Comprehensive Review of JMP Data Analysis Software
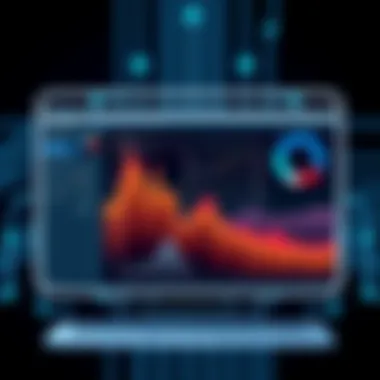
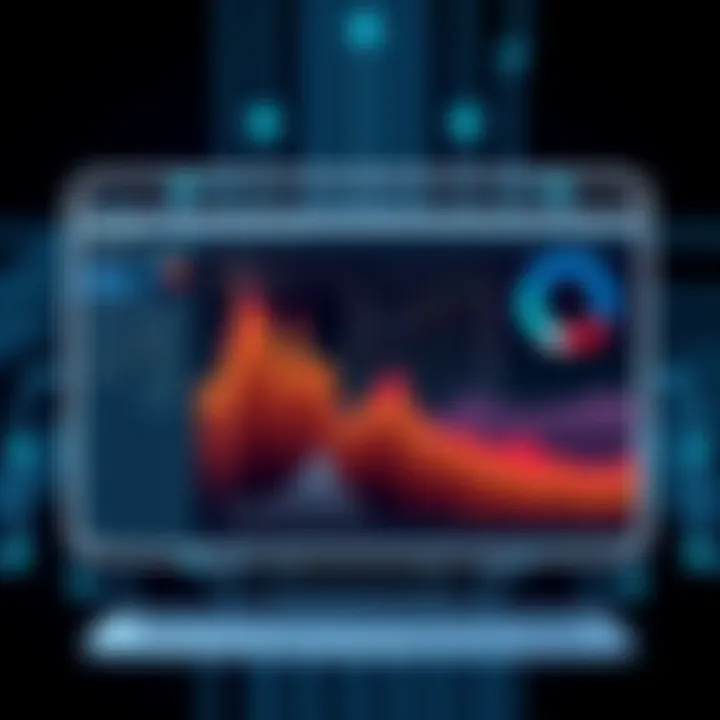
Intro
In the world of data analysis, the right tools can make all the difference. JMP data analysis software stands out as a robust option for those who dive deep into data. Whether one is a seasoned analyst or a curious student, JMP offers a blend of features that cater to diverse needs. This article will explore the underlying strengths, practical applications, and potential benefits of the software, shedding light on its user-friendly interface and powerful capabilities.
Many users are blown away by JMP's ability to handle complex datasets and provide insightful visualizations. Not to mention, the software is widely used across various industries, from academia to manufacturing, making it a versatile choice for different users. By the end of this exploration, you will gain a deeper understanding of how JMP can enhance your data analysis tasks, beyond just crunching numbers. Let's delve into its intricacies and uncover what sets JMP apart from the rest.
Overview of JMP Data Analysis Software
Understanding JMP Data Analysis Software is crucial for those who aim to leverage data effectively. JMP stands out as a powerful tool, merging statistical analysis with dynamic data visualization, allowing users to dig deeper into their datasets. In this section, we’ll explore the significance of JMP, its evolution, and the broad spectrum of industries that benefit from its robust features.
Preface to JMP
JMP is developed by SAS Institute, designed to assist professionals in various fields in making data-driven decisions. Launched in 1989, it has continuously evolved to adapt to the ever-changing landscape of data analysis. Its name originates from the acronym "John’s Macintosh Project," as it was initially developed for the Mac. Users appreciate JMP for its intuitive interface and the ability to conduct sophisticated statistical analyses without extensive programming knowledge. It provides an interactive environment where insight generation can happen seamlessly, making data analytics approachable for various skill levels.
History and Development
JMP has an interesting lineage, tracing its roots back to the late 1980s. Initially created to cater to the emerging need for a graphical data analysis tool, it has grown in functionality over the decades. The software has seen significant improvements with each release, including integrations with newer technologies, enhancements to user experience, and updates to statistical methodologies. For instance, with the rise in big data, JMP has incorporated advanced analytics, making it suitable for contemporary data challenges.
Such evolution is not just limited to technical upgrades; the community around JMP has also flourished. From user forums to extensive documentation, the support ecosystem is almost as rich as the software itself. This development narrative exemplifies how JMP has constantly adapted to serve its users better, ensuring it remains relevant in an increasingly data-driven world.
Target Users and Industries
JMP caters to a diverse audience. Its primary users include data analysts, researchers, and scientists who operate across a plethora of industries. From pharmaceutical companies relying on statistical methods to ensure drug safety, to industrial engineers conducting experiments for quality improvement, JMP proves its versatility.
Some key industries utilizing JMP include:
- Healthcare: For clinical trials and epidemiological research.
