Exploring Automatic Trade Systems: An In-Depth Analysis
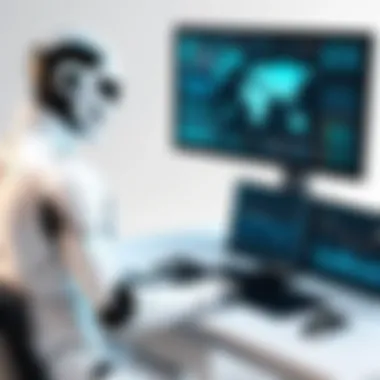
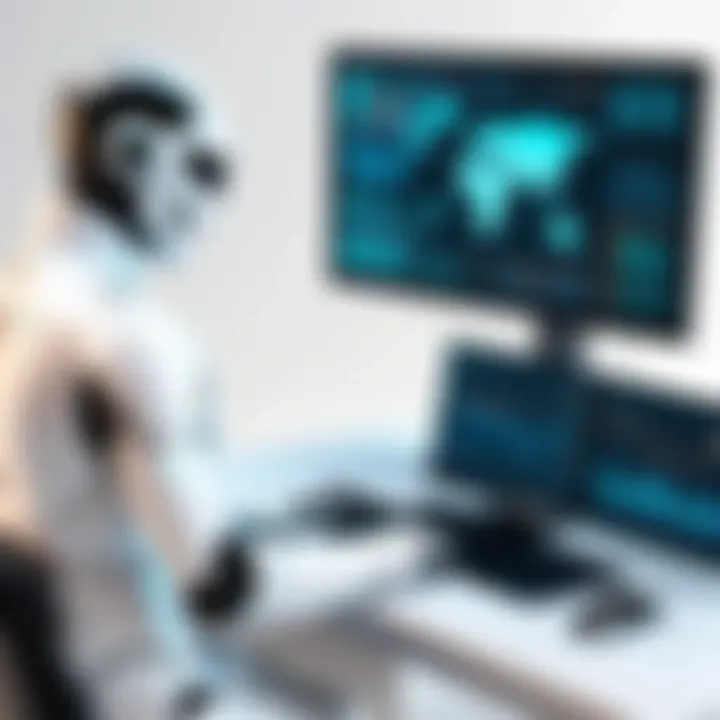
Intro
Automatic trade systems have gained significant prominence in the financial markets over the last few decades. This technology allows traders to execute trades with minimal human intervention. Understanding these systems becomes crucial for both novice and experienced investors. They offer speed, precision, and a systematic approach to trading that is often difficult to achieve manually.
In this article, we will explore the intricate details of automatic trade systems. We will examine their functionalities, benefits, and potential drawbacks. Insights from experts as well as real user experiences will enrich this analysis. The goal is to provide a comprehensive overview that empowers readers to navigate the complexities of automatic trading technology effectively.
Software Overview
Automatic trading systems, also known as algorithmic trading systems, rely on sophisticated software to execute trades based on predefined criteria. Here, we will outline their key features and system requirements.
Key Features
- Algorithmic Trading Strategies: These systems allow the implementation of various strategies, such as arbitrage or market making.
- Real-Time Data Analysis: They provide instant analysis of market data, enabling quick decision-making.
- Risk Management Tools: Built-in risk management features help minimize losses and protect capital.
- Customizable Interfaces: Users can often tailor the interface to suit their trading preferences, making it user-friendly.
- Backtesting Capabilities: Before live trading, strategies can be tested on historical data to assess their effectiveness.
System Requirements
Automatic trading systems typically require the following:
- Operating System: Most systems are compatible with Windows, Linux, or macOS, though specifics depend on the software.
- Internet Connection: A stable and fast internet connection is essential for real-time data access.
- Sufficient Memory and Processing Power: System performance may require substantial RAM and CPU processing power, depending on the complexity of trading strategies.
In-Depth Analysis
The real efficacy of automatic trade systems lies in their performance and how user-friendly they are. Understanding these aspects can help traders choose the right system for their needs.
Performance and Usability
Performance of automatic trading systems can vary widely. Some can process thousands of orders in seconds, while others might struggle with even basic tasks.
Usability is another critical factor. A well-designed interface can make complex functionalities more accessible. Users report differing levels of satisfaction based largely on how intuitive the system is.
Best Use Cases
Automatic trading systems are best leveraged in the following scenarios:
- High-Frequency Trading: Used by institutional traders who execute a large number of trades in short periods, relying on speed.
