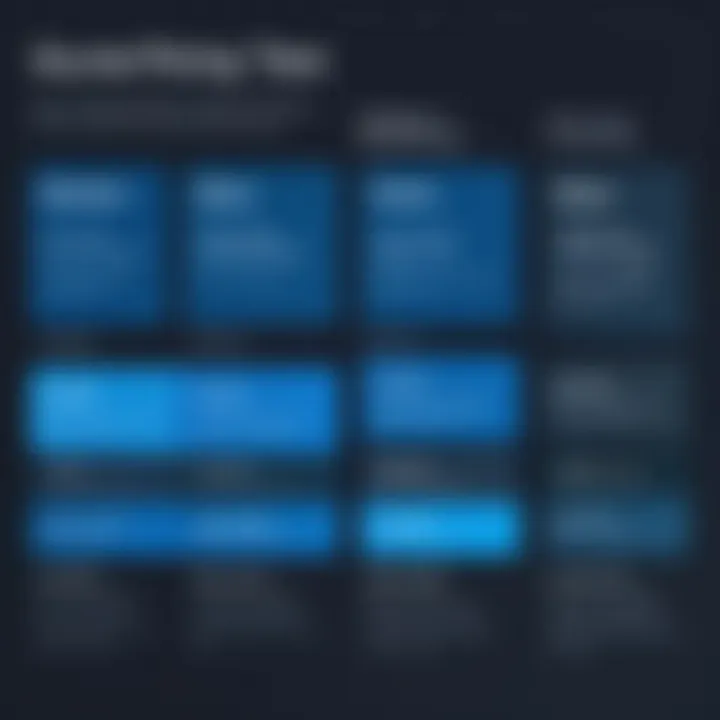
Intro
In today's data-driven world, businesses hinge on the ability to analyze and act on information in real-time. Azure Stream Analytics is a powerful service that supports this need, yet understanding its pricing structure often proves to be a perplexing endeavor. Knowing how costs are determined not only aids in budgeting but can also optimize application deployment while ensuring the best bang for the buck.
For tech professionals and software users alike, deciphering Azure's pricing can feel like uncovering the secrets of a treasure map. It’s crucial to sift through the available data, taking into account variables that influence costs and understanding billing models that might come into play. From a one-off project to continuous streaming analytics, each scenario demands its own careful consideration of expenses.
As we navigate this article, we’ll dissect how the Azure Stream Analytics pricing model functions, spotlight key features of the software, and provide insights on cost management strategies. Let’s get down to brass tacks and delve deeper into this subject.
Software Overview
Before we discuss pricing, it’s beneficial to spotlight what Azure Stream Analytics offers and its core features. Understanding these functionalities can provide a foundation for grasping potential costs.
Key Features
Azure Stream Analytics is a cloud-based service that enables real-time data stream processing and analysis. Some standout features include:
- Real-time analytics: Process streams of data to identify patterns and trends instantly.
- Integration capabilities: Seamlessly interacts with Azure and other third-party services for a flexible, cohesive environment.
- Scalability: Effortlessly adjust resource allocation based on workload demands.
- Built-in machine learning: Supports predictive analytics, elevating the depth of insights.
These features collectively position Azure Stream Analytics as a proactive tool tech professionals can leverage to stay ahead.
System Requirements
For those looking to implement Azure Stream Analytics, ensuring proper prerequisites is vital. Key requirements include:
- Azure subscription: This is fundamental for accessing Azure services.
- Access to Azure Portal: Provides a visual interface for setting up and managing streaming projects.
Understanding these essentials allows users to avoid potential roadblocks that can hinder data processing.
In-Depth Analysis
Having a firm grasp on what the software entails sets the stage for a deeper exploration into the crux of pricing.
Performance and Usability
Keeping performance in check is paramount. Azure Stream Analytics offers users a straightforward interface, making it a breeze for professionals with various technical backgrounds to harness its power. The processing capabilities are noteworthy—most businesses report impressive speeds in stream handling, resulting in timely insights necessary for decision-making.
Best Use Cases
Azure Stream Analytics is well-suited for a variety of applications:
- IoT applications: Ensuring real-time data processing from devices out in the field.
- Financial transactions: Analyzing patterns for fraud detection instantly.