- Manufacturing: To streamline processes through design of experiments.
- Education: Supporting academic research and helping students grapple with statistics.
- Finance: Assisting analysts in risk assessment and market analysis.
With such a wide-ranging applicability, JMP serves as an indispensable tool for professionals looking to harness the full potential of their data.
"In the world of data analysis, having the right tools can make all the difference between guesswork and informed decision-making."
Core Features of JMP
In the realm of data analysis, the phrase "data speaks" can sometimes feel more like an echo in an empty room without the right tools to bring those insights to life. JMP data analysis software stands out through its core features that not only enhance the clarity of data interpretation but also cater to a diverse user base ranging from students to seasoned analysts. The importance of these core features lies in their ability to transform complex datasets into discernible trends and actionable insights. As we delve deeper into this section, we'll uncover the essential elements that make JMP a leading choice for professionals navigating the intricate waters of data analysis.
Data Visualization Tools
Interactive Graphs
Interactive graphs within JMP are a game changer in visual data analysis. These tools allow users to engage with their data in real-time, rather than just observing static images. A distinct feature of interactive graphs is their ability to produce dynamic overlays—think of them as layers of information that come alive as one hovers over data points. Notably, this feature is beneficial because it enables users to drill down into specifics without getting lost in a sea of numbers.
"Interactive graphs improve understanding by marrying the visual with the analytical."
While they are undeniably helpful, the reliance on interaction might pose challenges for those unaccustomed to navigating complex visual data. Additionally, the initial learning curve can intimidate some users who need more straightforward visualization methods.
Dynamic Reports
Dynamic reports take the concept of static report generation and flip it on its head. Instead of presenting rigidly formatted pages, these reports adapt and present live data analytics, offering up-to-date insights. A significant advantage of dynamic reports is their customizable nature; users can modify them on-the-fly to reflect changes in datasets or analytic perspectives.
However, this adaptability can sometimes lead to information overload. Users may find themselves overwhelmed by endless options to manipulate and explore data visuals, which could lead to confusion if not managed wisely.
Customized Plots
Customized plots are the icing on the cake for JMP users looking to tailor their data presentations. Users can create unique visualizations that specifically align with their analysis needs, from scatter plots to box plots and more. The key characteristic here is flexibility—users can configure elements such as color, scale, and data shape to emphasize particular trends and findings.
While customized plots offer significant advantages in personalization, they can also require a bit of an investment in terms of time and skill to master. New users, in particular, might find themselves needing a hand navigating the myriad of customization options available.
Statistical Analysis Capabilities
Descriptive Statistics
Descriptive statistics form the backbone of any good data analysis. JMP excels in providing detailed summaries of datasets, covering vital metrics such as mean, median, standard deviations, and more. One attractive aspect of descriptive statistics in JMP is the immediate visualization provided alongside numerical summaries, allowing users to see patterns that simply observing numbers might miss.
This integration is synergistic—data stories emerge more clearly through visual representation. However, for complex datasets, relying solely on descriptive statistics can be misleading if the subtleties of the data are overlooked.
Inference Techniques
Inference techniques in JMP open up portals for hypothesis testing and confidence intervals. Users can delve into inferential statistics, determining whether their sample results can be generalized to a larger population. This capability is crucial for researchers looking to make evidence-based claims. The unique feature of JMP's inferential tools is their comprehensive support for multiple forms of hypothesis testing, making it a robust resource.
However, users should apply these techniques thoughtfully, as misinterpretation of results can lead to erroneous conclusions—a pitfall illustrated by the phrase "correlation does not imply causation."
Regression Analysis
Regression analysis is pivotal for modeling relationships between variables, and JMP doesn’t skimp here. It offers multiple regression frameworks—from linear to logistic regression. Highlighting the key characteristic of its regression tools is the ability to perform diagnostics, which ensures the validity of the model assumptions. This transparency is a significant benefit for users wanting to ensure the reliability of their models.
Nevertheless, users need to be wary of overfitting their models. Over-reliance on regression without proper data validation can obscure underlying truths and lead to misguided recommendations.
