Explore the Best Data Forecasting Tools for Success
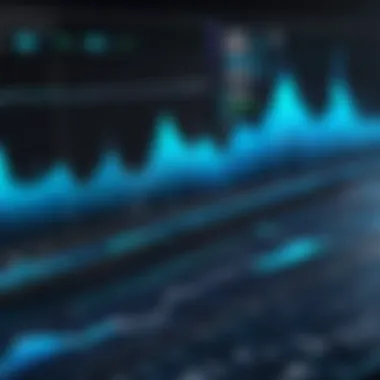
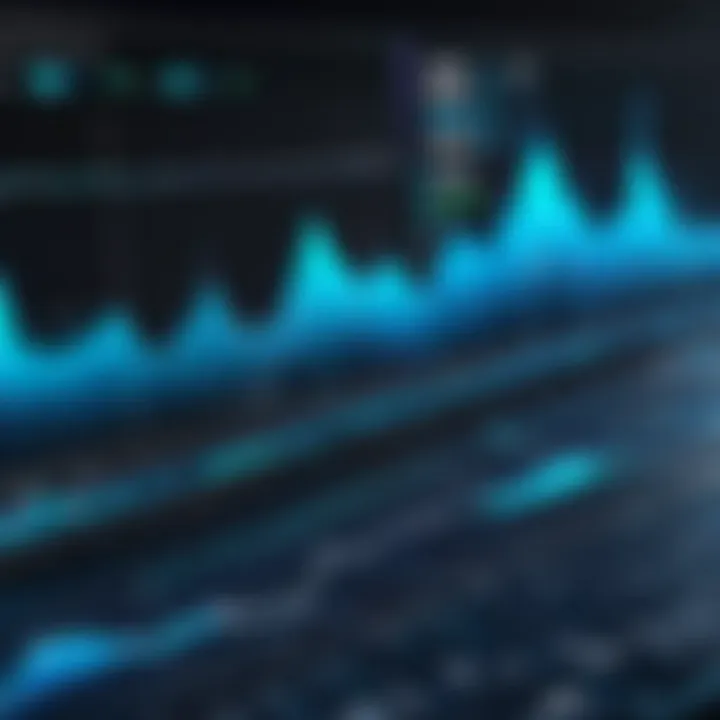
Intro
Data forecasting tools have become essential for various industries. They assist in predicting future trends based on historical data. The need for accurate forecasting has only increased with the rise of big data analytics. This guide will explore different aspects of data forecasting tools. We will cover key features, system requirements, and best use cases, among other topics. Choosing the right tool can greatly impact decision-making within an organization.
Several methods are used in data forecasting. These include time series analysis, regression methods, and machine learning techniques. Each method has its strengths and weaknesses, which can influence the effectiveness of predictions. Understanding these methodologies will help you better select a tool that fits your needs.
The landscape is filled with a variety of tools, each offering unique functionalities. Users must consider factors like ease of use, integration capabilities, and scalability when selecting a forecasting tool. This guide aims to provide detailed insights that will enable informed decision-making.
Ultimately, the goal is to empower users with the necessary knowledge to navigate the complex world of data forecasting tools. By the end of this guide, you’ll have a clearer understanding of what to look for and how to implement these tools effectively.
Foreword to Data Forecasting
Data forecasting is a critical element within the field of data science and analytics. It serves to predict future trends based on historical data. As industries increasingly rely on data-driven decision making, the importance of accurately forecasting outcomes has grown significantly. With a better understanding of what to expect in the future, organizations can strategically allocate resources, optimize operations, and improve financial performance.
In this guide, we aim to present a comprehensive view of data forecasting tools. We will discuss various types of forecasting methods, highlight key tools available in the market, and provide guidance on how to select the most suitable forecasting tool for particular needs. Understanding the various facets of data forecasting is necessary for professionals in IT-related fields, software developers, and students who aspire to work in domain.
Definition of Data Forecasting
Data forecasting is defined as the process of using historical data to make predictions about future events. This can include anything from sales predictions to stock market trends. Various mathematical models and statistical techniques are utilized to create forecasts. The reliability of the predictions often depends on the quality of the historical data and the methods used to analyze it.
Common methods of data forecasting include time series analysis, machine learning algorithms, and econometric modeling. Time series analysis focuses on trends over time, while machine learning can adapt and learn from new data to improve predictions continually.