- Social media monitoring: Gleaning insights from user interactions to drive marketing strategies.
Each use case highlights the myriad of ways Azure Stream Analytics can be utilized, but they also emphasize the importance of understanding the pricing structures as they vary significantly across applications.
"Effective decision-making hinges on timely and accurate insights; hence, knowing your costs beforehand is key."
By scrutinizing the varying factors influencing pricing and taking into account practical usage scenarios, this article aims to furnish readers with a holistic understanding necessary for making informed choices in the realm of Azure Stream Analytics.
Preamble to Azure Stream Analytics Pricing
Understanding the pricing structure of Azure Stream Analytics is crucial for those who engage with the platform, whether it be software developers, IT professionals, or even students in related fields. This section serves as the gateway to comprehending not only how costs are determined but also how to navigate these costs effectively. With a myriad of services and features bundled into Azure, having clarity on the financial aspect helps users make informed decisions, maximizing both efficiency and budget.
In the vast world of data processing, choosing the right tools can be akin to picking the right tool in a shipyard. One wrong tool, and the whole project may flounder. The same applies here: Azure Stream Analytics offers powerful capabilities but navigating its pricing landscape requires a keen understanding of how various factors play into the final figure on the bill. Thus, this article endeavors to dissect the different pricing models, identify the factors that affect costs, and lay out strategies for managing expenses.
Overview of Azure Stream Analytics
Azure Stream Analytics is a real-time data stream processing service offered by Microsoft Azure. It allows users to analyze data streams from various sources, such as IoT devices, social media feeds, or application logs, essentially transforming data into actionable insights on the fly.
This service is pivotal in environments where timely data analysis can result in competitive advantages, such as in more effective decision making, enhanced customer experiences, or predictive maintenance in manufacturing. Azure Stream Analytics enables complex event processing and allows users to write SQL-like queries to filter, aggregate, and analyze data. But, as with any powerful tool, understanding how its pricing works can significantly influence how users design their data architectures and operational workflows.
Importance of Understanding Pricing
The importance of understanding pricing for Azure Stream Analytics cannot be overstated. Without grasping the intricacies, businesses may find themselves in a financial pickle, overspending on resources, or underutilizing capabilities. Knowing what influences costs helps businesses tailor their use of Azure to align with their budgetary constraints and operational objectives.
Here are key elements that underscore the importance of this knowledge:
- Cost Control: Awareness of pricing allows for better budget management. When a user knows how charges accumulate, they can make real-time adjustments to keep expenses in check.
- Resource Optimization: Stream Analytics offers various features. Understanding what adds to the bill aids in making intelligent choices about which features to implement or scale.
- Scalability: As data volumes grow, costs can spiral unless users are prepared. Familiarity with how pricing scales ensures that growth does not come as an unwelcome surprise.
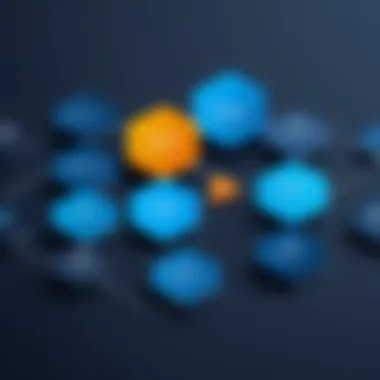
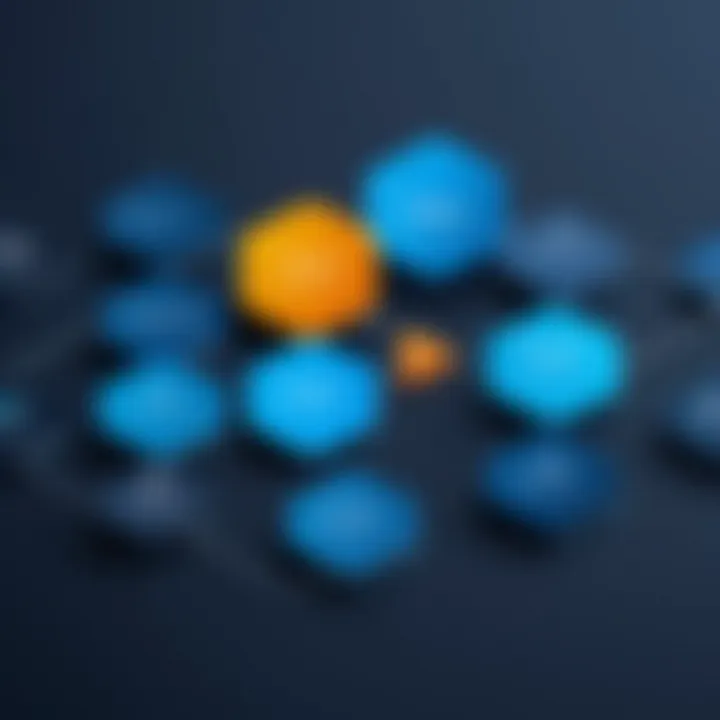
Understanding Azure Stream Analytics Pricing is not just about costs; it's about empowerment in decision-making.