- Market Trend Analysis: For traders who focus on following trends over extended periods, automatic systems can help identify entry and exit points effectively.
- Risk Diversification: By utilizing algorithms that operate across different markets and instruments, investors can spread risk efficiently.
"With algorithmic trading, decisions are made on data analyses rather than emotional impulses. This shifts the trading paradigm significantly."
Understanding these dimensions of automatic trade systems can position investors and traders to make informed decisions. The world of automated trading is evolving, and keeping abreast of trends and technologies is essential for success.
Prelims to Automatic Trade Systems
Automatic trade systems have become an integral part of modern financial markets. Their ability to analyze massive datasets and execute trades in split seconds makes them invaluable tools for investors and traders. Understanding these systems is crucial in todayโs fast-paced trading environment, where milliseconds can determine profit and loss. This section provides a thorough grounding in the essential components and historical developments that led to the current state of automatic trading.
Defining Automatic Trade Systems
Automatic trade systems, often referred to as algorithmic trading systems, utilize predefined rules and algorithms to trade financial instruments. These systems can process data far beyond human capacity, leading to more efficient market engagement. They operate based on various strategies such as trend following, mean reversion, or statistical arbitrage. The automation aspect minimizes the need for direct human intervention, allowing for trades to be executed quickly and precisely.
"Automatic trading systems leverage technology to enhance decision-making, reduce emotional bias, and improve execution efficiency."
The systems can be programmed to follow specific trading signals or market conditions, adapting quickly to changes in the financial landscape. They can also continuously improve through backtesting, enabling users to refine strategies based on historical data outcomes.
Historical Context
The roots of automatic trading systems can be traced back several decades. The evolution began with the introduction of electronic trading platforms and advanced computing in the late 20th century. Initially, trading was done manually, involving substantial risk and potential for human error. As markets became more electronic, traders sought methods to enhance speed and efficiency in their operations.
By the early 2000s, algorithmic trading gained significant traction following the rise of high-frequency trading firms. This marked a turning point, as these companies began using complex algorithms to purchase and sell in milliseconds, creating a somewhat volatile trading environment. Over time, regulatory changes affected the development of these systems, leading to the establishment of best practices and compliance measures.
In recent years, the integration of advanced technologies such as artificial intelligence and machine learning has further transformed these systems. Now they not only execute trades but also analyze market sentiments and complex patterns, allowing for more informed trading decisions. Understanding this historical context helps appreciate the rapid advancements and challenges that come with automatic trading systems today.
Core Components of Automatic Trade Systems
Automatic trade systems operate on several key components that are crucial for their functionality and effectiveness. Understanding these components is vital for professionals seeking to implement or enhance these systems. Each element plays a distinct role in ensuring that trades are executed efficiently and reliably. In this section, we will explore specific components such as algorithmic trading strategies, data sources and inputs, and execution mechanisms.
Algorithmic Trading Strategies
Algorithmic trading strategies form the backbone of any automatic trading approach. These strategies utilize mathematical models and statistical analyses to make trading decisions based on market data. The types of strategies are varied and can include market-making, trend-following, and statistical arbitrage, among others.
One significant benefit of algorithmic trading strategies is their ability to process large amounts of data quickly. This capacity allows traders to make decisions that would be impossible for humans to execute with the same speed and accuracy. Moreover, because these strategies are based on pre-defined rules, they eliminate emotional trading biases, leading to more consistent results.
It is essential to note that these strategies must align properly with the overall trading goals. Traders often backtest their strategies using historical data to evaluate their effectiveness before live trading. The need for constant refinement and adaptation is crucial since market conditions can change rapidly.