Importance of Forecasting in Decision Making
Forecasting serves as the backbone for informed decision making. Businesses that leverage forecasting techniques have a competitive edge. For example, accurate sales forecasts enable companies to manage inventory effectively, reducing the cost associated with excess stock or missed sales opportunities.
The ability to anticipate future trends can also impact budgeting and financial planning. For startups, understanding forecasted cash flow can determine their viability and inform funding requests. Conversely, failing to forecast adequately can result in missed opportunities or misguided strategies.
"Good forecasting benefits organizations by enabling them to make proactive decisions rather than reactive measures."
Ultimately, the importance of forecasting extends to numerous sectors, including finance, healthcare, and technology. At its core, forecasting is about risk management. Organizations that grasp this will likely be more successful in navigating uncertain futures.
Types of Data Forecasting Methods
Data forecasting is a vital practice for businesses and organizations, allowing them to prepare for future changes, trends, and challenges. The methods used for forecasting can be categorized broadly into two types: qualitative and quantitative forecasting methods. Understanding these methods helps professionals choose the appropriate approach based on their specific needs. Each method has distinct advantages and considerations that can significantly impact the quality and reliability of forecasts.
Qualitative Forecasting Methods
Qualitative forecasting methods rely on subjective judgment and intuition rather than numerical data. This approach is particularly useful when historical data is scarce or when the future is highly uncertain. These methods often involve gathering insights from experts or stakeholders to form predictions.
Several common techniques in qualitative forecasting include:
- Expert opinion: Consulting individuals with specialized knowledge can provide valuable insights.
- Focus groups: Engaging with a group of individuals to discuss trends can yield diverse perspectives.
- Delphi method: This technique utilizes rounds of anonymous opinions from experts, allowing for consensus on predictions.
The main benefit of qualitative methods is their adaptability to new trends or rapid changes that quantitative methods might miss. However, they can also be influenced by bias, making it crucial to use multiple perspectives to validate forecasts.
Quantitative Forecasting Methods
Quantitative forecasting methods use mathematical models and historical data to make predictions. These methods are grounded in numerical analysis and statistical techniques, allowing for more objective assessments. It is especially effective when data sets are abundant and reliable.
Key quantitative methods include:
- Time series analysis: This technique analyzes historical data over time to identify trends and seasonal patterns.
- Regression analysis: This method examines relationships between different variables to predict outcomes.
- Machine learning algorithms: Modern techniques utilize algorithms to derive insights from large datasets, often yielding more accurate forecasts.
One significant advantage of quantitative methods is their scalability. They can process large amounts of data quickly. However, these depend heavily on the quality of historical data, and if the data is flawed, forecasts can be greatly impacted.
Forecasting methods can be applied based on the context and needs of the business, ensuring a tailored approach to decision-making.
Choosing between qualitative and quantitative forecasting methods often depends on the specific situation and the availability of data. Both methods have their merits and can even be combined for a more comprehensive approach to forecasting.
Overview of Key Data Forecasting Tools
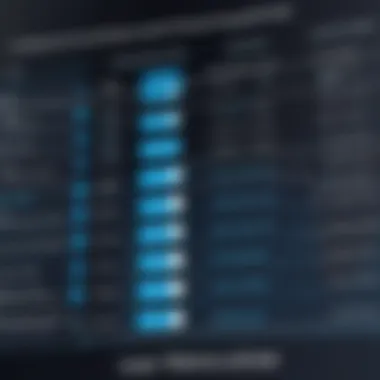
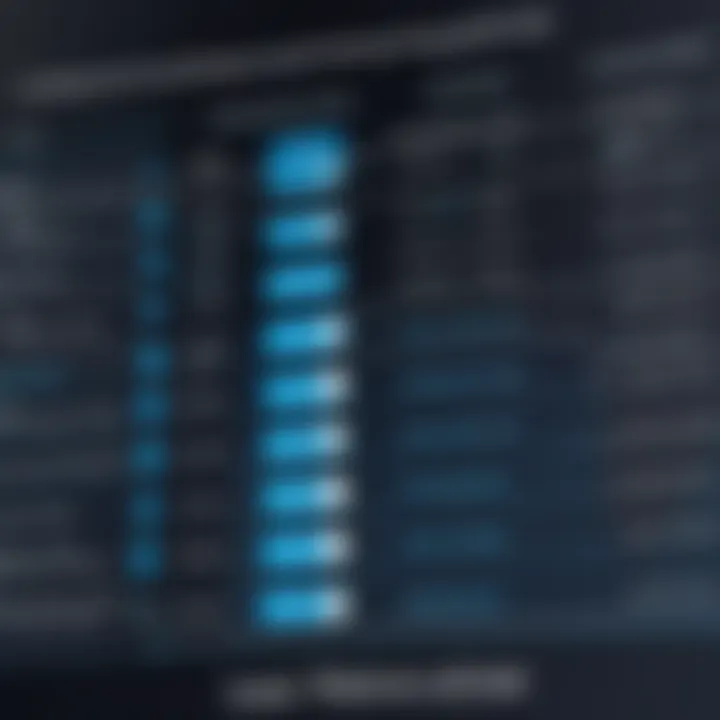
In the realm of data forecasting, the tools employed are crucial for transforming raw data into actionable insights. These tools facilitate a range of forecasting techniques that enable organizations to anticipate future events and trends based on historical data. Understanding the landscape of data forecasting tools is vital, as it empowers users to make informed decisions that can shape the direction of a business or project.
