Exploring the Evolution of Conversational Analytics Tools
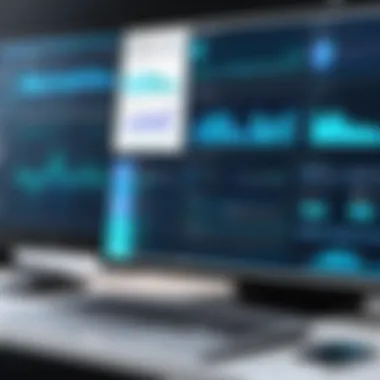
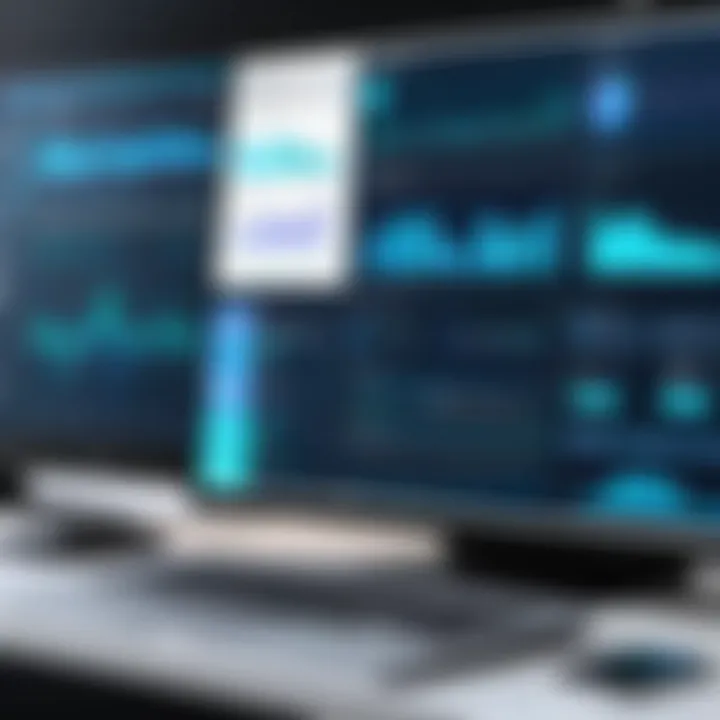
Intro
Conversational analytics tools have reshaped how businesses interact with their data. The rise of these tools has been significant in the last decade, primarily driven by advancements in natural language processing and artificial intelligence. People no longer need to be data scientists to extract valuable insights. Instead, they can simply ask questions in plain language, thus democratizing access to data. This has profound implications for various industries, enhancing user experience and business intelligence.
Through this article, we will explore the evolution of these tools, their key features, and how they impact organizations. From usability to performance, we aim to provide professionals in IT-related fields, students, and software developers with a comprehensive understanding of conversational analytics tools. By the end, readers will have a clearer picture of not only the technology itself but also its future trends and challenges in the business landscape.
Software Overview
Conversational analytics tools encompass a range of software solutions that allow users to engage with data through natural language interactions. Each tool may present different capabilities, but they generally aim to simplify data retrieval and analysis processes.
Key Features
- Natural Language Processing: This enables users to communicate with data in a way that feels intuitive, using phrases instead of complex queries, leading to improved accessibility.
- Real-Time Analytics: Many tools offer instant feedback, allowing users to explore data more dynamically and make prompt decisions based on findings.
- Integration with Data Sources: Conversational analytics tools often integrate seamlessly with existing databases and applications. This interoperability enhances data richness without requiring extensive changes to current systems.
- User-Focused Dashboards: Visualizations and dashboards are designed to be user-friendly, presenting complex data in an easily digestible format.
System Requirements
The system requirements for conversational analytics tools vary based on the software in question. However, several common requirements can typically be found:
- Operating System: Most tools run on Windows or macOS, while some may also support Linux platforms.
- Memory: A minimum of 8GB RAM is usually recommended, but 16GB or more is optimal for performance.
- Processor: Modern multi-core processors are preferred to handle data queries efficiently.
- Internet Connection: A stable and robust internet connection is essential, especially for cloud-based tools, allowing real-time data processing.
In-Depth Analysis
Understanding the intricacies of conversational analytics tools involves analyzing their performance and usability and their practical applications.