Scripting and Automation Functions
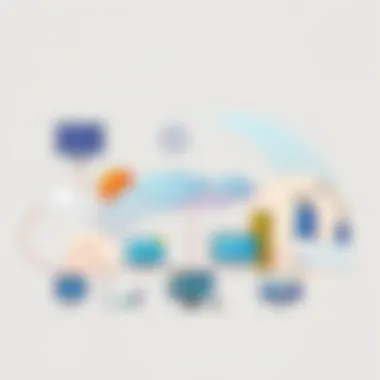
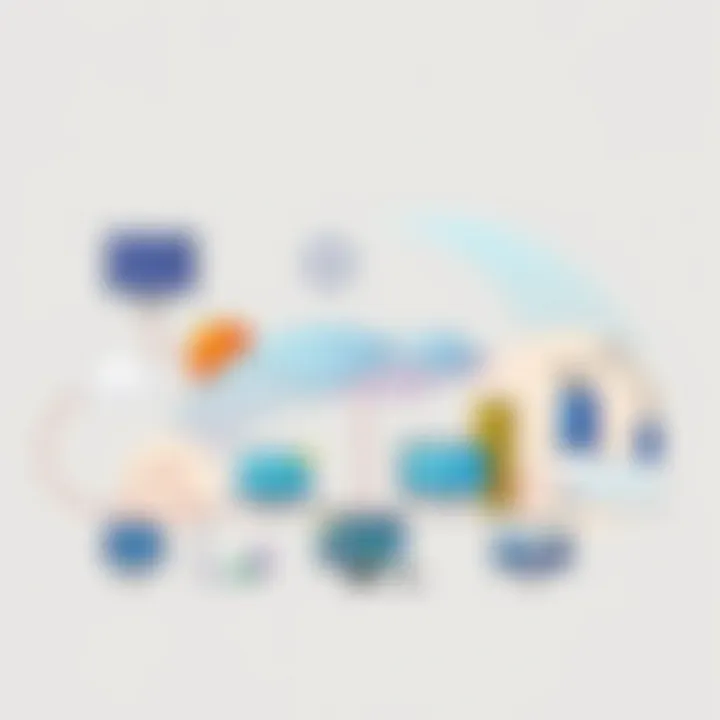
JSL Language Overview
The JMP Scripting Language (JSL) is a powerful tool enabling users to automate repetitive tasks and develop complex analyses. JSL provides flexibility and control, allowing users to tailor their workflows to suit specific needs. One key characteristic of JSL is that it grants access to deeper functionalities within JMP, which are often hidden from the graphical user interface.
While the learning curve can be steep for non-programmers, gaining proficiency in JSL can significantly enhance one's efficiency and capability in handling complex analyses. It’s a bit like learning to ride a bike: the initial struggle pays off in the long run.
Creating Custom Scripts
Custom scripts in JMP take advantage of JSL to create personalized analytical workflows. Users can script everything from simple data manipulations to complex simulations, significantly reducing time spent on analytical tasks. The unique feature of this is the ability to save these scripts for future use, creating a repository of reusable workflows.
However, the complexity involved in scripting may deter some users. Those without programming backgrounds might initially struggle but the effort tends to make the experience far more rewarding.
Automation Workflows
Automation workflows in JMP represent the pinnacle of efficiency in data analysis. Through JSL, users can schedule tasks, run overnight analyses, and automate reporting processes. The key benefit here is the tremendous time saved, allowing analysts to focus on interpreting results rather than manual data processing.
But, as with any automated system, there’s an inherent risk: if errors occur in automation scripts, they might not be detected until it’s too late, leading to potentially significant data misinterpretations. A well-placed check in the workflow can serve as a checkpoint, ensuring that automated systems yield accurate results.
Advanced Analytics and Modeling
In the realm of data analysis, advanced analytics and modeling hold a pivotal place. These techniques allow users to go beyond traditional reporting, delving deeper into data to extract meaningful insights and forecast outcomes. For users of JMP, this feature is especially significant as it enhances the software's capabilities, providing them with the tools needed to handle complex data scenarios effectively.
When we talk about advanced analytics, we are often referring to methodologies like predictive modeling and experimental design. These processes enable professionals to make educated decisions based on data-driven forecasts instead of gut feelings or historical trends. JMP empowers users with such tools, providing them the ability to apply sophisticated analytical techniques to their datasets. The result is a more robust understanding of data relationships, trends, and potential outcomes.
To break it down further, advanced analytics encompasses predictive modeling techniques alongside the design of experiments. Each of these elements brings unique advantages. For instance, the predictive modeling techniques help in identifying patterns and making forecasts, while design of experiments enables a structured approach to testing hypotheses and discovering interactions among variables.
Predictive Modeling Techniques
Predictive modeling techniques in JMP create pathways to forecast future events based on historical data. The ability to anticipate outcomes is invaluable for many sectors, allowing users to strategize and minimize risks.
Decision Trees
One of the most popular techniques in predictive modeling is the decision tree. This method is appreciated for its simplicity and effectiveness. The key characteristic of decision trees is that they break down complex decisions into a series of simpler decisions. Each branch of the tree represents a possible outcome, greatly helping in visualizing the decision-making process.
Unique Feature: Besides its straightforward visual representation, decision trees can handle both categorical and continuous data, making them versatile. However, they can be prone to overfitting, leading to models that may not generalize well to new data if not managed carefully.
Neural Networks
Neural networks represent another sophisticated method in predictive modeling, gaining traction due to their ability to uncover intricate patterns in large datasets. The fundamental characteristic of neural networks is their layered structure, mimicking the human brain, which allows them to learn from vast amounts of data.