Selecting an appropriate forecasting tool can be a complex decision as it involves evaluating various elements like functionality, ease of use, integration capabilities, and cost. Each tool comes with its own set of advantages, catering to different user needs and contexts. This section will delve into popular tools in the market, offering insights into their functionalities and how they compare against one another.
Popular Tools in the Market
The data forecasting landscape features several tools that stand out due to their effectiveness and reliability. Some of the most recognized tools include:
- Tableau: Known for its robust visualization capabilities, Tableau allows users to analyze data through interactive dashboards and offer predictive analytics features.
- Microsoft Excel: A staple in many industries, Excel provides basic forecasting functionalities through formulas and built-in regression analysis tools.
- SAP Analytics Cloud: This tool combines business intelligence and planning capabilities, providing users the ability to perform real-time forecasting and scenario modeling.
- IBM Planning Analytics: Leveraging IBM's cloud technologies, this tool supports advanced data modeling techniques and provides users with predictive analytics capabilities.
- Forecast Pro: Specializing in time series forecasting, Forecast Pro offers a range of models and supports automatic selection of the best forecasting method for users.
Each of these tools caters to different levels of expertise, from beginner to advanced users. The choice of tool often reflects the specific requirements of the forecasting task at hand.
Comparison of Leading Tools
When comparing forecasting tools, various factors come into play. These factors help in understanding the strengths and weaknesses of each tool. Here’s a brief comparison based on key considerations:
- Functionality: Some tools provide comprehensive features, while others focus on specific tasks. Tableau and SAP Analytics Cloud are highly functional for advanced analytics requirements, whereas Microsoft Excel is suitable for basic forecasting needs.
- Ease of Use: User experience varies significantly among tools. Tools like Excel are generally user-friendly, making them accessible for non-technical users. In contrast, specialized tools like IBM Planning Analytics may require a steeper learning curve.
- Integration Capabilities: The ability to work seamlessly with other systems is essential. SAP Analytics Cloud and IBM Planning Analytics excel in this area, integrating well with various enterprise applications.
- Cost: Pricing models also differ markedly. While some tools offer free versions, others operate on subscription or license models, impacting long-term cost effectiveness.
In summation, the choice of a data forecasting tool should align with specific organizational needs, from basic analytics to advanced predictive modeling. Assessing each tool against these criteria will aid in making a more informed decision, ultimately optimizing forecasting efforts.
Criteria for Selecting a Data Forecasting Tool
Selecting the right data forecasting tool is critical for achieving accurate projections and making informed decisions. Poor tool selection can lead to flawed forecasts, wasted resources, and missed opportunities.
Several criteria should be considered when evaluating data forecasting tools. These include functionality, user interface, software integration, and cost structures.
Functionality and Features
Functionality refers to the specific capabilities and features of a forecasting tool. A suitable tool should support the methods and models important for your forecasting needs.
Key features to look for include:
- Data Input Flexibility: The ability to import data from various sources is crucial. A good tool should accept data from spreadsheets, databases, and even APIs.
- Modeling Techniques: Different forecasting situations require different models. Tools that offer a range of methods, both qualitative and quantitative, provide flexibility in approach.
- Analytics Capabilities: The addition of advanced analytics can help in deriving insights. Choose tools that provide data visualization and analysis features.
The selection of features can influence forecasts’ accuracy and relevance to your specific business context.