Performance and Usability
The effectiveness of any software tool largely depends on its performance and usability. Conversational analytics tools strive to offer speed, accuracy, and ease of use.
Performance can be evaluated through:
- Response Time: Quick retrieval and analysis of data when queries are made.
- Scalability: The capacity to handle increasing amounts of data without compromising performance.
- Accuracy: Delivering correct insights based on the queried data.
Usability examines how accessible and intuitive the tools are to a diverse user base. Successful tools must cater to technical and non-technical users, ensuring a broad range of individuals can extract value from the platform.
Best Use Cases
Conversational analytics tools can enhance various professional settings. Recommended use cases include:
- Customer Support: Analyzing customer inquiries in real-time to improve service efficiency.
- Market Research: Gaining insights from a vast amount of consumer data and feedback through simple queries.
- Performance Monitoring: Allowing businesses to track metrics and key performance indicators seamlessly.
"The evolution of conversational analytics tools is not just a technological advancement but a significant shift in how we think about and engage with data."
Prologue to Conversational Analytics
Conversational analytics represent a transformative approach to data interaction. It shifts how users engage with information by allowing inquiry through natural language. This enhancement is significant, as it democratizes data access. Users no longer require advanced technical skills to extract insights or navigate complex query languages. Instead, they can ask direct questions, making data more accessible to broad demographics.
The importance of conversational analytics is particularly evident in a fast-paced business environment, where timely decision-making is critical. Instruments that simplify interaction facilitate quicker insights, which can translate into strategic advantages. Moreover, these tools help organizations capture nuances in user behavior and preferences, providing a richer understanding of their client base.
By integrating conversational analytics into business intelligence frameworks, companies can elevate user experience. This leads to greater satisfaction and enhanced engagement with analytical tools. The widespread adoption of this technology indicates a shift towards more intuitive, user-friendly data applications. Companies looking to gain a competitive edge must understand this evolution and its impact on their operations.
Defining Conversational Analytics
Conversational analytics can be defined as the interrogation of data through natural language, using AI-driven interfaces. These tools rely on natural language processing to interpret user queries and provide meaningful responses. The interaction often resembles casual conversations, allowing for an organic and straightforward dialogue with data. This capability contrasts sharply with traditional analytics tools, which often require users to learn specific syntax or formats to generate useful reports.
The essence of conversational analytics lies in its ability to comprehend user intent. For instance, if a user types, "What were last quarter's sales?" the system parses this inquiry and retrieves the relevant data without necessitating any special input format from the user. This fluidity makes data analysis more approachable for all, not just data specialists.
Importance in Modern Data Interpretation
The role of conversational analytics in contemporary data interpretation cannot be overstated. As businesses generate ever-increasing volumes of data, the challenge lies in curating actionable insights from this abundance. Conversational analytics tools streamline this process by transforming raw data into comprehensible and relevant information.
Some benefits of conversational analytics in modern data interpretation include:
- Increased User Engagement: Users are likely to engage more with data when it is accessible via conversational interfaces.
- Faster Decision-Making: By reducing the time needed to understand data queries, organizations can make quicker strategic decisions.
- Enhanced Collaboration: Teams can discuss insights in real-time, facilitating collaborative efforts and comprehensive understanding.
- Personalized Insights: These tools can adapt to user preferences, providing tailored insights that resonate with individuals' specific contexts.
Historical Context
Understanding the historical context of conversational analytics tools is signifcant for appreciating their current functionality and importance. The development of analytics tools has been a gradual process that reflects the changing requirements of data analysis and the increasing complexity of user interactions with data.