In summary, comprehending the pricing models and their implications is vital for anyone who wishes to leverage Azure Stream Analytics effectively. This knowledge lays the groundwork for strategic planning, enabling users to not only forecast their costs but also align their technical capabilities with their business goals.
Pricing Models Explained
Understanding the pricing models for Azure Stream Analytics is crucial for developers, IT professionals, and students alike. The costs incurred can significantly impact project budgets and resource allocation decisions. There are generally two main types of pricing models used in Azure Stream Analytics: consumption-based pricing and fixed pricing options. Each model offers its own set of benefits and considerations.
Consumption-Based Pricing
This is probably the most attractive option for many users. In a nutshell, it’s pay-as-you-go. With consumption-based pricing, you only pay for what you use. This can be particularly beneficial for projects with fluctuating workloads. For instance, if a project experiences varying data ingestion rates during peak times of the year, companies can save a pretty penny by avoiding fixed costs associated with underutilized resources.
- Charging Mechanism: Charges accumulate based on the actual resources consumed—compute and input/output data events. This structure can lead to very dynamic cost management, depending only on real usage.
- Scalability: With consumption-based pricing, scaling becomes flexible. Developers can ramp up resources when needed without the worry of sinking money into unused capacity.
However, there's a flip side. If the usage unexpectedly spikes, costs can balloon quickly. Make sure to monitor your usage trends regularly. Setting up alerts within Azure can help manage the expenses.
Fixed Pricing Options
Fixed pricing provides a little more predictability to budgeting. Essentially, this model offers a predetermined amount of resources, billed at a set rate. This can be advantageous for organizations that prefer to keep costs stable and avoid unexpected charges.
- Budgeting Certainty: You can plan your expenses more precisely. This is particularly useful for larger enterprises or long-term projects, where knowing the budget means less sleepless nights worrying about overspending.
- Resource Commitment: Fixed pricing may require more upfront planning. Once a level of resources is committed, it’s crucial to ensure that they are properly utilized to avoid waste.
It’s worth noting that fixed pricing may not be the best solution for everyone. Companies with variable needs may find this structure less appealing. The balance between predictability and flexibility is a key consideration when deciding which model works best for your specific needs.
As businesses evolve, understanding these pricing models becomes fundamental. When considering Azure Stream Analytics, it’s important to evaluate both approaches and align them with your project goals and financial strategies.
"All that glitters is not gold; not all models will suit every project."
Factors Influencing Azure Stream Analytics Costs
Understanding the factors that contribute to Azure Stream Analytics costs is crucial for organizations aiming to make the most out of their cloud resources. Several elements influence pricing, and recognizing them can lead to better budget management and optimal utilization of services. This section breaks down three key factors that significantly impact how much users ultimately pay: data ingestion rates, output data management, and job complexity.
Data Ingestion Rates
Data ingestion rates refer to how much data is brought into the Azure Stream Analytics service for processing. This is not merely a technical metric; it has direct implications for cost. The more data you process, the higher your bills will climb. Companies often underestimate the volume of data generated through various sources, leading to unexpected expenses.
For instance, if an organization is streaming real-time data from IoT devices, they might think their data needs are modest. However, as more devices connect and more data flows in, the ingestion rate can surge, resulting in high costs. Keeping tabs on ingestion rates is essential—monitoring traffic can help fine-tune what data is necessary and what can be culled.