Unique Feature: Neural networks excel in tasks like image and speech recognition. Despite their power, they require significant amounts of data and processing, which can be a drawback for smaller datasets or less powerful computing environments. Understanding the intricacies of configuring these networks is essential for maximizing their potential.
Support Vector Machines
Next up is the support vector machine (SVM), a preferred choice among many data scientists for its efficiency in classification tasks. The standout characteristic of SVM is its ability to find the optimal hyperplane that separates classes in feature space.
Unique Feature: SVM is particularly beneficial for high-dimensional spaces and can perform well with a clear margin of separation. However, the main drawback is that it can be less effective when the data is noisy, and selecting the right kernel function is crucial for optimal performance.
Design of Experiments (DOE)
Moving on to another essential component in JMP, the design of experiments (DOE) plays a vital role in structured testing and simulation. DOE encompasses various techniques to ensure efficient gathering of data that leads to well-founded conclusions. By systematically varying factors, DOE helps uncover relationships between input variables and output responses.
Preamble to DOE
The introductory principles of DOE are essential for conducting robust experiments. It emphasizes the importance of planning and structuring experiments to get the most pertinent data. The significant characteristic of this approach is its systematic way of testing multiple variables simultaneously, rather than isolating them.
Unique Feature: This holistic perspective often results in discovering interactions that might be missed in simpler experimental designs, but its complexity might require a steeper learning curve for newcomers.
Creating Experiments
Creating experiments within JMP enables users to set up their experimental designs efficiently. The characteristic of this process is the ability to utilize software tools to optimize how factors and levels are combined.
Unique Feature: This streamlined process allows for quicker hypothesis testing, but care must be taken to ensure that factors are chosen thoughtfully to avoid confounding variables.
Analyzing Results
Finally, analyzing results is the concluding chapter of DOE. This segment is crucial, as it turns raw data into actionable insights. The key characteristic of result analysis in DOE is its focus on determining the significance of the effects observed.
Unique Feature: JMP provides various tools for interpreting experimental data, which aid in visualizing outcomes. However, the complexity of analysis requires a sound understanding of statistical methods to draw correct conclusions.
Integration with Other Tools
In today's data-driven landscape, utilizing data analysis software like JMP is only part of the story. The real magic happens when JMP integrates seamlessly with other tools and platforms. Integration with databases, APIs, and cloud services not only enhances the functionalities of JMP but also drastically improves data handling capabilities. Whether it’s extracting insights from large datasets or collaborating with teammates remotely, integration is the cornerstone of effective data analysis. In this section, we will explore how connecting to databases and APIs, as well as collaborating with cloud services can elevate your experience with JMP.
Connecting to Databases and APIs
Database Connections
When you hear "database connections," think of bridging the gap between your JMP environment and the wide world of information stored in databases. The primary benefit of establishing database connections is the ability to access large volumes of data directly, which can simplify the analysis process and reduce errors that often arise from manual data importation.
A standout feature of JMP's database connection capability is its support for various database management systems, such as MySQL and Microsoft SQL Server, which makes it versatile. This flexibility means users can work with different types of data without getting bogged down in format conversions.
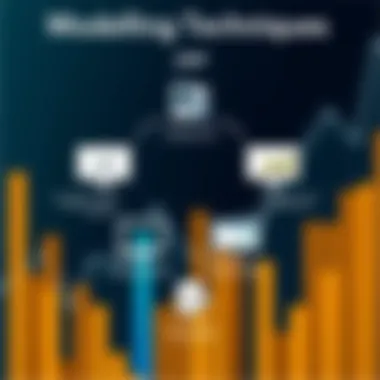
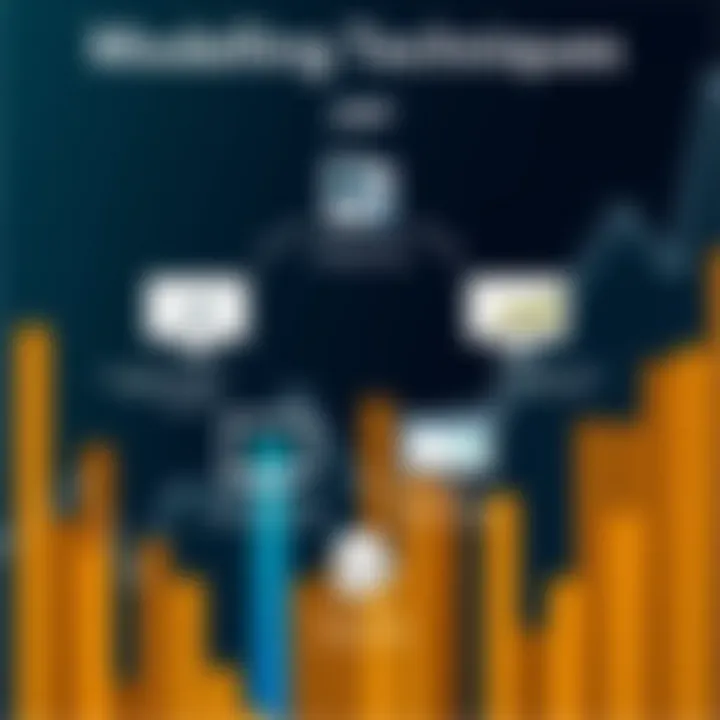
However, while the advantages are clear, there are also drawbacks to consider. Database connections might require some initial setup and configuration knowledge, which can be daunting for users who are not tech-savvy. But once set up, the ability to pull live data into JMP for analysis is undoubtedly beneficial.