The Development of Analytics Tools
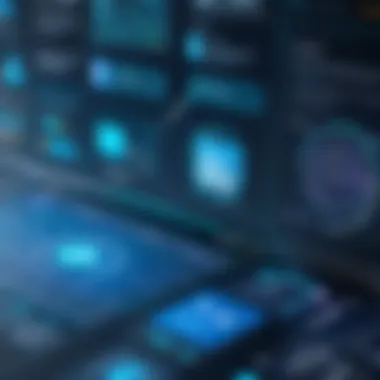
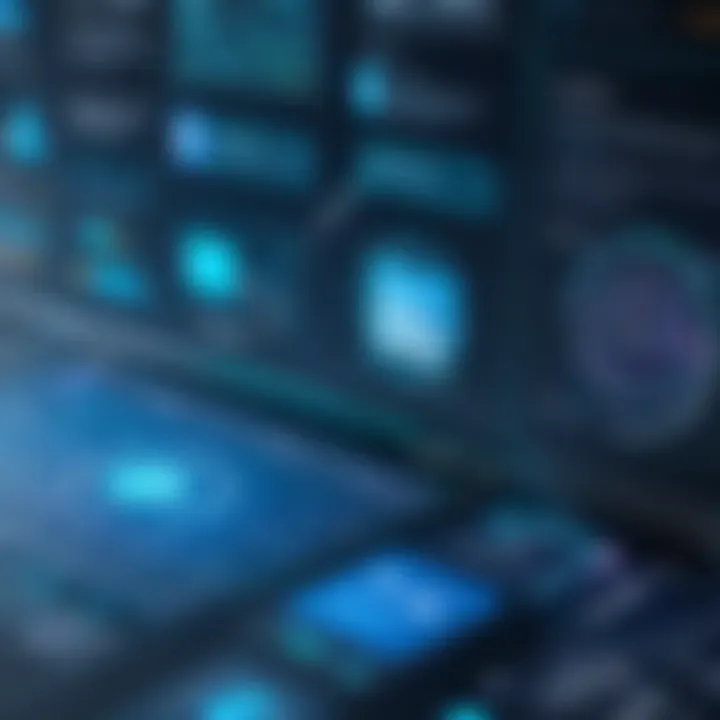
Analytics tools have existed for decades, evolving alongside technological advancements. Early analytics were primarily focused on quantitative data analysis. Software like Microsoft Excel laid the groundwork for data manipulation but relied on structured data sets. As businesses began realizing the importance of data-driven decisions, more sophisticated tools emerged. Programs such as SAP BusinessObjects and IBM Cognos made it possible to analyze large volumes of data effectively. However, these tools often required specialized knowledge to extract insights.
As data continued to grow in volume and complexity, the rise of big data analytics became apparent. Companies started utilizing platforms like Apache Hadoop and Google BigQuery, which allowed for the processing of massive data sets. Yet, these advancements still catered more to data experts and less to the average business user, who needed simpler and more intuitive ways to interact with data.
Evolution Toward Conversational Interfaces
The advent of conversational interfaces marked a pivotal shift in how users engage with data analytics. With the rapid adoption of smart devices and natural language processing capabilities, the demand for more intuitive interaction models became evident. Early examples like Appleβs Siri and Amazonβs Alexa demonstrated that voice commands could make technology more accessible.
As a result, developers started integrating conversational analytics into traditional analytics tools. Tools such as Microsoft Power BI and Tableau began incorporating natural language queries, allowing users to ask questions in plain language instead of sifting through dashboards. This transition enhanced user experience, enabling non-technical stakeholders to extract valuable insights without deep technical know-how.
"Conversational analytics tools can democratize data access, making it feasible for a wider range of users to grasp insights without needing extensive training."
This evolution towards conversational interfaces signifies a monumental leap. It demonstrates that understanding user needs can drive innovation in analytics. Tools that can interpret natural language facilitate a more dynamic interaction, satisfying the increasing expectation of convenience and efficiency in data analysis.
Core Technologies Behind Conversational Analytics
The core technologies that support conversational analytics tools are pivotal in bridging the gap between complex data sets and user-friendly interaction. These technologies enhance the ability of users to extract insights from vast amounts of data simply and intuitively. The benefits of investing in these core technologies are many including improved user engagement, increased efficiency in data retrieval, and ultimately, more informed decision-making across various sectors. By facilitating a conversational approach to analytics, these tools make data analysis accessible to broader audiences, not just data scientists.
Natural Language Processing Overview
Natural Language Processing, or NLP, stands at the forefront of conversational analytics technology. This subset of artificial intelligence focuses on the interaction between computers and human language. NLP enables programs to understand, interpret, and generate human language in a valuable way. Its importance in conversational analytics cannot be overstated.
NLP allows users to communicate with data through natural, conversational language. For instance, instead of writing complex SQL queries or using specialized software to dissect data, a user can simply ask, "What was our sales growth this quarter?" The NLP engine converts this human inquiry into a format that the underlying data system understands, executing the necessary analysis behind the scenes.
Some specific components of NLP crucial for conversational analytics include:
- Tokenization: Breaking down text into individual words or phrases for easier processing.