Key considerations for optimizing ingestion rates include:
- Prioritizing necessary data: Avoid processing data that isn’t crucial for decision-making.
- Implementing sampling: Instead of capturing every data point, consider collecting only a representative sample at intervals.
- Using compression: Where applicable, compress data before ingestion to reduce volume.
Output Data Management
Once data is ingested, how it’s managed has cost implications too. Output data management refers to the control of the processed data you send out of Azure Stream Analytics to other services, often requiring another layer of costs due to storage or transmission.
This can be a tricky area; unintended outputs can pile on costs quickly. For example, if you process data that is later dumped into various storage solutions, each transfer can incur charges. A strategic approach would be to define clear pathways for outputs:
- Target only necessary outputs: Ensure you’re only sending the data you really need, and that unnecessary data isn’t clogging your pipelines.
- Aggregate outputs when possible: Instead of sending raw data, consider aggregating it to lessen the frequency of data transfer.
- Evaluate storage solutions: Using services with lower costs for storing your output data can save you a pretty penny in the long run.
Job Complexity
Finally, the intricacy of the jobs you run in Azure Stream Analytics plays a significant role in cost determination. Simpler jobs that involve straightforward data transformations will generally be cheaper than complex jobs that require numerous operations and conditions. The more elaborate the query logic and the more resources needed to execute it, the larger the cost hit.
If your jobs include extensive calculations or advanced analytics, it’s time to take a close look at your setup. A few strategies can help mitigate cost:
- Simplifying queries where feasible: Review job definitions and consider whether all aspects are necessary.
- Breaking complex jobs into smaller tasks: Smaller, targeted jobs can often run more efficiently, while also improving manageability.
- Scaling resources accordingly: Sometimes investing in more substantial resources for computation can be more economical in the long run than letting inefficient jobs run for extended periods.
In summary, awareness of data ingestion rates, output data management, and job complexity is vital for anyone looking to use Azure Stream Analytics effectively and affordably. Recognizing these factors not only aids in budgeting but also enhances overall operational efficiency.
Detailed Pricing Breakdown
Understanding the intricate pricing breakdown of Azure Stream Analytics is crucial for any organization looking to derive value from their data streams. This section explores the various costs involved, helping you discern the financial implications of your data operations. Knowing the ins and outs of these prices allows businesses to set realistic budgets and forecast their expenses accurately. It’s all about aiming for a balance between cost and efficiency.
Compute Costs
In Azure Stream Analytics, compute costs are typically one of the most significant expenses. These costs stem from the processing power utilized during data analysis tasks.
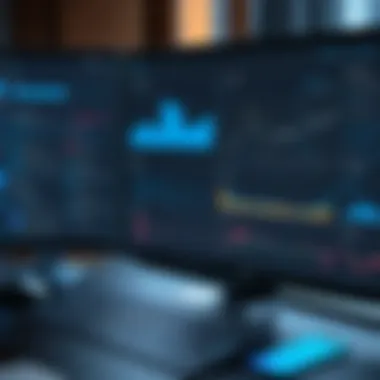
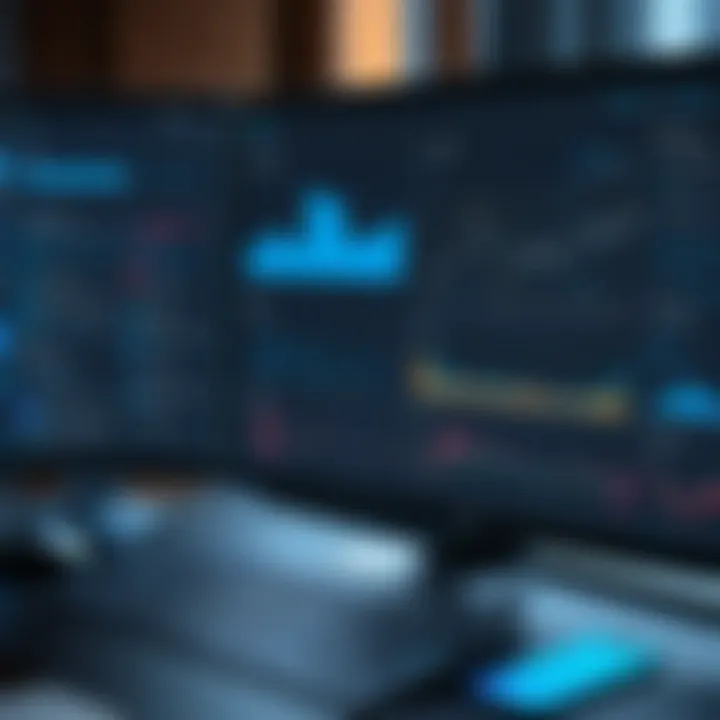
When evaluating compute costs, it’s vital to note factors like:
- Job Type: Different jobs require varying amounts of resources. For example, a simple query will naturally consume less computing power than a complex, multi-input job.