API Integrations
APIs, or Application Programming Interfaces, are the unsung heroes in the tech world. They allow different software applications to communicate with each other. In the context of JMP, API integrations enable you to pull data from various sources, including web services and third-party applications.
A key characteristic of API integrations is their dynamic nature. With real-time data access, users can perform analytics on the fly, which is a game changer for businesses that thrive on timely insights. This functionality sets it apart and makes API integration a popular choice for data professionals.
Yet, with great power comes potential pitfalls. Depending on your familiarity with APIs and their documentation, the setup process may vary in complexity. Some users might find the learning curve steep, so adequate training materials should be utilized for a smoother experience.
Data Importing Techniques
Data importing techniques in JMP are vital for transitioning data from other sources into the software. The ease with which you can import data factors heavily into how effectively you can analyze it. JMP allows for various importing solutions, from spreadsheets to more intricate database pulls, giving users flexibility.
Among the unique features of JMP’s importing techniques is the support for multiple data formats. This means users can comfortably work with CSV, Excel, and other common file formats without fussing over conversions. This flexibility ultimately saves time and streamlines the entire analysis workflow.
On the flip side, not all data can be imported seamlessly. Certain formatting requirements need to be adhered to, or users might face data integrity issues. Proper attention to detail during the initial import phase is crucial to mitigate these challenges.
Collaborating with Cloud Services
In a world where remote work is the norm, collaborating with cloud services is becoming increasingly important. JMP does not fall short in this area, allowing users to collaborate efficiently with integrated cloud storage solutions and remote collaboration tools.
Cloud Storage Solutions
Cloud storage solutions paired with JMP enable users to store and share data effortlessly. The immediacy of accessing files from anywhere significantly enhances collaborative endeavors, especially in team-oriented analyses.
A key feature of these storage solutions is the capacity for version control. This capability allows multiple users to work on the same data files while keeping previous versions intact, which is crucial in environments where iterative analysis is common.
However, users must remain aware of data security concerns when sharing sensitive information via cloud services. As convenient as it is, ensuring proper security protocols are in place is essential to safeguard your data.
Remote Collaboration Tools
The rise of remote collaboration tools means teams can work together without being in the same room—or even the same country. JMP’s integration with various remote collaboration platforms helps facilitate discussions and analysis sharing.
The standout aspect of using these tools is the ability to conduct real-time discussions alongside your data analysis. This synchronization creates an interactive environment where insights can be generated and discussed seamlessly.
Again, there are considerations to keep in mind. Selecting the right remote tools that integrate smoothly with JMP takes some research. Time spent in setup can initially feel like a burden, but the potential efficiency gains are well worth it in the long run.
"In data analysis, integration isn’t just an add-on; it’s a necessity. It unifies disparate data points and allows for a holistic view that single software solutions can’t always provide."
As we dissect the importance of integration with other tools, it becomes clear that JMP is not just powerful on its own—its real potential shines through these connections, be they via databases, APIs, or cloud services. Leveraging these integrations can significantly enhance the overall data analysis experience.
User Experience and Interface
User experience (UX) and interface design are critical components of any software, including JMP. A well-designed interface not only enhances usability but also significantly impacts productivity and user satisfaction. In the realm of data analysis, where complex tasks need to be managed, a clear and intuitive interface becomes even more paramount. Users must navigate various tools and menus seamlessly; otherwise, they can become bogged down by frustration, which might result in overlooking important insights from their data.
User Interface Design
Layout and Navigation
The layout and navigation within JMP are intuitively structured to accommodate both beginners and seasoned analysts alike. The design promotes an organized workspace, where functionalities related to data analysis are logically grouped. A major characteristic of this layout is its use of a ribbon menu at the top, which dynamically populates based on the selected data or task.
This characteristic is beneficial because it allows users to find necessary tools without sifting through endless menus. However, the unique feature of quick-access buttons scattered throughout the interface can also work against some users who may prefer keeping everything tightly grouped. This means while the layout offers flexibility, it can sometimes create a divide between user preferences and the software's design philosophy.
Customization Options
Customization options in JMP stand tall as a significant feature. Users can tweak the interface to mirror their foreign preferences, which is vital for creating a personalized working environment. This ability to customize can mean changing toolbars, selecting default graph types, or designing workflow templates that align with specific analytical processes.