- Named Entity Recognition: Identifying and classifying key information in the text, such as names of products or organizations.
- Sentiment Analysis: Understanding the emotional tone behind a series of words, helping businesses gauge customer satisfaction.
The integration of NLP enhances user experience by making data exploration feel more like a dialogue, rather than a series of rigid commands. This tailored interaction is essential in modern applications where speed and clarity are key.
Machine Learning in Conversational Tools
Machine Learning plays a crucial role in refining the effectiveness of conversational analytics tools. It provides systems with the ability to learn from interactions and adapt over time. By analyzing patterns in how users query data, machine learning algorithms can improve the accuracy of responses and tailor future interactions to individual user preferences.
Some key aspects of machine learning relevant to conversational analytics are:
- Predictive Analytics: Anticipating user needs based on historical interactions, allowing for proactive data exploration.
- Classification Algorithms: Automatically sorting and categorizing data to streamline the analytics process and make relevant information more accessible.
- Reinforcement Learning: Continuously improving the system based on user feedback, enhancing both understanding and responsiveness.
"Machine learning not only predicts what a user may want but also personalizes the experience to meet those expectations."
The combination of machine learning and NLP creates a robust framework for conversational analytics. Businesses can leverage these technologies to drive more informed decisions and establish deeper engagements with their audience, leading to enhanced operational performance.
Functionality and Features
The functionality and features of conversational analytics tools are critical to understanding their impact on data analysis and user experience. These tools possess unique capabilities that transform how users interact with data. Their design enables non-technical users to access complex data insights simply by using natural language. This ease of use enhances productivity and democratizes data access, allowing more stakeholders within an organization to make informed decisions based on data.
User Interaction Models
User interaction models in conversational analytics tools focus on how users engage with the system. Typically, this interaction occurs through chat interfaces, voice commands, or even simple text inputs. A significant feature is the ability of these tools to understand context and follow the flow of conversation. This capability allows users to ask follow-up questions or clarify data points without needing to retrace their steps.
- Chatbots and Virtual Assistants: These are common interfaces for conversational analytics. Users can type queries and receive responses in a dialogue format. This direct interaction mimics human conversation, thus reducing the intimidation often felt when dealing with data analytics.
- Voice Command: As voice recognition technology improves, it is now feasible to conduct analyses through voice commands. This feature fosters hands-free operation, making it more accessible during multitasking jobs.
- Feedback Loops: Incorporating feedback mechanisms in these interfaces enables users to refine inquiries and tailor outputs according to their specific needs. This enhances user satisfaction and increases the tool's usefulness over time.
Incorporating these interaction models means organizations can engage their workforce at all levels. It empowers users from various backgrounds to gain insights from data without relying solely on data scientists or analysts.
Integration with Business Systems
Integrating conversational analytics tools with existing business systems is fundamental for maximizing their effectiveness. Businesses often rely on multiple software solutions for different functions, such as customer relationship management, project management, and sales tracking. Thus, seamless integration helps aggregate data from different sources into a unified view.
"Integration with existing systems is crucial to leverage the full potential of conversational analytics tools."
- APIs and Connectors: Most tools provide Application Programming Interfaces (APIs) that facilitate the connection to various data sources. This allows for real-time data insights, maintaining the timeliness and relevance of the information provided.
- Data Warehousing: Integrating with data warehouses ensures that the conversational analytics tools operate on a comprehensive dataset. This leads to more accurate and reliable insights.
- Streamlining Workflow: By connecting with other business systems, conversational analytics can automate certain processes and workflows. This streamlining reduces manual labor and the possibility of errors, enhancing efficiency across various operations.
Ultimately, understanding the functionality and features of conversational analytics tools provides a pivotal understanding of their significance in shaping data-driven decision-making processes in contemporary business environments.
Comparative Analysis of Key Tools
The comparative analysis of key tools in conversational analytics is crucial for understanding the varying capabilities and functionalities offered by different software. This analysis provides insight into how various tools perform in real-world applications. Users can benefit from knowing what features are most valuable, enabling better decision-making when selecting a tool for their specific needs. Understanding differences among tools can lead to optimizations in data interpretation and management, as well as enhancing user experience.