Ease of Use and User Interface
User friendliness is vital in ensuring that all team members can utilize the tool effectively. A well-designed user interface reduces the learning curve and encourages adoption across your organization.
Considerations for this criterion include:
- Intuitive Navigation: Users should navigate the tool easily, without requiring extensive training.
- Accessibility of Features: Important features should be easily accessible. If users struggle to find what they need, it can hinder productivity.
- Customizable Views: The capability to customize the dashboard or interface according to user preferences can enhance usability.
A focus on ease of use ensures efficiency in generating forecasts and utilizing the available data effectively.
Integration with Other Software
Integration capabilities can significantly expand the functionality of a forecasting tool. The ability to connect seamlessly with existing software applications allows for a more comprehensive data utilization strategy.
Key aspects include:
- APIs for Connectivity: A tool that offers APIs enables users to integrate it with CRM, ERP, or other business management systems.
- Data Synchronization: Ensure the tool can synchronize data in real-time to reflect accurate forecasting.
- Collaboration Features: Tools that facilitate collaboration among team members enable better sharing of forecasts and insights.
Effective integration contributes to a smoother workflow and enhances the overall data analysis process.
Cost and Licensing Options
When puchasing a forecasting tool, understanding the pricing structure is essential. Costs can vary widely depending on features, licensing models, and support options.
Consider these factors:
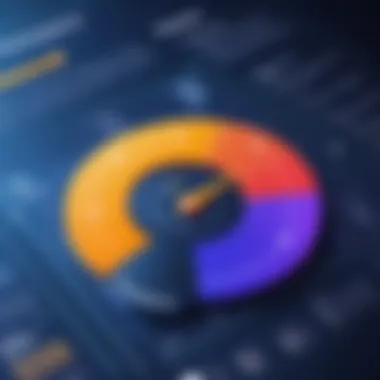
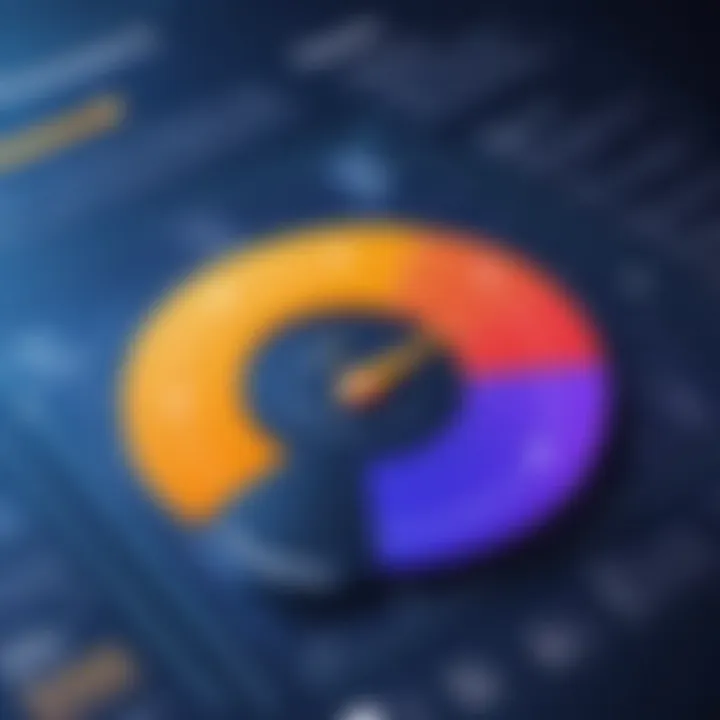
- License Types: Determine whether the software offers subscriptions, perpetual licenses, or pay-per-use models.
- Total Cost of Ownership: Factor in not only the initial purchase price but also ongoing costs like maintenance, upgrades, and support.
- Free Trials: Opting for tools that offer free trials can provide insights into usability and value before making a commitment.
Evaluating costs ensures that you acquire a forecasting tool that meets your budget while delivering the features and support needed.
Implementing Data Forecasting Tools
Implementing data forecasting tools is a critical phase in leveraging the power of predictive analytics. The success of these tools does not stem merely from choosing the right software; instead, it involves a comprehensive approach that includes various aspects such as setup, configuration, user training, and ongoing support. Understanding these elements can significantly enhance the effectiveness and reliability of the forecasts generated.