- Execution Time: The longer your job runs, the more you’ll pay. It’s similar to running a marathon versus a sprint; the longer you go, the more energy you expend.
- Select Number of Streaming Units: You can choose how many streaming units to allocate depending on your needs. More units equal higher costs but also better performance.
"Understanding compute costs lets you tailor your usage to avoid unnecessary waste, similar to how one would manage energy in daily life."
By tactically managing these aspects, you can optimize your compute expenses while still meeting your analytic needs.
Storage Costs
Storage costs come into play when dealing with the data you ingest and retain. Azure charges for both the amount of data stored and the duration of that storage. Key considerations in storage pricing include:
- Data Retention Policies: An arbitrary retention policy can lead to inflated costs. It’s smart to evaluate how long you actually need to keep the data, because retaining unnecessary data can strain your budget.
- Data Redundancy Options: Azure offers various levels of redundancy that also influence the overall storage costs. Choosing the right balance means assessing your actual needs versus the risks of potential data loss.
Increasing storage efficiency can not only trim costs but also bolster your data management strategies regional spans.
Data Transfer Charges
Finally, let’s not overlook data transfer charges, often underestimated in budget computations. These charges accrue when data is moved in and out of Azure Stream Analytics:
- Inbound vs. Outbound Data: Data entering Azure is typically free, but outbound data transfers can rack up costs, particularly if dealing with large datasets.
- Inter-regional Transfers: Transferring data between different Azure regions comes with additional fees.
It’s useful to keep an eye on where your data is moving. Knowing its path can help you strategize and minimize costs.
When combined, an awareness of compute, storage, and data transfer costs provides a comprehensive understanding of how to effectively manage your Azure Stream Analytics expenses.
Cost Management Strategies
When it comes to Azure Stream Analytics, managing costs can feel like navigating through a maze without a map. Proper cost management strategies are vital for anyone looking to keep their expenses in check while maximizing the value of this powerful tool. You see, the beauty of Azure Stream Analytics lies in its flexibility, but this very flexibility might lead to unforeseen expenses if not handled wisely. It’s not just about working with a technology; it’s about ensuring that the benefits outweigh the costs.
The focus here is to explore key elements of cost management which not only maintains fiscal health but also enhances the effectiveness of your projects in the long run.
Budgeting for Azure Stream Analytics
Budgeting is the backbone of any successful operation. Without a clear budget, you might find yourself tossing funds at the wall, hoping something sticks. A well-defined budget for Azure Stream Analytics involves understanding the various pricing models in conjunction with anticipated usage rates. This proactive approach lays the groundwork for developing a reliable financial plan.
Start by breaking down your expected workloads. Identify peak usage periods and align them with your budgeting specifics. This way, if you know certain times of the year will require more data processing, you can allocate more resources accordingly. Here are some tips for effective budgeting:
- Estimate usage based on historical data: Look for patterns that can help you make informed predictions.
- Account for data spikes: Sudden increases in data volume or processing may demand urgent adjustments.
- Consider testing environments: It's wise to factor in costs for developmental work or testing as well, as these can accumulate quickly.
By approaching budgeting strategically, you can create a safety net that ensures you’re never caught off guard when bills arrive at the end of the month.
Optimizing Resource Usage
Optimization is an art form unto itself. In the context of Azure Stream Analytics, optimizing resource usage means striking a balance where you leverage the full capability of the service without overspending. Think of it like tuning a musical instrument – it’s all about finding that sweet spot where everything resonates perfectly.
