Data Analytics Tools and Trends for Business Success

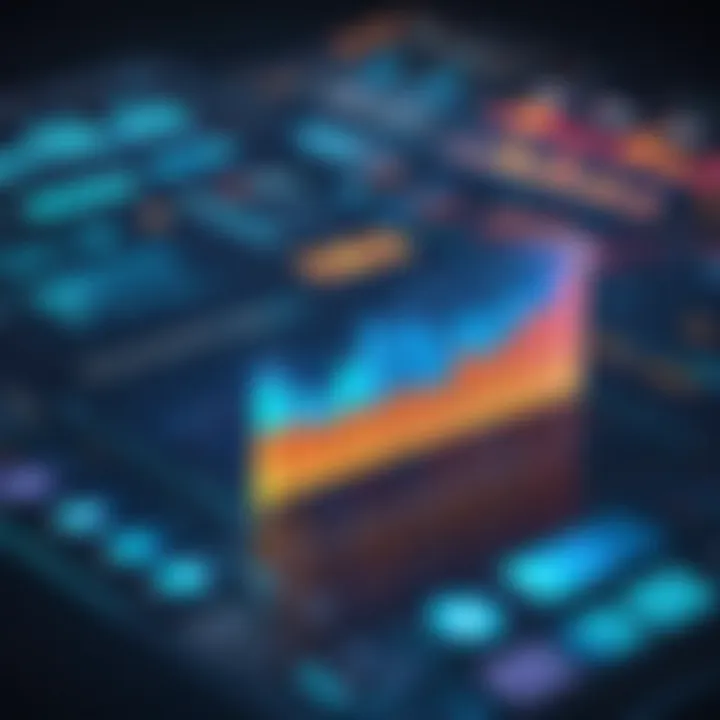
Intro
In today's fast-paced world, the sheer volume of data generated daily poses both challenges and opportunities. Understanding how to effectively analyze this data becomes paramount for decision-makers in various sectors. This guide dives into the applications of data analytics, focusing on the tools and techniques that power this essential practice. Whether you're a software developer seeking relevant tools, an IT professional staying abreast of industry trends, or a student eager to learn, this comprehensive breakdown is tailored for your needs.
Software Overview
Data analytics software has burgeoned in recent years, reflecting the growing necessity for efficient data handling and insightful analysis. The right software can illuminate pivotal trends and inform strategic decisions.
Key Features
When seeking data analytics software, here are crucial features to consider:
- Data Visualization: The ability to convert complex data into understandable visual formats—like charts, graphs, and dashboards—is essential for clear communication of insights.
- Data Processing: Look for tools that efficiently handle both structured and unstructured data, ensuring that information from varied sources can be cohesively analyzed.
- Collaboration Tools: Given the often interdisciplinary nature of data analytics projects, software that allows for easy sharing and joint analysis can significantly enhance workflow.
- Integration Capabilities: Software that can seamlessly connect with existing systems, databases, and other applications will save time and reduce frustration.
System Requirements
Before committing to a specific software option, it's vital to understand the system requirements. Key considerations include:
- Operating System Compatibility: Ensure the software runs smoothly on your operating system, whether it’s Windows, macOS, or Linux.
- Hardware Specifications: Some data analytics tools require higher processing power or memory, especially for large datasets. Consider the specifications of your machines.
- Network Requirements: With many applications being cloud-based, adequate internet connectivity becomes crucial.
In-Depth Analysis
Going beyond the basic features and requirements, understanding a software’s performance and usability can shed light on its overall effectiveness.
Performance and Usability
Performance hinges on how quickly a tool can process data and deliver insights. Effective analytical software maintains high speeds even when working with substantial datasets.
Usability is another important factor. Even the most powerful software is ineffective if users find it difficult to navigate. User-friendly interfaces with intuitive workflows can lead to better utilization of the tool.
Best Use Cases
Data analytics tools find application across numerous industries, enhancing decision-making processes:
- Healthcare: Analyze patient data to predict health trends or improve treatment pathways.
- Finance: Monitor market trends, manage risks, and identify opportunities through predictive analytics.
- Retail: Understand customer behavior to optimize inventory and enhance marketing strategies.
As organizations continuously aim for data-driven approaches, the demand for robust analytic tools will likely increase.
The right data analytics software can turn noise into signals, providing clarity and direction amid the chaos.
By being discerning about software selection, organizations can harness the true potential of data analytics. Ultimately, understanding which tools align with specific business needs will pave the way for future success.