Not only does this customization empower users, but it also heightens efficiency by streamlining repeated tasks. Nonetheless, this feature demands a time investment upfront to set up, and some users may feel overwhelmed by the variety of choices available when starting out.
Learning Curve and Support Resources
Training Materials
Training materials provided by JMP are robust and diverse. They range from video tutorials, extensive webinars, to step-by-step documentation that guides users through different functionalities. This extensive support is pivotal for anyone aiming to harness JMP's full potential.
The standout aspect of the training materials is the real-world examples that translate theoretical concepts into practical applications. For instance, users can find case studies that detail how certain statistical techniques were applied in actual projects. This not only solidifies understanding but also enhances retention. Although, newcomers might feel a little daunted by the breadth of resources available, navigating them can lead to a significant return on investment in terms of knowledge gained.
Community Support
Community support serves as a fundamental backbone for JMP users. The forums and discussion boards are brimming with insights, allowing users to ask questions and receive detailed answers from experienced members. This peer support fosters a sense of camaraderie while also providing a shortcut to problem-solving.
The unique feature of user-generated content, such as tips and tricks shared in various online groups, cannot be understated. This resource is invaluable; however, sometimes the quality of advice can be inconsistent. New users must navigate this landscape carefully, validating the suggestions they receive.
Documentation Availability
Documentation availability is a strong suit of JMP as it delivers comprehensive manuals and resources that users can consult at any stage of their learning journey. The user manuals detail everything from setup instructions to advanced analytical techniques. Highlighting the key characteristic of this documentation is its organizational structure. Users can easily find information categorized by topic and functionality, which saves significant time.
One standout aspect in this realm is the online documentation search feature. It allows users to input keywords and immediate access relevant sections. However, while the manuals are detailed, some users have noted they can be overly technical in nature, which may lead to confusion for those without a strong analytical background.
Overall, the user experience and interface in JMP play a crucial role in empowering users to perform advanced data analysis efficiently. With its well-crafted design, extensive training resources, community support, and thorough documentation, JMP effectively supports its users in traversing the complex landscape of data analytics.
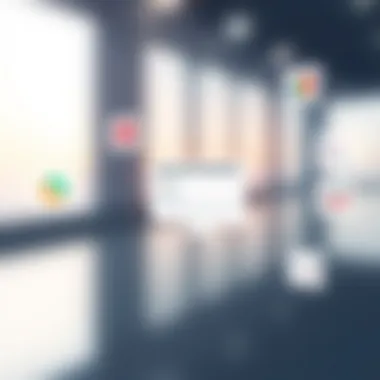
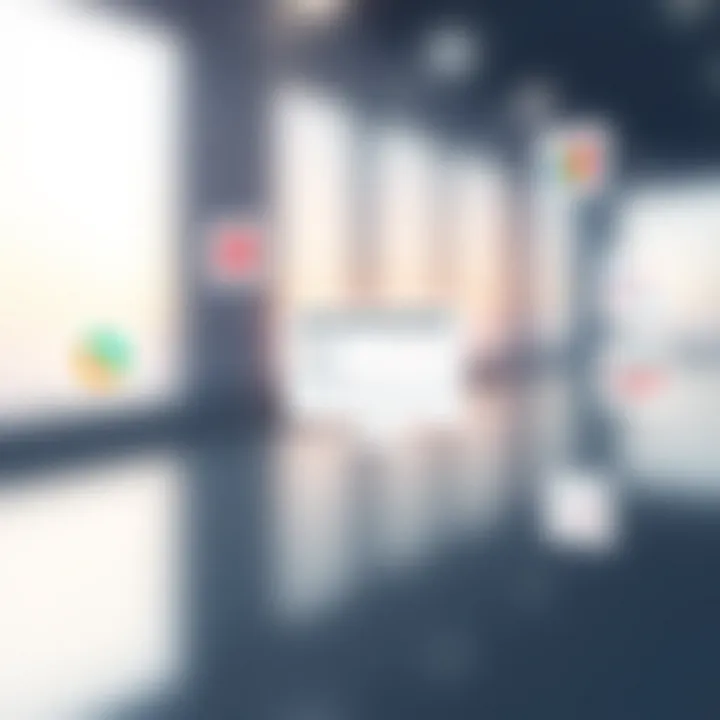
Pricing and Licensing Options
When exploring software like JMP, understanding the pricing and licensing options is crucial for making informed decisions. This section sheds light on various purchasing models, addressing the potential benefits and considerations that come with them. The right licensing agreement not only impacts budget constraints but also affects software usability across different user profiles. For example, a small business may require a different approach compared to an academic institution. Thus, analyzing these options can aid potential users in selecting a plan that aligns with their organizational needs and financial resources.