Start by identifying which resources you’re utilizing most. Are you maxing out on compute costs? Or perhaps your data transfer charges are creeping higher than expected? Here are some strategies to ensure efficient resource usage:
- Use auto-scaling features: Azure provides options that automatically adjust resources based on current processing needs. This can prevent overprovisioning.
- Thorough monitoring: Keep an eye on all aspects of job performance - thanks to Azure’s built-in monitoring tools. This insight can alert you to unnecessary resource consumption.
- Job design and Queries: Simplify queries when possible. Complicated queries can take additional processing time, leading to increased costs.
By staying vigilant and adjusting as necessary, the aim is to trim the fat from your resources while sharpening your operational edge.
"Managing costs effectively can empower users to focus on innovation rather than financial strain."
Comparative Analysis with Alternative Solutions
In today’s data-driven world, making informed decisions about which streaming analytics solution to adopt is more pivotal than ever. A comparative analysis with alternative solutions provides insights that can guide organizations in selecting the most suitable platform for their specific needs. Not only does this approach highlight the strengths and weaknesses of Azure Stream Analytics, but it also enables a side-by-side understanding of pricing structures, capabilities, and overall value propositions among competing products.
Advantages of Azure Stream Analytics
Azure Stream Analytics shines in several areas that makes it a top choice. Here are a few noteworthy advantages:
- Integration with Other Azure Services: One of its standout features is seamless integration with Azure services like Azure Event Hubs and Azure Functions. Businesses using a suite of Azure products will find it easy to incorporate Stream Analytics without extra friction.
- Real-Time Processing: It excels in real-time processing. If your application relies on instant insights from data streams, Azure Stream Analytics serves as a reliable option. The ability to process millions of events per second allows for immediate data analysis, which is crucial for many businesses in sectors like finance and telecommunications.
- User-Friendly Interface: The user-friendly interface and simple query language reduce the learning curve. New users can easily craft queries without extensive technical knowledge, promoting broader adoption within teams.
- Cost Efficiency: When optimized, the consumption-based pricing can lead to significant savings, especially for businesses with fluctuating workloads.
- Scalability: Azure understands the necessity for businesses to scale with growth. Stream Analytics can manage both small and vast data streams without compromising on performance.
Limitations in Pricing
Despite its many strengths, it’s essential to be wary of certain limitations associated with Azure Stream Analytics pricing:
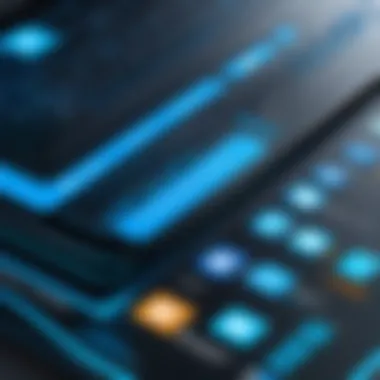
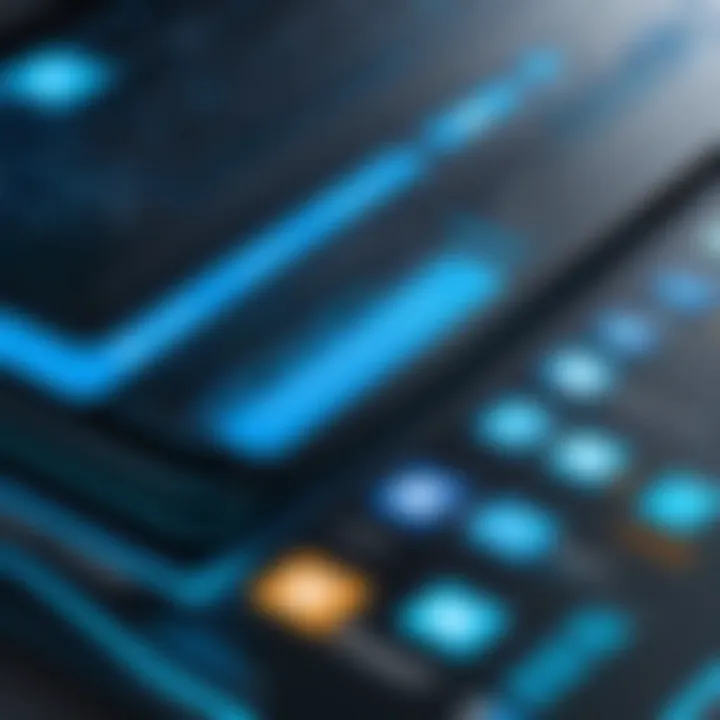
- Complex Pricing Structure: The pricing model can be somewhat convoluted. Potential users might find it difficult to assess total costs until they gather enough historical usage data to predict their needs accurately. Many variables, such as ingress and egress data and job complexity, can impact pricing.