Preamble to Data Analytics Applications
In the rapidly evolving landscape of technology and business, the role of data analytics applications has become increasingly pivotal. Companies across various sectors are adopting these tools to harness the power of data, transforming raw information into actionable insights. Understanding the nuances of data analytics applications is crucial for professionals in IT-related fields, as it directly impacts decision-making processes.
Data analytics applications are not just about tracking numbers; they are integral to developing strategies that enhance operational efficiency and drive growth. The importance of these applications lies in their ability to synthesize vast amounts of data quickly and accurately, allowing organizations to uncover hidden trends and patterns. This capability can be the difference between stagnation and thriving in today’s competitive market.
Definition and Significance of Data Analytics
Data analytics refers to the systematic computational analysis of data. It involves collecting data, cleaning it, and applying statistical methods to derive insights that inform decisions. The significance of data analytics cannot be overstated; it's like having a magnifying glass that reveals subtle details otherwise overlooked.
Tools like SQL, Tableau, and Google Analytics exemplify the diversity of applications available. Each tool serves unique purposes, from data visualization to complex predictive modeling. In short, data analytics is crucial for businesses seeking to thrive; it demystifies the information overload, making sense of the chaos.
Overview of Data Analytics Applications in Today's Market
Today’s market showcases a plethora of data analytics applications designed to cater to the varying needs of businesses. From small startups to multinational corporations, these tools have become indispensable. For instance, in retail, applications like Adobe Analytics aid in understanding customer behavior by analyzing purchase patterns. In the healthcare sector, data analytics software such as SAS allows for improved patient care by processing vast amounts of clinical data and identifying potential treatment outcomes.
"Innovative data analytics applications are reshaping business landscapes, enabling smarter decisions and more effective strategies."
The trend of real-time data analysis has gained traction, as businesses look for immediate insights to make quick decisions. Applications that boast real-time capabilities provide organizations with the agility needed to stay ahead. Moreover, as data privacy becomes a pressing concern, many tools now integrate robust security features, ensuring compliance with legal standards.
Types of Data Analytics Applications
Data analytics applications come in various forms, each uniquely tailored to meet specific business needs. Understanding these types is critical for anyone looking to harness the wealth of information available today. Each kind of data analytics plays its own distinct part, from shaping company strategy to enhancing customer experiences. As we navigate the intricate landscape of data analytics, it's vital to recognize how these applications can deliver actionable insights and drive decisions.
Descriptive Analytics Applications
Descriptive analytics provides a snapshot of historical data, answering the question, "What happened?" This form of analytics is crucial for any organization aiming to assess past performance and identify trends over time. By dissecting large sets of data, organizations can pinpoint successes and failures, shaping future strategies effectively.
Moreover, descriptive analytics can streamline operational processes by providing insights into key performance indicators. For example, retailers often explore sales data across different periods to discern seasonal buying patterns. This allows them to adjust inventory and marketing efforts accordingly.
- Use Cases:
- Analyzing customer measurements for targeted marketing.
- Compiling sales reports for internal reviews.
- Monitoring social media engagement to improve outreach.
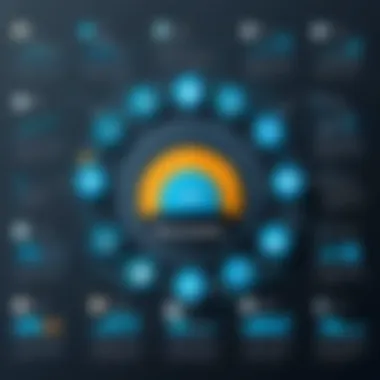
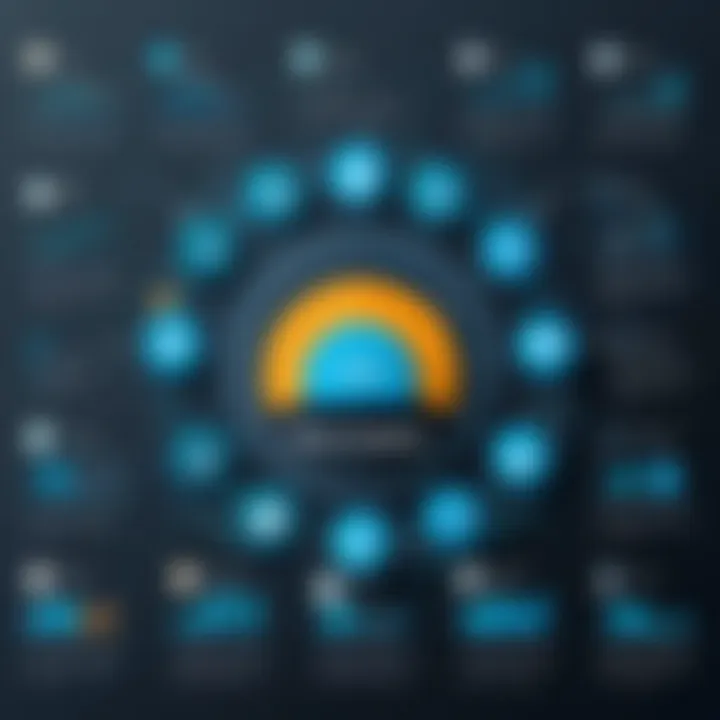
Descriptive analytics tools like Tableau or Google Analytics have become essential for businesses across sectors. They enhance transparency and provide a clear vision into varied performance metrics.