Data Sources and Inputs
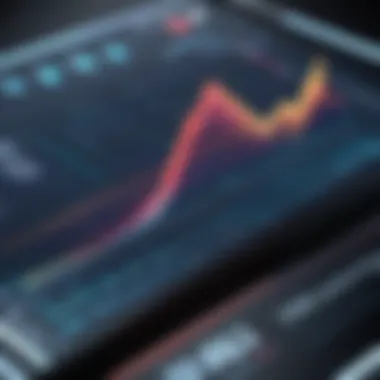
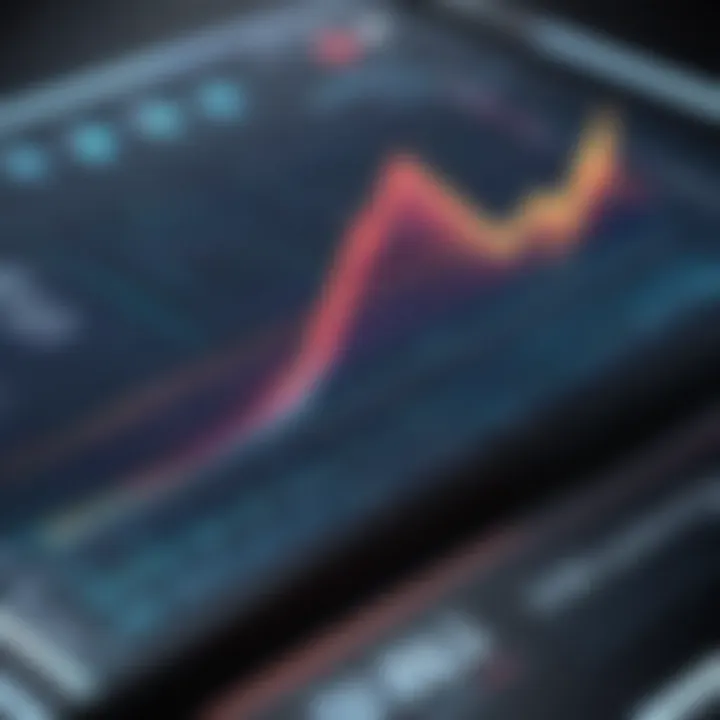
Data is the lifeblood of automatic trading systems. Reliable and timely data sources are fundamental for the successful implementation of trading strategies. These sources can include market feeds, news articles, and economic indicators. In many cases, high-frequency traders rely on ultra-low latency data to stay ahead of the competition.
Integrating diverse data sets can enhance strategy performance significantly. For example, leveraging both price data and alternative data, such as social media sentiment, can provide traders with comprehensive insights into market behavior. This integration requires sophisticated data management tools to ensure that the information is accurate and formatted correctly for analysis.
The inputs to an automatic trading system must be meticulously curated. Poor data quality can lead to suboptimal trading decisions, resulting in financial losses. Furthermore, as new data sources emerge, keeping pace with advancements in data acquisition technology is essential for traders.
Execution Mechanisms
Execution mechanisms are the processes by which buy and sell orders are transmitted to the market. Efficient execution is critical, particularly in high-frequency trading scenarios where even milliseconds can dictate profit or loss. Automatic trade systems utilize various methods, including direct market access and algorithmic execution strategies, to optimize order execution.
Multiple factors influence execution effectiveness, such as market liquidity and order type. For instance, using market orders typically leads to immediate execution but might incur higher costs due to slippage, while limit orders can control entry prices but may not execute instantly.
Traders must assess their execution strategies while evaluating their systems. Monitoring execution quality is essential to identify inefficiencies and reduce trading costs. Developing an execution strategy that aligns with market conditions and the specific trading goals is paramount for long-term success.
In summary, the core components of automatic trade systems are fundamental in shaping how trades are conducted in todayโs dynamic markets. Algorithmic strategies, robust data management, and optimized execution mechanisms collectively drive the effectiveness of these systems. Understanding these elements will provide traders and professionals with the tools they need to navigate the complexities of modern trading environments.
Types of Automatic Trade Systems
Understanding the various types of automatic trade systems is crucial for anyone involved in financial technology. Each type has distinct features, advantages, and ideal use cases. By grasping the differences among these systems, traders can make informed choices that align with their trading strategies and risk tolerance. This section will clarify the three main categories: High-Frequency Trading, Swing Trading Systems, and Arbitrage Systems.
High-Frequency Trading (HFT)
High-Frequency Trading represents one of the most advanced techniques in automatic trading systems. HFT involves executing a large number of orders at extremely high speeds. This requires sophisticated technology, including low-latency data feeds and direct market access.
The essential goal of HFT is to capitalize on very small price discrepancies. Traders engaging in HFT aim for profit from minor price variations that may exist for just seconds. Usually, trades might last less than a second. This means that profits per trade are slim, but volume is what creates the potential for significant returns.
Key Features of High-Frequency Trading:
- Speed: HFT systems operate on microsecond timeframes, which is critical for capitalizing on fleeting market opportunities.
- Algorithms: These systems rely on complex algorithms that analyze market data and execute trades without human intervention.