Leading Conversational Analytics Software
A few leading conversational analytics software are at the forefront, providing users with unique features and functionality. Among these, Google Dialogflow stands out for its natural language processing capabilities, allowing developers to create conversational interfaces seamlessly. IBM Watson Assistant is another popular choice, known for its deep learning and integration capabilities. Tools like Amazon Lex provide robust frameworks for building chatbots that integrate smoothly with AWS services.
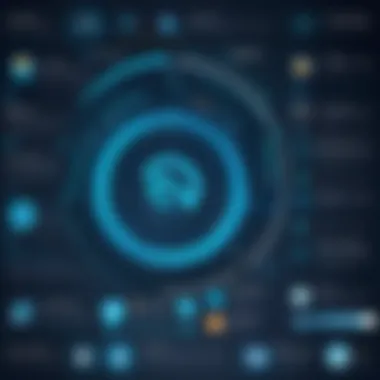
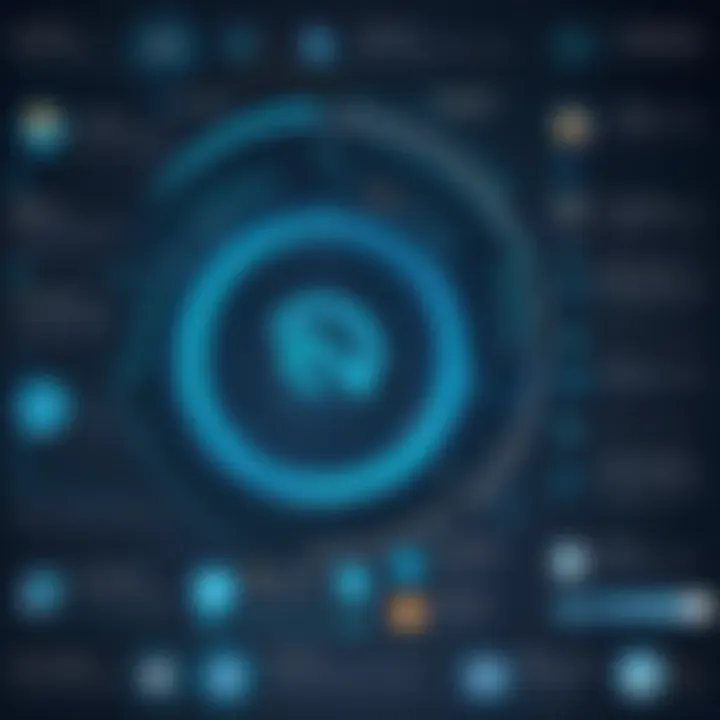
Additionally, Microsoft Bot Framework delivers a comprehensive approach for creating enterprise-level conversational solutions, while tools like LivePerson focus on messaging and customer engagement. Each of these tools offers distinct advantages, depending on the complexity of the project and the required integrations.
The choice of software typically depends on user experience, specific use cases, and integration capabilities with existing digital infrastructure.
User Feedback and Case Studies
User feedback plays a pivotal role in assessing the effectiveness of conversational analytics tools. Many organizations share their experiences through case studies, shedding light on the practical application of these tools. Practical examples illustrate how particular software can solve specific problems.
For instance, an enterprise utilizing IBM Watson Assistant reported a significant reduction in customer support response times, leading to increased customer satisfaction.
Conversely, some users express challenges, such as the learning curve associated with complex platforms like Microsoft Bot Framework.
"The integration process was not straightforward, but once we got through it, the efficiency improved dramatically." β Feedback from a tech lead.
When analyzing case studies, the following points often emerge:
- Efficiency Gains: Many companies report reductions in time spent on manual data handling.
- Customer Insights: Tools have enhanced the ability to collect valuable customer feedback and insights.
- Scalability Issues: Some tools struggle to scale with increasing demand, which can hinder performance.
In summary, the comparative analysis of key tools in conversational analytics highlights the importance of thoughtful selection. Understanding the comparative capabilities allows businesses to tailor their approach, ensuring they choose a tool that meets their specific goals and provides a strong return on investment.
Applications Across Industries
The application of conversational analytics tools is significant in various sectors, as they enable organizations to derive insights and make informed decisions through natural language interactions. These tools harness user-friendly interfaces, allowing users, regardless of their technical expertise, to engage with complex data sets easily. Businesses leverage these capabilities for enhanced decision-making, customer interaction, and operational efficiency.