Predictive Analytics Applications
In a world that thrives on information, predictive analytics steps up, attempting to foretell the future based on historical data. By using statistical algorithms and machine learning techniques, organizations can predict potential outcomes and trends. This branch of analytics often raises eyebrows due to its impressive accuracy in forecasting outcomes based on previous data sets.
Businesses can utilize predictive analytics in numerous ways. For instance, financial institutions employ these applications to assess loan risk or determine fraudulent activities. Meanwhile, marketing teams can leverage predictions to enhance customer targeting, thus leading to improved conversion rates.
- Key Benefits:
- Anticipating customer needs, which leads to proactive service delivery.
- Enhancing product development through trend predictions.
- Managing inventory levels based on predicted demand.
As predictive analytics continues to evolve, tools such as IBM Watson and Microsoft Azure are making a significant impact, assisting analytics professionals in turning complex data into valuable foresight.
Prescriptive Analytics Applications
Unlike its descriptive and predictive counterparts, prescriptive analytics goes a step further by answering the question, "What should we do?" It combines historical data and predictive models with optimization and simulation algorithms. Essentially, prescriptive analytics offers guidance on optimal actions to take in diverse scenarios, enabling organizations to refine strategies and improve outcomes.
For example, in the supply chain industry, prescriptive analytics might help decide the best routes for delivery trucks, taking into account traffic patterns, delivery urgency, and operational costs. This analysis can save time and reduce expenses, directly influencing a company's bottom line.
- Applications:
- Workforce management to optimize employee scheduling.
- Marketing strategies that adjust based on consumer behavior in real-time.
- Financial forecasting to recommend best investment options.
Leading tools like SAS and Oracle's analytics suite offer robust platforms for prescriptive analytics, empowering businesses to not just react to data but actively shape their future based on insightful analysis.
With the right data analytics applications, organizations can navigate the unpredictable waters of modern business, harnessing the potential hidden within their data to seize opportunities and mitigate risks.
Key Features of Effective Data Analytics Applications
In the realm of data analytics, having the right tools at your disposal is crucial. Successful data analytics applications share several key features that enhance user experience and effectiveness. Understanding these elements becomes essential for anyone looking to leverage analytics for better decision-making. By focusing on usability, integration, real-time capabilities, and visualization, businesses can draw actionable insights from their data that drive strategy and resolve challenges.
User-Friendly Interface
A user-friendly interface is not just a luxury; it's a necessity in data analytics tools. If the application is too complex or unintuitive, users may find themselves frustrated, potentially disengaged from the analytics process altogether. This applies to both seasoned data scientists and non-technical stakeholders who need insights for strategic decisions.
An effective interface minimizes the learning curve and allows users to navigate seamlessly. Features such as drag-and-drop functionalities and intuitive dashboards help users explore data with ease. Additionally, customizable layouts empower users to tailor their environment, boosting productivity. In essence, a user-friendly interface fosters engagement and promotes a deeper understanding of data.
Integration Capabilities
When businesses utilize multiple databases and various software applications, integration becomes paramount. Data analytics platforms with strong integration capabilities allow for a smoother data flow from different sources.
Seamless integration means users can pull data from their existing systems, whether they are CRM software like Salesforce, databases like MySQL, or cloud services like Google Drive. This interoperability leads to a more comprehensive view of performance metrics. It is also beneficial for organizations looking to maintain their current tech stack while still moving toward advanced analytics capabilities.