- Market Making: Many HFT firms act as market makers, providing liquidity to markets, which helps in smoothing price fluctuations.
Despite its advantages, HFT also comes with challenges, particularly regarding market stability. The reliance on speed may lead to issues if the technology falters. Understanding these nuances is vital for practitioners in the field.
Swing Trading Systems
Swing trading systems represent a middle ground between day trading and long-term investing. Unlike HFT, which focuses on milliseconds, swing trading aims to capture gains over several hours or days. Traders utilizing swing trading systems typically analyze market trends and use technical indicators to plan their entry and exit points.
These systems take advantage of market volatility and often rely on a combination of technical analysis and market sentiment. Swing traders typically look for swings in asset prices, aiming to profit from price movements over short to medium timeframes.
Benefits of Swing Trading Systems:
- Flexible Timeframe: These systems allow traders to hold positions longer than HFT, which can result in less stress and time commitment.
- Lower Transaction Costs: Fewer trades can mean lower fees compared to the multitude of trades executed in HFT.
- Potentially Higher Returns: Successful swing trades can yield significant profits since they capture larger price movements than HFT transactions often do.
Overall, swing trading systems are ideal for those who prefer a balanced approach in automated trading and wish to engage in analysis without a constant monitoring commitment.
Arbitrage Systems
Arbitrage systems focus on profit exploitation from price differences between markets. This form of trading can involve multiple exchanges or even different financial instruments. The concept of arbitrage is fundamentally predicated on the principle that markets should reflect similar asset values. However, temporary inefficiencies can arise, creating opportunities for arbitrage.
Common Types of Arbitrage:
- Spatial Arbitrage: This involves purchasing an asset in one market and simultaneously selling it at a higher price in another market.
- Statistical Arbitrage: This strategy uses statistical models to find price differences and execute trades based on predicted price convergence.
- Triangular Arbitrage: In forex markets, it can be done by converting one currency into another through a third currency, capitalizing on the rate differentials.
Arbitrage systems tend to be less risky since they often rely on simultaneous execution to eliminate exposure to market movements. However, they require robust technology and quick decision-making, as price discrepancies tend to persist only for very short durations.
These systems exemplify the versatility and dynamism present in automated trading options, revealing multiple avenues for developers and traders in the financial landscape.
Advantages of Automatic Trade Systems
Automatic trade systems offer significant benefits in the dynamic world of finance. Their growing popularity stems from unique advantages that transform trading strategies. Understanding these advantages equips investors and tech-savvy professionals with informed tools for market engagement.
Efficiency and Speed
Automatic trade systems excel in their efficiency and speed. These systems process large volumes of data faster than a human trader can. By utilizing advanced algorithms, they execute trades within milliseconds. This speed is critical in volatile markets where prices fluctuate rapidly.
"In automated trading, losing mere seconds can lead to significant financial losses."
Efficiency also extends to the management of multiple trading strategies concurrently. Traders can deploy various algorithms across different markets at the same time. This high level of automation reduces human error and allows for seamless execution of complex strategies without fatigue.
Emotionless Trading
One major benefit of automatic trading systems is their ability to trade without emotional interference. Human traders often succumb to fear, greed, and panic, which can lead to poor decision-making. Automatic systems rely solely on defined algorithms and strategies, stripping away emotional factors. This leads to disciplined and consistent trading, even in stressful situations.
By promoting a systematic approach, these systems allow traders to adhere to their strategies. A well-designed automatic trade system follows preset rules, minimizing the influence of emotions and enabling rational decision-making. As a result, investors can focus on the long-term performance rather than reacting to short-term market fluctuations.