Standard Licensing Models
In terms of standard licensing, JMP typically offers several models, tailored to various types of users. Each model possesses unique characteristics that can suit different environments:
- Single-user License: This straightforward approach allows a single user to install and run the software on one machine. It’s ideal for individual analysts or consultants who do not require collaboration with others.
- Multi-user License: A multi-user license enables multiple employees within an organization to use JMP simultaneously, usually through a shared server. This can be advantageous in settings with collaborative projects.
- Site License: For larger organizations or institutions, purchasing a site license might be the most economical choice. This allows unlimited installations across all devices within a specific location, making it efficient for consistent software access.
The cost varies widely based on the licensing type. Therefore, it's wise to consider how many users will be using the software and their specific needs, striking a balance between cost-effectiveness and access.
Educational and Institutional Pricing
Educational pricing is one area where JMP truly shines, reflecting its commitment to supporting learning and research. Institutions often have access to specially discounted rates, making the software more affordable for students and academic bodies.
Some noteworthy aspects include:
- Significantly Reduced Prices: Students and educators can often receive substantial discounts, lowering initial barriers to entry.
- Free Trials and Grants: Certain educational institutions may have the chance to acquire free trials or grants from JMP, further enhancing access to the software.
- Training Resources: Many educational licenses come bundled with access to additional training materials and community resources, fostering skill development in statistical analysis and data visualization.
JMP’s focus on educational pricing not only supports institutions but also nurtures the next generation of data scientists and analysts. It represents an investment in the future, as academic exposure can lead to increased proficiency and deeper analytical capabilities among students.
"The proper use of data analytics not only empowers organizations but also shapes the way future professionals engage with complex datasets."
By wrapping this piece up, potential users should now have a clearer understanding of how pricing and licensing options influence the overall usability and accessibility of JMP, tailoring the experience based on specific user contexts.
Comparative Analysis with Other Software
In the realm of data analysis, relying on a single tool can limit one’s ability to harness the full potential of available data. That’s where a comparative analysis comes in handy, as it allows one to meaningfuly evaluate various software options and find the best fit based on specific requirements. JMP, with its slew of features, invites comparisons with other data analysis platforms like SAS, R, and SPSS, among others. By weighing strengths and weaknesses, users can make informed decisions that align with their data handling needs.
Strengths of JMP
JMP stands out for several reasons, making it a preferred choice among professionals in various sectors. Firstly, its user-friendly interface simplifies complex tasks, lowering the barrier to entry for users who may not be seasoned data analysts. The combination of point-and-click functionalities and robust scripting capabilities means it serves both beginners and advanced users well.
- Dynamic Data Visualization: One of JMP's major advantages lies in its exceptional data visualization tools. Users can instantly create a range of interactive graphs, from simple scatterplots to intricate heatmaps, ensuring that data storytelling becomes intuitive and accessible.
- Integrated Statistical Methods: JMP is not just a visualization tool. It incorporates a comprehensive suite of statistical methods that cover descriptive statistics, fitting models, and more, all of which are wrapped in an easy-to-use interface and guided exploration.
- Real-Time Data Manipulation: The ability for analysts to interact with data in real-time—refreshing visual outputs and statistical calculations as data changes—enhances decision-making processes and significantly speeds up data exploration.
Most noteworthy, JMP integrates seamlessly with numerous other systems and databases. Users can connect directly to enterprise-level databases or cloud services, enhancing overall workflow efficacy.
Limitations and Areas for Improvement
While JMP shines in many categories, it’s not without its shortcomings. Understanding these limitations is essential for making an informed choice. Some areas where JMP could improve include:
- Cost Considerations: JMP is often perceived to be on the pricier side compared to alternatives such as R and Python, which are both open-source. For small businesses or startups, this can represent a significant hurdle.
- Learning Curve for Advanced Features: Though it claims to be user-friendly, users may experience a steep learning curve when attempting to leverage its more advanced features, particularly for scripting with its proprietary JMP Scripting Language (JSL).
- Limited Customization in Certain Visuals: While JMP excels at generating high-quality visual output, there may be instances where customization is not as flexible compared to competitors which offer more extensive programming capabilities for tailored visuals.
"Every software has its charms and tricks; knowing which to dance with is the game of savvy users."
By evaluating JMP in light of its strengths and limitations relative to other data analysis software tools, users are better positioned to choose the right software that aligns with their analytical goals.
Case Studies and Application Scenarios
In today's rapidly evolving data landscape, understanding how a tool like JMP can be applied practically is crucial. Real-world examples of its use not only underscore its flexibility and power but also serve as valuable learning experiences for practitioners. By analyzing case studies and application scenarios, we can gain insight into how JMP stands up to real challenges in diverse fields. It is particularly vital for software developers, IT professionals, and students to see tangible outcomes from their work. This section delves into two main areas: the significance of JMP in academic research and its application across various industries.