- Eases data collection from various sources
- Reduces errors that occur during manual data handling
- Enhances collaboration by combining insights from various departments
Real-Time Analysis
In today’s fast-paced environment, waiting hours or days for data interpretation can result in lost opportunities. Real-time analysis enables businesses to make swift decisions based on the most current data available, addressing issues promptly and capitalizing on emerging trends.
Using streaming analytics or in-memory data processing, applications can continuously monitor input data and generate insights without delay. This capability is particularly beneficial for industries like finance and healthcare, where timely information can impact decision-making. Having access to real-time data leads to:
- Immediate response to customer needs
- Enhanced operational efficiencies
- Increased ability to adapt to changing market conditions
Data Visualization Tools
Data in raw form can be overwhelming and hard to interpret, but visualization tools bring clarity. The importance of intuitive visual representation cannot be overstated. Good visualization tools not only present data but also tell a story, guiding users to understand complex concepts at a glance.
From charts and graphs to heat maps and dashboards, effective data visualization allows users to uncover patterns, identify trends, and make comparisons easily. A well-designed visualization can drive engagement, encouraging stakeholders to dive deeper into the data story being told. Furthermore, by enabling interactive visualizations, users can manipulate data views to answer specific questions without requiring technical skills.
As a result, organizations are often able to:
- Communicate insights powerfully across teams
- Foster a data-driven culture by making insights accessible
- Assist in better decision-making by reducing information overload
"IIllustrative visualizations can be the beacon that guides users through the vast sea of raw data."
Industry Applications of Data Analytics Software
Data analytics software serves a vital role across various industries, helping organizations make informed decisions through insights derived from data. In today’s data-rich environment, the application of analytics has shifted from being a luxury to a necessity. With the right tools and techniques in place, businesses can analyze trends, understand customer behaviors, and optimize operations. Each industry implements data analytics uniquely, depending on its specific needs, challenges, and goals.
Healthcare Industry
In healthcare, data analytics applications become a linchpin for improvement in patient outcomes and operational efficiency. The industry uses these tools to analyze patient data, optimize treatment plans, and reduce costs. For instance, predictive analytics can anticipate patient readmission rates, allowing hospitals to tailor discharge plans effectively. Furthermore, the integration of electronic health records (EHR) with analytical tools helps in tracking treatment pathways and identifying best practices.
The trend of using real-time data also enhances clinical decision-making. For example, machine learning algorithms can analyze a patient’s vital signs during a procedure and alert medical staff to potential complications. By leveraging data visualization, stakeholders can easily interpret complex data sets, leading to quicker, more informed choices. The advent of telemedicine platforms further underscores analytics' importance by ensuring that healthcare providers can make data-driven decisions remotely.
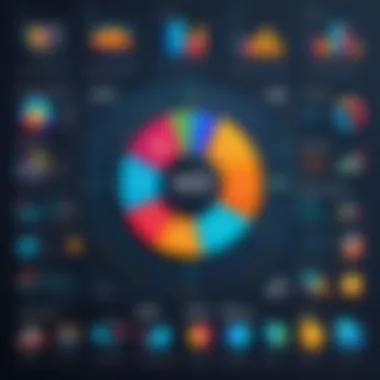
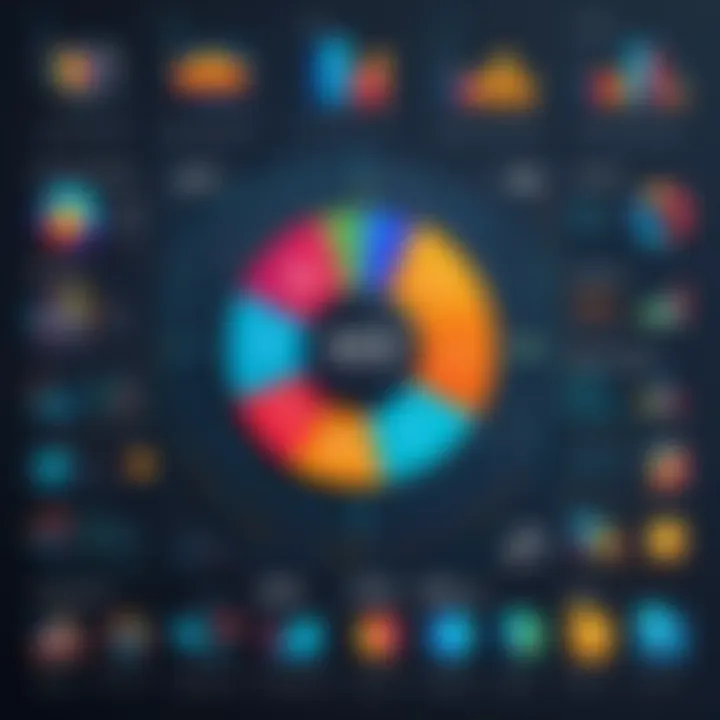
Retail Industry
The retail space thrives on the insights provided by data analytics applications. Retailers harness them for inventory management, customer segmentation, and personalized marketing strategies. Using historical sales data, companies can forecast demand accurately, preventing stockouts or overstock situations. This optimization not only saves costs but enhances customer satisfaction.