Setting Up the Tool
The setup process is often overlooked but is essential for achieving accurate and actionable insights. Proper installation and configuration lay the foundation for the tool’s subsequent performance. It is imperative to evaluate the specific prerequisites of the data forecasting tool you are using; this includes necessary data inputs, integration capabilities with existing systems, and accessibility of the user interface.
A structured setup process generally involves the following steps:
- Installation of Software: Follow the guidelines provided by the vendor. Ensure that your hardware can support this tool’s requirements.
- Data Input Configuration: Prepare your data sources. Whether it's collecting data from databases or importing spreadsheets, accuracy here is crucial.
- Integration with Existing Systems: Connect the forecasting tool with databases or analytics platforms. This step can improve data flow and facilitate a seamless analytical workflow.
- User Access Setup: Determine who in your organization will utilize the tool and their respective access levels. Proper management of user settings can prevent data leaks and maintain project integrity.
Training and Support Resources
User training and support resources are equally critical in ensuring that your team can effectively utilize the forecasting tool. Even the most powerful tools can become ineffective without proper usage. Comprehensive training programs can facilitate a deeper understanding of the tool’s functionalities, ensuring users harness the full potential of the software.
Here are key aspects to consider for training and support:
- Initial Training Sessions: Schedule hands-on training sessions that allow users to engage directly with the tool. Practical experience can alleviate many common issues users encounter.
- Resource Materials: Provide access to user manuals, video tutorials, and online courses. These resources empower users to troubleshoot independently and can boost their confidence.
- Dedicated Support Team: Identify a support team or contacts for immediate assistance. Having this resource available can reduce downtime during the implementation phase.
- Feedback Mechanism: Establish a system for collecting user feedback. Continuous improvements based on real user experiences can enhance tool utilization and satisfaction.
Implementing data forecasting tools with diligence will support your business in making decisions based on solid predictions. As you embark on this journey, remember that the effort invested in setup and training yields substantial returns in data accuracy and forecasting capabilities.
Common Challenges in Data Forecasting
Data forecasting presents a range of challenges that can significantly affect the reliability of outcomes and the decision-making processes in various industries. Understanding these challenges is essential for those involved in data analysis, planning, and implementation of forecasting tools. Addressing these challenges not only improves the accuracy of predictions but also enhances the overall effectiveness of a business strategy. This section delves into the common challenges in data forecasting, highlighting key elements and considerations that professionals should be aware of.
Data Quality Issues
One of the foremost challenges in data forecasting is maintaining data quality. Poor data quality can arise from various factors, including incomplete data, inaccurate data entry, and outdated information. This results in unreliable forecasts that can mislead stakeholders and result in poor decision-making.
To mitigate data quality issues, it is crucial to implement processes for rigorous data validation and cleaning. This can involve:
- Regular audits: Periodically review data to identify inconsistencies and errors.
- Standardized formats: Use consistent formats for data collection to minimize variations that can lead to errors.
- Training staff: Ensure that all personnel involved in data entry understand the importance of accuracy and completeness.
Investing in quality assurance measures contributes to more reliable forecasting models and ultimately supports better business outcomes.
Overfitting and Underfitting Models
Another major challenge lies in model selection—specifically, the issues of overfitting and underfitting. Both concepts represent potential pitfalls in creating predictive models, affecting their effectiveness.
Overfitting occurs when a model learns not only the underlying pattern of the training data but also the noise. This results in a model that performs extraordinarily well on training data but poorly on unseen data. Overfitting can be addressed through:
- Regularization techniques: These methods add a penalty to the loss function, discouraging the model from fitting the noise.
- Cross-validation: This technique helps ensure that the model generalizes well to new data by evaluating performance on different subsets of data.
On the contrary, underfitting happens when a model is too simple to capture the underlying trend of the data. This can lead to low accuracy in both training and test datasets. To combat underfitting, consider:
- Feature engineering: Adding relevant features can provide additional information for the model.
- Complex models: Use more sophisticated algorithms that can capture intricate patterns in the data.
The balance between overfitting and underfitting is critical for building effective forecasting models. Striking the right balance will enhance predictive performance and improve decision-making processes.
"Effective forecasting is a blend of art and science, requiring attention to data quality and model appropriateness."
Understanding these challenges equips IT professionals, software developers, and students in related fields with the insight necessary for developing accurate and reliable forecasting strategies. By addressing data quality issues and model fitting challenges, organizations can vastly improve their forecasting capabilities, leading to more informed decisions.