Backtesting Capabilities
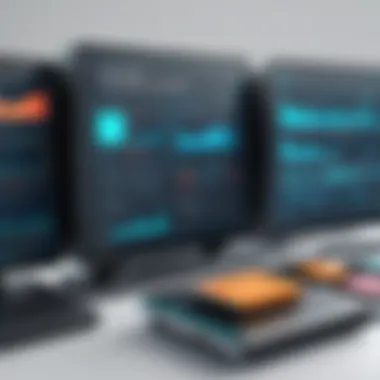
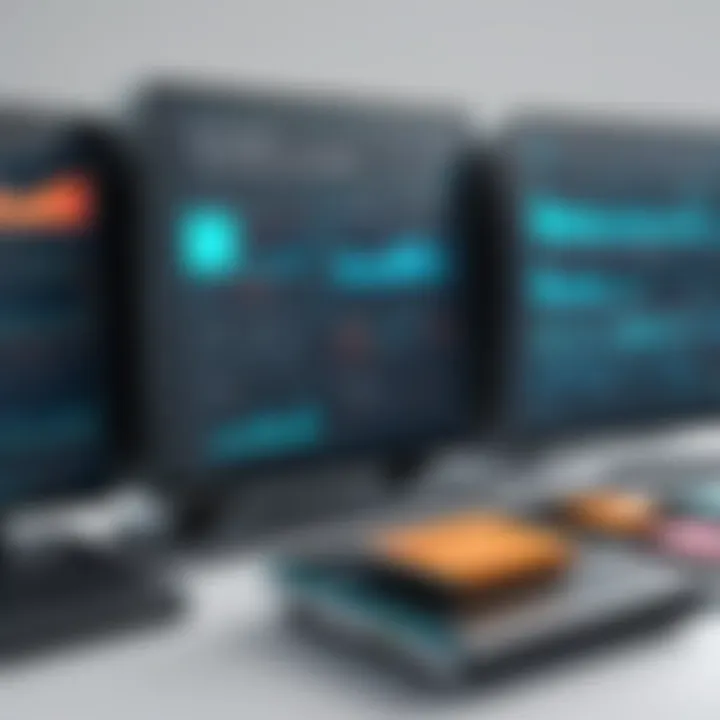
Backtesting is an essential component of automatic trade systems. This capability enables traders to test their strategies against historical data before implementing them in real-time. With backtesting, users can analyze how tactics would have performed under various market conditions.
Employing backtesting tools provides valuable insights. It allows traders to refine their strategies based on historical performance. Traders can assess potential risks and adjust parameters accordingly to optimize future outcomes.
Backtesting can be executed quickly and efficiently. Unlike traditional testing methods, automatic systems save time and resources when evaluating numerous market scenarios.
The focus on backtesting reinforces strategic planning and risk management. Investors can make more informed decisions by understanding potential pitfalls before live trading.
Challenges and Risks of Automatic Trade Systems
In the evolving landscape of finance, automatic trade systems present significant advantages. However, delving into these benefits necessitates an understanding of the associated challenges and risks. A comprehensive grasp of these issues can enhance investorsโ decision-making processes and mitigate potential pitfalls. By recognizing the inherent challenges, traders can implement more robust strategies that account for risks, ultimately improving their success rates.
Overfitting in Algorithms
Overfitting occurs when a trading model is excessively complex, capturing noise rather than underlying patterns in historical data. As a result, the algorithm will perform well with backtested data but fail to generalize when applied to real-time markets. This phenomenon emphasizes the necessity of striking a balance between model complexity and the accuracy of predictions.
- Key considerations about overfitting:
- Validation: It is crucial to validate the model using out-of-sample data to confirm its performance.
- Simplicity vs. Complexity: A simpler model can often provide better performance by focusing on the essential patterns without incorporating extraneous correlations.
- Continual assessment: Algorithms should undergo regular assessments to adapt to changing market conditions.
Market Volatility
Market volatility can dramatically impact the functioning of automatic trade systems. Factors such as political events, economic reports, or unexpected market movements can lead to rapid price changes. High volatility can wreck strategies that rely on stable market conditions.
- Impacts of market volatility:
- Slippage: During fast price movements, orders may execute at different prices than intended, affecting profitability.
- Drawdowns: Unexpected volatility may cause significant drawdowns, challenging risk management protocols.
- Reevaluation of strategies: Traders must consistently revise their strategies in light of market volatility to ensure effectiveness.
Technical Failures
Technical failures pose a significant risk in automatic trading systems. These failures range from software bugs to hardware malfunctions and include connectivity issues with trading platforms. Such failures can result in missed opportunities or cause unintended trades at inappropriate times.
- Preventing technical failures:
- Redundancy systems: Implementing backup systems and fail-safes can minimize the impact of failures.
- Regular updates: Frequent software and hardware updates will help maintain optimal performance.
- Monitoring: Continuous monitoring of system performance ensures that any anomalies are quickly identified and addressed.
In summary, while automatic trade systems offer potential advantages, understanding their challenges and risks is crucial for long-term success. Addressing overfitting, market volatility, and technical failures can significantly enhance the effectiveness and sustainability of these trading systems.