Customer behavior analysis is another critical application. Retailers analyze purchase patterns and preferences to tailor recommendations, thereby increasing conversion rates. For instance, loyalty programs gather valuable customer data that can be processed to create targeted promotions, ensuring that shoppers receive offers that resonate with their habits.
Furthermore, data visualization tools allow retailers to monitor sales performance across different regions and demographics, revealing where adjustments are necessary. This constant feedback loop is essential for staying competitive in a market that's ever-evolving.
Financial Services
In financial services, the stakes are high, and so are the benefits derived from data analytics. Risk assessment is an area where these applications shine, helping institutions detect fraud and manage risk effectively. Financial organizations utilize predictive models to assess the likelihood of default on loans, enabling better decision-making on lending operations.
Moreover, investment firms apply analytics to analyze market trends, identify investment opportunities, and enhance portfolio management. By employing sophisticated algorithms, they assess vast amounts of market data in real-time, giving them a distinct advantage over competitors.
Compliance with regulations is another critical concern. Data analytics applications facilitate tracking transactions and customer behaviors to ensure adherence to legal requirements. The necessary adjustments can be made swiftly, reducing exposure to penalties or reputational damage.
Manufacturing Sector
Manufacturing has seen significant transformations through the application of data analytics. By deploying Internet of Things (IoT) devices, manufacturers capture real-time data throughout the production process. This data enables them to monitor equipment efficiency, reduce downtime, and identify maintenance needs before equipment failures occur.
Predictive analytics in quality control helps identify defects early in the manufacturing process. By analyzing historical data, manufacturers can refine processes and reduce waste, contributing to sustainable practices. Furthermore, supply chain analytics ensure that materials are available when needed, enhancing productivity.
Data visualization tools further enrich this landscape by presenting complex data in an understandable format. Decision-makers can grasp the health of the production line at a glance, making timely adjustments that drive efficiency and profitability.
"The true power of data analytics in industry lies in its ability to transform raw data into actionable insights."
To sum up, data analytics applications deeply impact healthcare, retail, financial services, and manufacturing sectors. They not only help in understanding past performance but also shape future strategies. Using the right tools allows businesses to traverse their respective landscapes confidently, ultimately leading to sustained growth and success.
For further information, check out Wikipedia, Britannica or join discussions on platforms like Reddit.
By acknowledging the specialized applications of data analytics across these industries, organizations position themselves to leverage data for better outcomes, client satisfaction, and operational efficiency.
Evaluating Data Analytics Tools
In the rapidly evolving landscape of data analytics, evaluating tools effectively is crucial. The right data analytics tool can significantly improve an organization's ability to derive insights, make informed decisions, and enhance overall operational efficiency. It goes beyond just picking a popular name from the market. Decisions made here affect everything from data quality to the user experience. Understanding which criteria are essential when selecting a tool can be the difference between success and mediocrity.
Criteria for Selection
When evaluating data analytics tools, several critical factors come into play:
- Functionality: What capabilities does the tool offer? It should be equipped to perform various analyses, from basic reporting to complex modeling. This flexibility ensures that as your business grows, your tool can adapt to new demands.
- User Experience: A tool needs a user-friendly interface. If the learning curve is too steep, it could discourage team members from engagement. Tools that prioritize intuitive design are likely to yield better adoption rates.
- Integration Capability: Can the tool seamlessly integrate with your existing systems? Consideration of how well it meshes with other software could save time and reduce inconsistencies in data reporting.
- Scalability: As datasets grow and needs change, you need a solution that can scale effectively. Not every tool is built to handle large, complex datasets effectively.
- Support and Training: Having a reliable support system is paramount. A tool may have fantastic features, but without adequate support or training resources, users may feel lost.