Case Studies of Successful Data Forecasting
Examining case studies provides a tangible view into how data forecasting tools apply in real-world scenarios. These examples illustrate the varied applications of forecasting in industries such as retail, finance, healthcare, and technology. The analysis of these cases helps readers understand not only the functional aspects of tools but also the intricate dynamics of decision-making and strategy formulation.
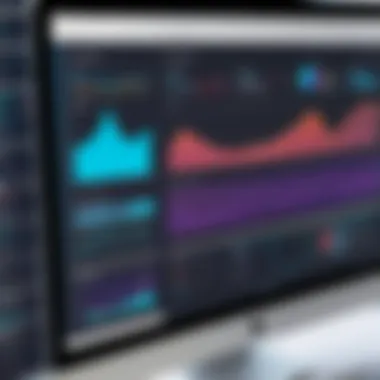
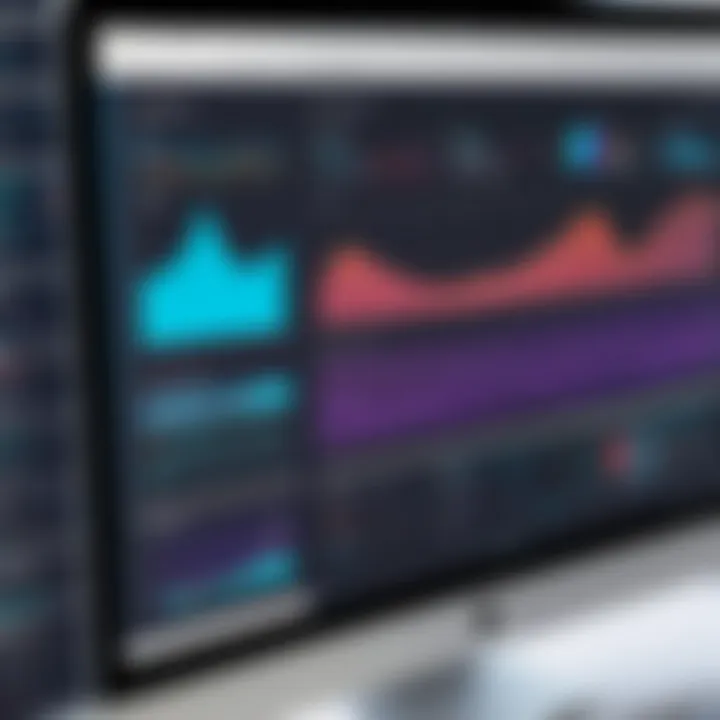
Industry Examples
1. Retail Sector
Target recently utilized an advanced forecasting tool to optimize its inventory management. By analyzing historical sales data along with seasonal trends, Target improved its stock levels. The impact was substantial, resulting in a 25% reduction in excess inventory while increasing product availability. The case emphasizes how retail businesses can harness data forecasting to enhance efficiency and customer satisfaction.
2. Financial Services
Goldman Sachs implemented a predictive analytics platform that forecasts stock price movements. By integrating various data sources like market trends and news reports, the tool provides insights that inform trading strategies. This initiative led to a notable increase in the accuracy of their forecasts, effectively guiding investment decisions. Financial institutions can benefit enormously from leveraging sophisticated data forecasting tools in volatile markets.
3. Healthcare Industry
A major hospital chain, Cleveland Clinic, deployed a forecasting model for patient admissions. The tool examines historical admission data and external factors such as weather patterns and local events. This has enabled the clinic to prepare better for fluctuations in patient numbers, optimizing resource allocation and improving patient care outcomes. This case highlights the potential of forecasting in enhancing operational capabilities in healthcare.
"The applications of data forecasting across various industries exemplify its essential role in effective decision-making."
Lessons Learned from Implementations
Case studies reveal valuable lessons regarding the implementation of data forecasting tools. These insights can be extraordinarily beneficial for professionals considering similar investments.
1. Importance of Data Quality
All the examples underline the necessity of high-quality data. Accurate forecasts are contingent upon the quality of data input. Organizations must invest effort in cleaning and validating data to enhance the reliability of outputs.
2. User Training and Engagement
Successful implementation requires user buy-in and adequate training. Employees need to understand how to use tools effectively, fostering an environment that embraces data-driven decision-making.