Regulatory Considerations
Understanding the regulatory landscape surrounding automatic trade systems is crucial for anyone operating in the financial markets. Regulations shape the environment in which these systems function, affecting everything from algorithm design to operational protocols. For software developers and professionals in IT-related fields, grasping the nuances of compliance is essential to build systems that not only perform efficiently but also adhere to existing laws and guidelines. As more traders leverage automation technology, the scrutiny on these systems intensifies, emphasizing the need for robust compliance measures.
Compliance Requirements
Regulatory compliance is a multifaceted endeavor. It typically encompasses a range of requirements designed to ensure fair trading practices, integrity in the markets, and protection of investors. Various jurisdictions enforce different regulations, each with specific criteria that trading systems must meet. Key compliance elements include:
- Licensing: Automatic trading systems often require operators to obtain licenses, demonstrating their operation within the bounds of the law. This may involve registration with financial authorities such as the Securities and Exchange Commission (SEC) in the United States.
- Risk Management Procedures: Firms must implement comprehensive risk management strategies. This is to guard against potential algorithmic failures or market anomalies that could lead to significant losses.
- Data Privacy: Adherence to data protection laws, such as the General Data Protection Regulation (GDPR) in Europe, is mandatory. Firms must ensure that the personal data of traders is handled securely and ethically.
- Reporting Standards: Automated systems may face requirements for regular performance reporting. This includes the documentation of trades executed under their algorithms to promote transparency.
Adhering to these compliance requirements not only mitigates legal risks but also enhances the trustworthiness of trading systems.
Impact of Regulations on Trading Systems
The impact of regulations on automatic trade systems can be profound, influencing both design and functionality. Regulatory changes often necessitate swift adaptations, challenging developers to ensure that their systems comply with new rules without sacrificing performance. Some notable impacts include:
- Increased Complexity: Regulatory compliance can significantly complicate the architecture of trading systems. Developers must build in flexible features that enable the system to adapt to changing legal landscapes. Algorithms may require adjustments to meet new guidelines without hindering their performance.
- Higher Operational Costs: Compliance can increase operational costs. Firms must allocate resources for compliance personnel, legal consultation, and regular audits. These expenses could impact the overall profitability of automatic trading systems.
- Evolving Standards for Risk Management: Regulations are increasingly emphasizing risk management frameworks. This focuses on systematic approaches to identifying, measuring, and controlling risks associated with trading activities. As a result, traders may find that fewer high-risk strategies are permissible under new rules.
"Regulatory considerations serve as both a challenge and a necessary component of effective trading strategies. Compliance promotes both market integrity and investor confidence."
As the regulatory environment evolves, it is paramount for developers, traders, and financial firms to stay informed and agile, ensuring their automatic trade systems maintain compliance while achieving their operational goals.
User Experiences and Case Studies
User experiences and case studies are critical in understanding the real-world application of automatic trade systems. Practical insights from users reveal both the strengths and weaknesses of these systems. Investors and developers often look for verified results to inform their choices and refine their strategies. By exploring how these systems perform in different scenarios, stakeholders can derive lessons that guide future implementations and avoid common pitfalls.
Successful Implementations
Successful implementations of automatic trade systems provide a benchmark for those entering the field. Variations in trading strategies, market conditions, and system configurations lead to a wide range of outcomes. Here are key aspects to consider:
- Real-world Examples: High-frequency trading firms often showcase successful deployment of auto-trading systems, which have improved their market access and execution speed. These firms frequently share their results, demonstrating enhancements in performance metrics.
- User Testimonies: User feedback from platforms like Reddit or traders' forums highlights positive experiences when systems are effectively tailored to their trading style. For instance, users report significant gains when algorithms are optimized for specific market conditions, such as volatility or liquidity.
- Efficiency Metrics: Successful case studies often present quantifiable metrics, such as reduced trade execution time or higher profit margins compared to traditional manual trading. This data serves as a testament to the efficacy of the trading strategies employed.
Lessons from Failures
On the other hand, lessons from failures illustrate the complexities and risks involved in automated trading. Analyzing case studies of unsuccessful implementations offers vital insights into what can go wrong:
- Overdependence on Technology: Some traders relied too heavily on their automated systems without sufficient oversight, leading to considerable losses during market anomalies. Understanding these trends can help others maintain a balanced approach between automation and manual intervention.
- Algorithmic Blind Spots: Failure to account for market asymmetries or sudden economic shifts often resulted in significant losses. By examining these cases, developers can improve their algorithms to be more adaptive in changing environments.