- Security Features: In an age where data breaches are all too common, don’t overlook robust security features. Assess how the tool handles data protection and compliance with regulations.
By thoroughly evaluating these criteria, organizations ensure a strategic selection of tools that will serve their long-term analytics needs.
Pricing Models and ROI Considerations
Understanding the pricing structure of data analytics tools is essential for making a well-informed decision. Pricing can vary significantly, and what seems like a bargain might end up costing more in terms of lost productivity or ineffective insights. Here are key considerations:
- Upfront Costs: Some tools may have a hefty initial cost, while others may adopt a subscription-based model. Evaluating which aligns best with your budgeting capabilities is essential.
- Ongoing Fees: Apart from the initial outlay, consider any hidden ongoing fees. Subscription fees and potential costs for extra features or user licenses can add up.
- Cost per User: For teams with fluctuating sizes, a pay-per-user model might make more sense. This provides flexibility without committing to a high-cost tool you won't fully utilize.
- Expected Return on Investment: When investing in analytics tools, consider how they will pay for themselves. Projects using advanced analytics often yield improved operational efficiency, leading to substantial savings over time.
- Long-Term Viability: Assess the long-term benefits of the tool. A more expensive tool that offers superior insights can lead to better business decisions down the line.
In summary, evaluating data analytics tools should involve a nuanced approach, weighing cost against potential value. Transparency in pricing, along with a clear understanding of the expected ROI, is critical for making investments that foster growth and efficiency.
"Choosing the right tools is not just about the numbers; it’s about empowering your team to make data-driven decisions that lead to better outcomes."
By combining a structured evaluation process with careful consideration of pricing models and the anticipated benefits, organizations can enhance their data analytics capabilities and stay ahead in a data-driven world.
Common Challenges in Data Analytics Adoption
Adopting data analytics applications comes with its fair share of hurdles. Organizations on this journey encounter numerous challenges that can hinder their success in fully leveraging data insights for informed decision-making. Understanding these obstacles is crucial as it not only guides organizations in making informed choices but also helps in strategizing for smoother implementation processes.
Data Quality Issues
Data quality is the bedrock upon which effective analytics is built. Poor data quality leads to erroneous analyses, which result in misguided decisions. Imagine relying on a dataset where half the entries are corrupted or inaccurate; the outcome could be catastrophic. For instance, a retail company launching a targeted marketing campaign based on flawed customer data might waste significant resources and miss the mark with their audience. The stakes are high, especially when the decision-makers rely heavily on the information derived from this data for strategic directions.
Factors Affecting Data Quality:
- Inconsistency: When different departments use varied formats or definitions for data, it can create havoc during analysis.
- Incomplete Data: Missing entries can skew results, making it hard to draw accurate conclusions.
- Outdated Information: Even valid data can lose its relevance over time, leading to misguided interpretations.
Improving data quality requires investments in thorough data management practices. Regular audits, cleaning processes, and robust data entry protocols are necessary. Without addressing these aspects, organizations risk operating with one hand tied behind their back, trying to make sense of data that is, frankly, unreliable.
Resistance to Change within Organizations
Despite the proven advantages of data analytics, resistance to change often rears its head in organizations. Employees may feel threatened by new tools, fearing that their roles could become obsolete or that the learning curve might be steep. For example, amid the rollout of advanced analytics software, a marketing team might prefer sticking to traditional methods of reporting and insights, viewing the new system as a cumbersome obstacle rather than a beneficial upgrade.