3. Continuous Review and Adaptation
Forecasting tools need periodic review. As market conditions evolve, models may require recalibration. Organizations that maintain agility in adapting their forecasting methods tend to succeed more than those that do not.
4. Cross-functional Collaboration
Data forecasting relies on input from diverse departments. For instance, in the retail case, collaboration between marketing and supply chain teams was essential. Organizations can achieve better forecasting outcomes when departments share insights and data proactively.
Future Trends in Data Forecasting Tools
The realm of data forecasting is evolving rapidly. This evolution, fueled by advancements in technology, influences many aspects of business operations. Understanding future trends is essential for professionals who seek to remain competitive and informed. The integration of artificial intelligence and machine learning plays a crucial role in shaping these trends. Moreover, emerging technologies offer new opportunities and challenges that organizations must navigate carefully.
Advancements in AI and Machine Learning
Artificial intelligence and machine learning have become integral to data forecasting. These technologies enhance the accuracy and efficiency of forecasting models. With machine learning algorithms, systems can learn from historical data patterns without explicit programming. This ability allows for the identification of complex, nonlinear relationships in datasets that traditional models may overlook.
Some of the advantages of AI in forecasting include:
- Improved Prediction Accuracy: Machine learning algorithms can adapt based on new data, resulting in more reliable forecasts.
- Automation of Processes: Many routine tasks involved in data preparation or model selection can be automated. This saves time and reduces the possibility of human error.
- Real-Time Forecasting: AI capabilities allow for continuous learning and updating of models with real-time data.
This shift towards AI-driven forecasting tools necessitates that professionals be proficient not only in traditional statistical methods but also in these advanced technologies. Understanding how to interpret AI output is equally important for making informed decisions.
Emerging Technologies and Their Impacts
Several emerging technologies are impacting data forecasting significantly. Technologies such as blockchain, IoT, and big data analytics provide new avenues for enhancing forecast accuracy and data integrity.
For instance, the Internet of Things (IoT) generates massive amounts of data in real time. Harnessing this data can improve forecasting in industries like manufacturing and supply chain management. Blockchain ensures transparency and security in data collection and sharing. This is crucial when data integrity is paramount for accurate forecasting outcomes.
Key considerations regarding these technologies include:
- Data Management: With larger volumes of data comes the challenge of effectively managing and interpreting this data.
- Integration with Existing Systems: Companies must ensure new technologies work seamlessly with their current data infrastructure.
- Skill Development: Professionals need to continually update their skills to leverage these emerging technologies effectively.
As data forecasting tools advance, organizations must adapt to these changes to stay relevant. Embracing the future will involve understanding these trends and their implications while preparing for the challenges they may present.
Ending
The conclusion of this article is paramount, encapsulating the intricacies and vital insights related to data forecasting tools. It serves as the final synthesis, reiterating the significant points discussed throughout the text. Understanding the essence of data forecasting is more than a mere academic exercise. It is crucial for decision-making, strategic planning, and operational effectiveness across various industries.
Recap of Key Points
A thorough recap of the key elements reveals the complexity yet necessity of data forecasting tools. First, the article elucidated the definition and importance of data forecasting. The methods were classified into qualitative and quantitative, each with its characteristics, strengths, and weaknesses. The overview of tools highlighted the leading software available, emphasizing their functionalities.
Additionally, the criteria for selecting the right tool—such as functionality, ease of use, integration capabilities, and cost—were unpacked, giving readers a practical framework for evaluation. Implementing these tools comes with challenges and requires proper setup, training, and ongoing support. Real-world case studies demonstrated how different industries successfully navigated these tools, while future trends painted a picture of where this landscape is headed, including advancements in AI and machine learning.
Final Thoughts on Selection and Utilization
In selecting and utilizing data forecasting tools, professionals should prioritize a clear understanding of their specific needs and objectives. Not every tool fits every circumstance. It is essential to evaluate the tool's features against the actual requirements of the organization. As the landscape evolves, staying updated with trends will provide a competitive edge.
Furthermore, it is important to recognize that effective forecasting is an iterative process. Continuous refinement based on feedback and results ensures that the predictions become more accurate over time. Ultimately, leveraging the right data forecasting tools empowers businesses to make informed decisions, fostering growth and resilience in an ever-changing market.
"Forecasting is not just about predicting the future: it is about shaping it through informed decisions."
The landscape of data forecasting is intricate but essential. The tools discussed in this article can provide critical advantages to those ready to engage with them thoughtfully.