Marketing and Sales Optimization
Conversational analytics plays a crucial role in marketing and sales. By utilizing these tools, companies can analyze customer interactions and feedback in real-time. This analysis allows marketing teams to identify trends and preferences, directing their strategies effectively.
Specific benefits include:
- Customer Insights: Understanding customer behavior and sentiment helps businesses tailor their marketing campaigns.
- Lead Management: Sales teams can use insights from conversations to prioritize leads and refine their pitches.
- Personalization: Conversations can reveal individual customer interests, leading to personalized offers and solutions.
The result is not just increased efficiency but also enhanced customer satisfaction. When businesses respond to the needs of customers promptly, it fosters loyalty and brand trust.
Healthcare Data Analysis
In healthcare, conversational analytics tools transform how data is interpreted and utilized. These tools assist in extracting meaningful insights from patient data through conversational queries, enabling healthcare providers to make better, evidence-based decisions.
Key applications include:
- Patient Engagement: By analyzing feedback and interactions, healthcare providers can improve patient experiences and outcomes.
- Operational Efficiency: Understanding staff resources and patient flow can lead to more efficient hospital operation management.
- Treatment Optimization: Conversational analytics helps in tracking treatment effectiveness and patient response.
Ultimately, these tools enhance patient care quality and operational management within the healthcare sector.
Financial Services Insights
Conversational analytics also has a vital presence in the financial services industry. They allow organizations to interpret vast amounts of data quickly through natural language queries, providing critical insights that influence investment and operational strategies.
Considerations include:
- Risk Management: Analyzing historical conversation data assists in identifying potential risks and issues.
- Customer Service Improvement: Financial institutions can focus on service quality based on real-time feedback analysis.
- Regulatory Compliance: Prompt and accurate analytics can help ensure compliance with financial regulations by interpreting necessary data correctly.
Overall, the implementation of conversational analytics tools in finance leads to improved decision-making and strategic planning, ultimately benefiting clients and financial institutions.
"Conversational analytics tools enable organizations not just to interpret data, but to interact with it in ways that were previously unimaginable."
The value of conversational analytics cannot be understated. Each industry harnesses these tools uniquely, emphasizing their adaptability and necessity in the evolving landscape of data analysis. Understanding their application across sectors equips professionals to leverage these technologies effectively for improved outcomes.
Challenges in Implementing Conversational Analytics
Implementing conversational analytics tools can be a complex endeavor for organizations. A broad overview reveals multiple challenges that decision-makers must consider. Understanding these challenges is crucial as they impact data management, user engagement, and ultimately the return on investment. Making informed choices requires an awareness of issues such as data privacy, security, and the overall accuracy of the systems in use.
Data Privacy and Security Concerns
Data privacy is becoming increasingly important in an era where information is constantly being collected and analyzed. Conversational analytics tools often handle sensitive data, which can lead to significant privacy risks if not managed correctly. Organizations must comply with regulations such as GDPR in Europe or CCPA in California, which dictate how personal information must be handled.
The challenges include:
- Data Protection: Ensuring that data is encrypted both in transit and at rest to minimize the risk of breaches.
- User Consent: Implementing processes that inform users about data collection and securing explicit consent.
- Data Retention Policies: Establishing how long data will be stored and the measures for its secure deletion.
The threat of cyber attacks is another concern. A breach could not only compromise user information but also damage an organization's reputation. The potential fallout emphasizes the importance of rigorous security measures when deploying conversational analytics tools.