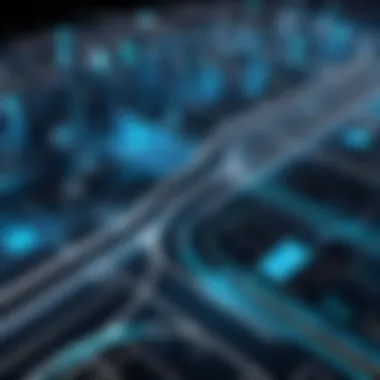
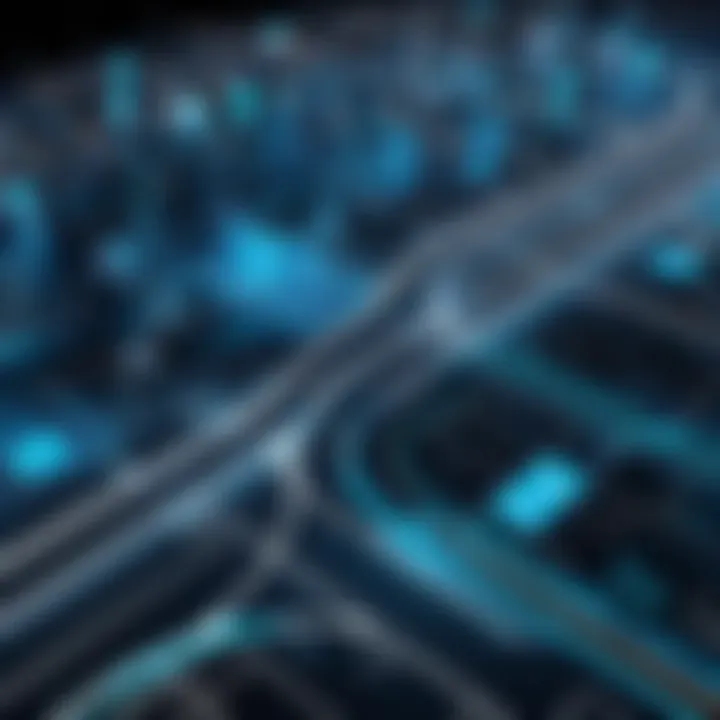
Influencing Factors:
- Lack of Training: Employees may feel overwhelmed if they haven't been properly trained to use new tools and techniques.
- Cultural Barriers: An organizational culture that is averse to innovation can stifle the adoption of analytics solutions.
- Fear of Job Displacement: Employees might view automation and advanced analytics as threats rather than opportunities for enhancement or job growth.
It is essential for leadership to foster an environment where change is embraced. Strategies such as engaging employees during the selection and implementation phases and providing adequate training can smooth the transition.
Effective communication about the benefits of data analytics and how they align with everyone’s goals can also alleviate fears. As a quote famously states, "Change is the only constant in life; to resist change is to resist growth."
In summary, these common challenges illuminate critical considerations for organizations venturing into the realm of data analytics adoption. By addressing issues related to data quality and resistance to change, companies can pave their pathway to successful data-driven decision-making.
Emerging Trends in Data Analytics Applications
The arena of data analytics is always shifting and evolving. As technology innovates, fresh methodologies and tools are emerging that redefine how organizations utilize data. Understanding these emerging trends is set to enable businesses to remain competitive, agile, and informed amidst a continuously changing landscape. Consequently, keeping a finger on the pulse of these trends is invaluable for professionals seeking to harness the advantages of data analytics.
Artificial Intelligence and Machine Learning Integration
In the past few years, integrating artificial intelligence (AI) and machine learning (ML) into data analytics has become the talk of the town. At its core, this amalgamation allows for smarter data analysis, offering insights that human analysts might overlook.
With machine learning algorithms, systems can analyze patterns in vast amounts of data and refine themselves over time, leading to more accurate predictions. This means businesses can forecast trends or customer behaviors more effectively, allowing for better decision-making. AI acts as an agile partner, interpreting data at lightning speed compared to traditional analyses. The benefits are manifold:
- Improved Decision-Making: Organizations can rely on data-driven decisions, reducing reliance on intuition.
- Cost Efficiency: Automated analytics can cut down on labor costs by doing the heavy lifting.
- Personalization: Better insights help businesses tailor their offerings to meet client needs precisely.
However, adopting these technologies comes with its own set of challenges. Organizations must address the steep learning curve and ensure they have the right data infrastructure in place. There is also the question of maintaining data integrity and oversight.
"AI and ML transform raw data into actionable insights, turning the ordinary into the extraordinary."
Data Privacy and Ethics Concerns
As reliance on data grows, so does the necessity for strict adherence to data privacy and ethical considerations. Organizations are now confronted with the pressing need to not just comply with regulatory requirements, such as the General Data Protection Regulation (GDPR), but to genuinely commit to ethical data use.
Thoughtful management of data analytics can build trust with clients. However, mishandling data or utilizing it in ways that breach privacy can lead to reputational damage, legal consequences, and a host of other issues. Here are a few paramount considerations:
- Transparency: Organizations should be clear about how they collect and use data.
- Consent: Prioritizing user consent is crucial. Users should have the right to know what their data is being used for.
- Data Minimization: Collect only what is necessary for analytics to limit exposure and risk.