- High Costs for Heavy Users: Organizations that require constant, heavy streaming analytics might find costs accumulating rapidly compared to other solutions which might offer more predictable flat rates.
- Dependence on Data Egress Costs: Users might face unexpected bills if they frequently transfer data out of the Azure region. Revenue generation from data transfer can add considerable expenses to projects, which is vital to consider before a commitment.
- Limited Features Compared to Alternatives: Though Azure Stream Analytics is robust in real-time analytics, some alternative solutions might offer advanced features like better support for machine learning or data visualization tools, which could be crucial for some organizations.
"Choosing the right streaming analytics solution isn’t just about features; it’s about finding the perfect fit for your unique needs and budget."
In summary, understanding Azure Stream Analytics involves recognizing its strengths as well as its pricing pitfalls compared to alternatives. This evaluation allows stakeholders to see if Azure aligns with their project requirements and budget constraints. By weighing these factors carefully, businesses can arrive at decisions that are well-informed and strategically beneficial.
Real-World Applications
Azure Stream Analytics serves as a pivotal tool in today’s data-driven world, with applications across numerous industries, each harnessing its capabilities in unique ways. Understanding these real-world applications is not just helpful; it’s essential for tech professionals looking to visualize the potential impacts on their specific domains. Businesses can make informed decisions on how best to leverage Azure's features, minimizing costs and maximizing benefits in practical settings.
Industries Utilizing Stream Analytics
A variety of sectors find value in implementing Azure Stream Analytics due to its flexibility and robust features. Here are several key industries:
- Finance: Banks and financial institutions utilize real-time analytics to monitor transactions for fraud detection. They need instantaneous feedback, which is vital for safeguarding customer data.
- Healthcare: This industry employs stream analytics to track patient data in real-time, aiding in monitoring health parameters for critical patients. By analyzing streams of data as it arrives, healthcare providers can improve patient outcomes and reduce hospital costs.
- Retail: Retailers use stream analytics to personalize customer experiences by analyzing shopping patterns on-the-fly. This allows businesses to adjust stock levels and launch promotions quickly based on consumer behavior.
- Telecommunications: Telecom companies exploit Azure Stream Analytics for network performance monitoring, analyzing call data for better service delivery. They enhance customer satisfaction by identifying and resolving issues promptly.
- Transportation and Logistics: This industry benefits from tracking vehicles and shipments in real-time, ensuring that operations run smoothly and efficiently.
Stream analytics can significantly streamline operations, providing insights that lead to better decision-making and cost efficiency.
Case Studies on Pricing Impact
It's essential to look at how real businesses experience the cost implications of Azure Stream Analytics. For example:
"A major retail chain implemented Azure Stream Analytics to better predict inventory needs during peak shopping seasons. They noted a decrease in overstock, saving approximately 20% on storage costs just in the first quarter."
In another instance:
- Healthcare Provider: A healthcare organization invested in Azure Stream Analytics to monitor patient vitals. Their initial costs seemed steep, but by effectively utilizing the platform, they reduced patient transfer times significantly, resulting in cost savings that surpassed their initial investment within a year.
By examining these case studies, it's clear that the pricing of Azure Stream Analytics can lead to substantial returns when applied correctly. Businesses can tailor the service according to their unique requirements, leading to a better cost-to-benefit ratio overall.
Through understanding these real-world applications, professionals and companies can envision how Azure Stream Analytics might fit into their frameworks, allowing for more strategic planning and investment.