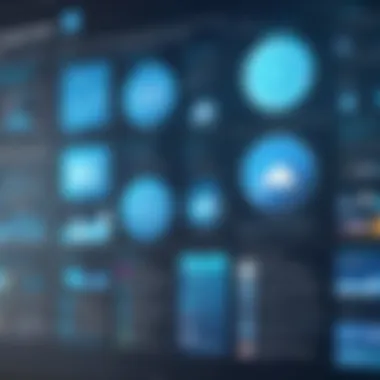
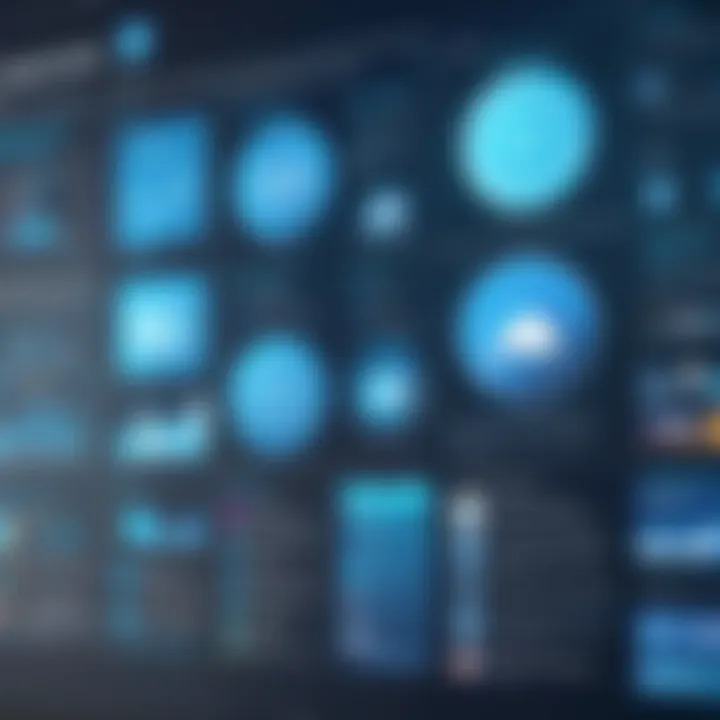
"The right blend of security protocols and user trust is essential for the successful integration of these tools."
Accuracy and Reliability Issues
Accuracy in conversational analytics is paramount. Users rely on the data and insights generated for decision-making. If tools misinterpret queries or provide erroneous data, it could lead organizations astray.
Some key challenges related to accuracy and reliability include:
- Natural Language Processing Limitations: While advancements have been made in NLP, there are still limitations in understanding context, slang, or idioms, which can hinder accurate interpretation.
- Training and Dataset Quality: The performance of machine learning models heavily depends on the quality of the training data. Poorly labeled or biased datasets can result in inaccurate outputs.
- Real-Time Data Processing: Achieving real-time analysis without sacrificing accuracy is a delicate balance that requires robust systems.
Inaccurate results not only diminish the utility of conversational analytics but also erode user trust. Organizations must prioritize proper model training, dataset selection, and continuous evaluation to ensure reliability.
Standardization and Best Practices
Establishing standardization and best practices is crucial in the realm of conversational analytics tools. As organizations increasingly adopt these tools, ensuring reliable and consistent performance becomes necessary. The significance lies not only in improving efficiency but also in fostering user trust. When users know what to expect from these tools, their confidence in using them increases, leading to better adoption rates.
Standardization facilitates seamless integration across various platforms. When different tools follow similar protocols, the process of switching between them or integrating multiple systems becomes easier. This reduces friction for users who need to navigate through different interfaces with distinct functionalities and designs.
Moreover, best practices promote effective utilization of conversational analytics. They guide businesses in implementing these tools in ways that maximize their analytical capabilities while minimizing errors or misinterpretations. By adhering to a set of proven guidelines, organizations can better harness insights from their data.
"Standardization in conversational analytics not only improves usability but also enhances data integrity and security, essential for modern enterprises."
Establishing Best Practices for Usage
Best practices for using conversational analytics tools encompass a range of strategies that emphasize clarity, user engagement, and data integrity. First, training users adequately on how to interact with these tools cannot be overlooked. Comprehensive training programs educate users on expectations and capabilities, which helps in minimizing frustration and errors in data analysis.
Furthermore, businesses should encourage users to provide feedback on their experiences with the tools. Regularly analyzing this feedback allows organizations to adjust processes and interfaces, addressing any usability concerns as they arise.
Maintaining an updated knowledge base is also essential. This resource serves as a point of reference where users can find answers to common inquiries or challenges they might encounter. By fostering a community approach to knowledge sharing, companies can further enhance user experience and engagement.
Standardization Across Tools
Standardization across conversational analytics tools is vital for ensuring interoperability and user familiarity. As the market becomes saturated with various software solutions, it is imperative that they follow a uniform set of standards. This unification is essential for creating a cohesive user experience across different platforms.
Interoperability ensures that data can move freely between tools without significant alterations in format or structure. Adopting common data formats can save considerable time in analysis as data does not require extensive reformatting before use in another tool.
In addition, standard definitions for metrics and reporting can help avoid confusion in analytics. When everyone is using the same terminology and frameworks, it becomes easier to draw insights and communicate findings across departments.