In an ecosystem where data is a vital asset, balancing analytics with ethical considerations is crucial for sustainable development. Businesses that prioritize responsible data use position themselves as leaders and innovators, earning the trust of their clientele.
Navigating the delicate waters of emerging trends requires a commitment to both technology and integrity. As AI and ML continue to weave their way into data analytics, and as the focus on privacy and ethics intensifies, organizations must be both innovative and responsible.
Future Directions of Data Analytics Applications
The landscape of data analytics is continuously morphing, responding to technological advancements, changing market needs, and evolving user expectations. Understanding the future directions of data analytics applications becomes crucial for practitioners and organizations alike. It is essential not only to keep ahead of the curve but also to harness upcoming trends effectively to enhance decision-making and drive innovation.
Evolution of Analytics Tools
The evolution of analytics tools is a fascinating narrative of technology catching up with human ingenuity. In earlier days, analytics applications were largely rudimentary, serving mainly to sort and filter data. Fast forward to today, and we see sophisticated platforms that leverage AI and machine learning, allowing businesses to look beyond historical data to forecast future trends.
Each generation of tools brings new features and capabilities. For instance, tools like Tableau and Power BI have set the standard for visually representing data, making it accessible for non-technical users. More recent entrants, such as Google Data Studio, have capitalized on cloud capabilities to enable real-time collaboration. These advancements make analytics not just an IT function but a role accessible to various departments within organizations.
It’s worth noting that the adaptability of these tools is critical. As data continues to multiply exponentially, the tools must evolve in sophistication. This evolution involves integration capabilities with emerging technologies. Flexibility allows organizations to incorporate various data sources, thus crafting a more comprehensive view of the business landscape.
The Role of Data Governance
A robust data governance framework stands as a pillar for successful data analytics applications. As analytics tools evolve, the volume and complexity of data continue to grow. This necessitates a structured approach to handling data ensuring that it stays reliable, secure, and compliant with regulations.
Good data governance ensures that there are clear policies and standards in place for managing data quality. This leads to better decision-making as the data utilized is credible. Furthermore, it builds trust among users, as they can rest easy knowing that the data they are working with is validated and well-managed.
Instituting effective governance practices means setting up a collaborative effort across departments. This often involves creating data stewardship roles that are responsible for maintaining data quality and compliance.
With advances in data privacy laws, like GDPR, organizations that neglect data governance not only risk their credibility but may also face legal repercussions. By prioritizing governance, organizations can build analytics applications that are not just powerful but also secure and aligned with prevailing standards.
Proper data governance is not just a hedge against risks; it is an enabler of quality insights and robust decision-making.
Culmination
In an era dominated by data, the importance of effective data analytics applications cannot be overstated. These tools are not merely accessories to business strategy; they serve as vital components that drive informed decision-making across various industries. As explored in previous sections, data analytics applications encompass a broad spectrum of functionalities—from descriptive insights that illuminate past performance to predictive modeling that forecasts future trends. Each type contributes uniquely to an organization's ability to leverage data.
Summation of Key Insights
To sum it all up, a few key insights emerge:
- Key Functionalities: The variety of analytics tools ranges from those focusing on real-time data visualization to those employing advanced predictive analytics. Each tool serves a particular purpose.
- Industry Relevance: As demonstrated, applications in healthcare, finance, and retail reveal specific case studies where data analytics tools have resulted in significant advancements. For example, hospitals utilizing predictive analytics to readmission rates has shown promising outcomes.
- Emerging Trends: The ongoing integration of AI and machine learning indicates a clearer trajectory for future advancements in analytics tools. This evolution is essential, as consumers and businesses alike continue to demand rapid insights in an increasingly digitized world.
"The future isn’t just happening; it’s being crafted by those who understand their data."
Mitigating challenges associated with data quality and resistance to change remains crucial in ushering in new analytics tools successfully. The delicate balance between leveraging technology and ensuring data privacy further complicates these developments, but it's a challenge worth tackling.
Final Thoughts on the Importance of Data Analytics Applications
Looking ahead, the increasing emphasis on data-driven strategies will only heighten the significance of data analytics applications. Organizations willing to adapt and incorporate these tools into their decision-making routines stand to benefit tremendously. They gain the capacity not just to react, but to anticipate, thereby enhancing competitiveness in their sectors.
Moreover, an understanding of data governance will only deepen the value derived from analytics. As data becomes a cornerstone of strategic development, effective governance ensures that organizations not only comply with regulations but also foster a culture of accountability and ethical data usage.