Future Trends in Azure Stream Analytics Pricing
The landscape of cloud computing and real-time analytics is consistently evolving, forcing organizations to adapt their strategies and budget estimations. The future of Azure Stream Analytics pricing holds significant relevance in understanding how businesses can utilize this powerful tool while keeping a close eye on costs. It's not just about the present pricing models, but also about anticipating potential changes that could affect long-term investments.
Understanding future trends in pricing allows organizations to prepare for shifts in technology, capacity, and resource consumption. A proactive approach in assessing these trends provides a competitive edge amid fluctuating market forces and tech advancements. Here's what makes this a crucial area of study:
- Anticipating costs: Organizations can better manage their budgets by forecasting potential price increases or adjustments.
- Maximizing resource usage: Awareness of trends encourages smarter allocation of resources, improving efficiency.
- Adopting emerging technologies: Keeping an eye on the horizon can lead to early adoption of beneficial solutions, enhancing overall operational capabilities.
Emerging Technologies and Their Implications
The rise of new technologies invariably impacts the pricing structures of existing platforms. As Azure Stream Analytics integrates with advanced solutions like Artificial Intelligence and Machine Learning, pricing may reflect the additional features and capabilities that come along with these innovations. For instance, one could see how enhancements such as automated scaling and more robust data processing might shift costs in new directions.
Some potential ramifications include:
- Variable pricing models: As features expand, Microsoft might introduce more flexible pricing options, such as tiered structures based on the level of AI integration.
- Cost-benefit analyses: As new technologies emerge, businesses will need to conduct solid cost analyses to ensure that the gains from advanced capabilities justify any price hikes.
- Competitive dynamics: Innovations could accelerate competition among analytics platforms, forcing Azure to adjust its pricing to remain attractive against alternatives.
Potential Pricing Adjustments
Anticipating specific pricing adjustments is as important as understanding the technologies driving them. The Azure team listens to customer feedback and market demands, which often leads to shifts in pricing strategies. Such adjustments could be driven by:
- Value-based pricing: If new features significantly enhance the utility of Azure Stream Analytics, we might see a transition toward a value-based approach, where prices are tied directly to the value delivered.
- Usage patterns: Monitoring how organizations use the service can provide insights that allow Microsoft to adjust rates based on usage efficiency and provide incentives for optimization.
- Regulatory changes: As data regulations evolve, there might be pressure to increase transparency in pricing or adapt charges based on compliance costs.
"The only constant is change." - Heraclitus. In the context of Azure Stream Analytics, this wisdom rings true. Understanding emerging technologies and pricing adjustments is essential for any business aiming to navigate the complexities of pricing structures in the realm of real-time analytics.
Finale
As we wrap up our exploration of Azure Stream Analytics pricing, it's vital to reflect on the intricacies that govern this area. Grasping the pricing model isn’t merely about knowing costs; it’s about equipping oneself to navigate a sea of data streams efficiently and economically. The insights gained from this analysis can have a significant impact on how organizations manage their resources and budget for cloud solutions.
Recap of Key Pricing Insights
The pricing structure hinges on multiple factors. Here’s a quick rundown:
- Consumption-Based Pricing: Costs are directly related to usage; organizations pay only for what they process.
- Fixed Pricing Options: Certain scenarios may favor a flat-rate pricing model, which helps in budget predictability.
- Variable Data Ingestion Rates: The amount of data ingested impacts costs, necessitating a keen eye on data volume trends.
- Job Complexity and Management: More intricate jobs require greater computational power, which affects overall pricing.
Understanding these dynamics allows businesses to align their strategies in a way that maximizes resource efficiency while mitigating costs.
Final Thoughts on Cost Management
In the end, effective cost management is a blend of keen awareness and strategic planning. Organizations should regularly review their data consumption and job setups. Keep the following principles in mind:
- Regular Monitoring: Use Azure’s built-in tools to track usage patterns and adjust resources dynamically.
- Budgeting Techniques: Establishing a solid budgeting procedure based on historical data will help. Consider how aspects like data volume and job complexity influence your costs.
- Optimization Focused on Efficiency: Find ways to simplify data workflows—this reduces the chances of incurring unplanned expenses.
By staying informed about market trends and leveraging these cost management techniques, organizations can navigate Azure Stream Analytics pricing with confidence. It’s not just about understanding numbers, but also about harnessing data-driven insights that inform strategic decision-making.