Future Trends in Conversational Analytics
The future of conversational analytics is a pivotal topic in the ongoing evolution of how data is analyzed and utilized. As organizations aim to extract meaningful insights from large datasets, the ability to communicate with data through natural language is becoming indispensable. Conversational analytics tools not only democratize data access but also enable faster decision-making. In this context, it is essential to explore innovations that will shape these tools and the crucial role of artificial intelligence in enhancing analytics capabilities.
Innovations on the Horizon
The landscape of conversational analytics is rapidly changing. Several innovations are anticipated to revolutionize how users interact with data. Here are key trends expected to emerge:
- Voice Recognition Enhancements: Technology advancements are leading to more sophisticated voice recognition systems. Users will find it easier to query data verbally, significantly improving user experience.
- Contextual Awareness: Future tools will incorporate contextual information to provide deeper insights. For example, a tool might consider the user's previous interactions and adapt responses based on historical data.
- Integration with IoT Devices: As the Internet of Things continues to expand, conversational analytics will likely integrate with various IoT devices. This will allow users to derive insights from real-time data across multiple platforms seamlessly.
- Improved Multimodal Interfaces: The ability to interact through both text and voice, and even visual data representations will offer users more flexibility. This multimodal approach will cater to diverse user preferences, making data more accessible.
These innovations promise to not only enhance the user experience but also to drive deeper insights from data.
The Role of AI in Enhancing Analytics
Artificial Intelligence is at the core of transforming conversational analytics tools. As these tools become more sophisticated, AI will play several crucial roles:
- Natural Language Understanding (NLU): AI technologies will continue to improve NLU capabilities, enabling tools to interpret user intentions accurately and respond in a meaningful way.
- Predictive Analytics: By leveraging machine learning algorithms, future conversational analytics tools will provide predictive insights. Organizations will be able to anticipate trends and customer behavior, which can lead to proactive decision-making.
- Sentiment Analysis: AI will analyze user sentiment, suggesting adjustments in strategy based on user feedback. Understanding customer emotions allows businesses to craft personalized experiences.
- Automated Insights: As tools evolve, AI will provide automated insights, reducing the time taken for data analysis. Users can receive immediate answers and more efficient workflows.
"The coming wave of advancements in conversational analytics is not just about tools; it's about reshaping how we think about data interaction altogether."
Focusing on these trends and innovations is critical for software developers and IT professionals who are poised to impact this field significantly. They must remain abreast of these developments to harness the potential of conversational analytics effectively.
Finale
In the fast-paced realm of data analysis, conversational analytics tools have carved a significant niche. Their evolution represents a shift towards more intuitive interactions with data. This development has broad implications for various sectors, from marketing to healthcare. Conversational analytics not only simplifies the process of data interpretation but also democratizes access to insights that were once the domain of data specialists.
Summary of Key Takeaways
At the core of this article, several key elements about conversational analytics tools stand out:
- User-Centric Design
These tools prioritize user experience, enabling individuals to engage with data using natural language. This reduces the barriers to understanding complex datasets. - Integration Capabilities
Most conversational analytics solutions easily integrate with existing business systems. This allows organizations to leverage their current data while gaining insights more efficiently. - Machine Learning and AI
Ongoing advancements in these fields enhance the capabilities of conversational analytics, making them not only more effective but also increasingly proactive in problem identification and trend detection. - Application Versatility
From sales strategies to patient outcomes in healthcare, these tools find relevance across various industries, demonstrating their adaptability and necessity in contemporary business environments. - Challenges Ahead
While promising, the journey of conversational analytics is not without its hurdles. Issues surrounding data privacy, security, and the accuracy of insights pose significant challenges that need addressing as the technology evolves.
Final Thoughts on Future Directions
Looking ahead, the future of conversational analytics tools is bright yet complex. As companies become more reliant on data-driven decisions, the need for advanced analytics solutions will only increase. The role of artificial intelligence in this context cannot be overstated. AI is poised to enhance the predictive capabilities of these tools, providing insights that are not just reactive but forward-looking.
Moreover, as the data landscape continues to evolve, standardization in conversational analytics will become critical. Establishing common frameworks and best practices will help ensure that businesses can seamlessly adopt and benefit from these technologies.
"Conversational Analytics Tools are more than just a trend; they represent a fundamental shift in how we interact with data."