Use in Academic Research
The academic realm often demands rigorous data analysis, and JMP has cemented its place as a critical component in many research projects. Universities around the globe rely on JMP for its robust statistical capabilities and visualization tools that facilitate in-depth exploration of data. Researchers can quickly employ complex models without diving too deep into coding, thus allowing them to focus more on their research questions rather than the nuances of software syntax.
For instance, a study at the University of California utilized JMP to analyze a large dataset concerning environmental changes. The researchers employed decision trees and regression analysis to draw correlations between various climate parameters and ecological outcomes. This helped them to efficiently identify trends without getting lost in the clutter of raw data.
More broadly, because JMP provides an interface that's relatively user-friendly, it's an attractive choice for students who are just getting started in data analysis. Training programs and coursework often use JMP due to its educational focus. Consequently, students can easily conduct hands-on experiments to solidify their understanding of theoretical concepts through practical application.
Applicable Industry Cases
JMP's versatility shines through its application in various industries. From healthcare to manufacturing, the software finds use across a spectrum of sectors. For example, in the pharmaceutical industry, JMP has been instrumental in clinical trials where data integrity can dictate the success or failure of new drugs. A pharmaceutical company, facing difficulties in processing vast amounts of trial data, turned to JMP for insights. Through its advanced analytics features, the company was able to expedite its development process, reducing overhead and increasing the speed to market for its new drugs.
Similarly, in the manufacturing sector, an automotive firm applied JMP to optimize its supply chain management. By leveraging JMP's predictive modeling technique, the company was able to forecast inventory needs more accurately, thus slashing waste and ensuring that resources were allocated efficiently. In both cases, firms harnessed JMP not just to analyze data but also to foster innovation and drive strategic decision-making.
"Using JMP not only saved our team time but also led us to insights we never would have discovered using traditional methods," shared a data analyst from the automotive sector who highlighted the software’s impact on operational efficiency.
In summary, case studies and application scenarios provide compelling evidence of JMP's capabilities and effectiveness. Whether in academic research or industry applications, the lessons learned from these instances serve as a guide for potential users, shedding light on both expected outcomes and best practices for data analysis.
Future Trends in Data Analysis
Data analysis is an ever-evolving landscape, where the future is shaped by advancements in technology and changing user needs. In this context, examining emerging trends becomes vital, not just for understanding current paths but for anticipating shifts that might impact analytical practices. For users of JMP data analysis software, keeping tabs on these trends means leveraging progressive tools and strategies that elevate their analytical prowess.
Emerging Technologies and Their Impact
As we look forward, several technologies are gaining traction that will reshape data analysis in profound ways. Here are some notable innovators to keep an eye on:
- Artificial Intelligence (AI) and Machine Learning (ML): These terms are used more often than a puppy chasing its tail. However, their applications within data analysis are genuine. AI algorithms can process vast datasets far more quickly than humans, providing insights that can lead to better decision-making. JMP can integrate AI tools to assist users in predictive modeling, making sense of complex patterns that might otherwise go unnoticed.
- Cloud Computing: Gone are the days when heavy data processing could only happen on a singular desktop. Cloud technologies enable users to access robust computing resources remotely. This shift allows for enhanced collaboration, real-time updates, and greater flexibility. JMP's collaboration with cloud platforms could facilitate smoother data sharing and increased efficiency in data analysis workflows.
- Big Data Analytics: An oldie but a goodie, the challenge of handling large datasets is consistently evolving. As more organizations generate data at unprecedented rates, tools that can efficiently analyze massive datasets are crucial. JMP must adapt and integrate features capable of handling big data, helping users unravel the narrative behind volumes of numbers.
- Internet of Things (IoT): In a world where devices are interconnected, the amount of data generated is skyrocketing. Data from IoT devices can provide unprecedented insights into real-world phenomena. JMP can offer specialized modules that analyze IoT outputs, helping industries better understand operational efficiencies.
"Staying ahead of technology trends is not just about embracing change, but about anticipating future needs and preparing for them."
JMP’s Role in Future Analytics
Looking ahead, JMP is poised to play a significant role in shaping future analytics. As the tools available evolve, so too must the software that users rely on. Some critical points worth considering include:
- Enhancing User Experience: Simple designs blended with sophisticated functions characterize future data analysis software. JMP is likely to emphasize user-centered design, streamlining interfaces to accommodate both novice and seasoned users.
- Integrative Functionality: As data sources diversify, the capability of JMP to connect and integrate various platforms will be crucial. The ability to seamlessly incorporate and analyze data from disparate sources can position JMP as a leader in the analytics field.
- Focus on Interactivity: As users increasingly desire hands-on experiences, says the experts in user engagement, platforms offering dynamic and interactive visualizations will gain an upper hand. JMP's historical strength in data visualization will serve it well in this regard, pushing for richer, more engaging user experiences.
- AI Features: With AI continuing to evolve, JMP can focus on embedding more AI-driven features that automate complex analysis tasks. This would free professionals to focus on higher-level decision-making and strategy.