- User Feedback Importance: Many failures stemmed from a lack of user feedback during development. Ensuring that end-users are involved in the process helps identify potential issues before they manifest in live trading scenarios.
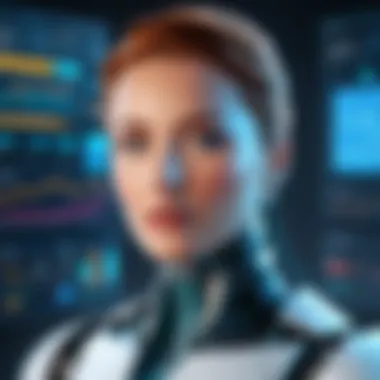
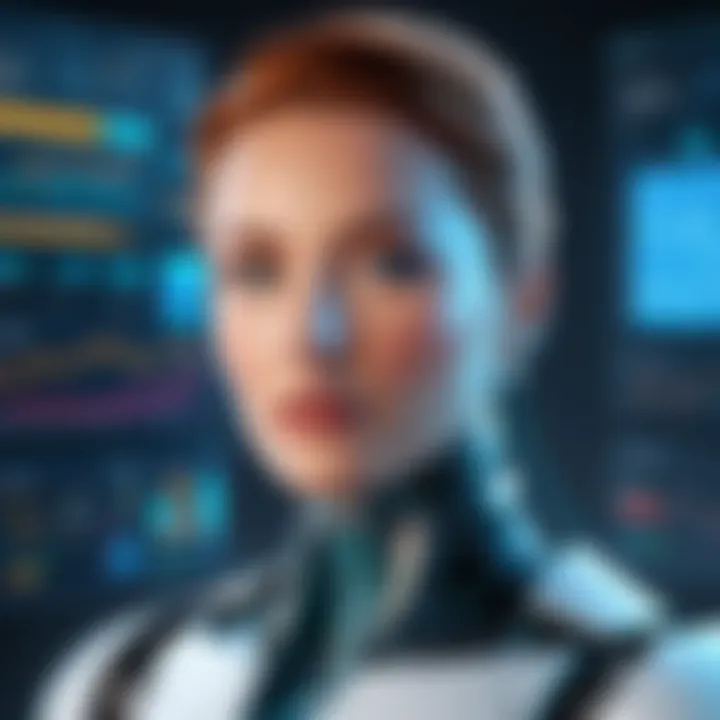
In summary, user experiences and case studies form an essential part of understanding automatic trade systems. They not only highlight success stories but also provide valuable lessons to mitigate risks. Investors and developers can leverage this information to make more informed decisions that enhance their trading strategy.
Future Trends in Automatic Trade Systems
As we move further into the era of digital finance, the evolution of automatic trade systems continues to gain momentum. Recent technological advancements play a crucial role in shaping the future of these systems, making it necessary for both industry professionals and investors to remain informed about emerging trends. Understanding these future trends allows stakeholders to identify opportunities and mitigate potential risks associated with automated trading. This section covers two primary areas: the integration of artificial intelligence and the evolution of market analysis tools.
Integration of Artificial Intelligence
Artificial Intelligence (AI) is dramatically transforming automatic trade systems by enabling more sophisticated algorithms. With advances in machine learning and data analysis, AI can process vast amounts of financial data efficiently. This gives traders an edge by identifying patterns that human traders might overlook.
Key benefits of integrating AI into trading systems include:
- Enhanced Decision Making: AI can analyze market conditions in real time and adjust trading strategies accordingly. This leads to better-informed investment decisions.
- Predictive Analytics: By analyzing historical data, AI algorithms can predict future market movements. This capability enhances the accuracy of trading strategies.
- Risk Management: AI tools can provide risk assessments and identify potential exposure levels automatically.
This integration also raises considerations:
- Data Quality: The effectiveness of AI models heavily relies on the quality of the input data. Poor data can lead to inaccurate predictions and bad investment decisions.
- Ethical Implications: The rise of AI in trading raises ethical questions concerning job displacement and decision-making transparency.
- Security Risks: As systems become more intelligent, they also face heightened cybersecurity threats. Protecting sensitive financial data is paramount.
In summary, the integration of AI promises innovation in automatic trading, but it also necessitates careful consideration of its implications for users and the broader market.
Evolution of Market Analysis Tools
The landscape of market analysis tools is constantly evolving as technology matures. These tools are essential for automatic trading systems as they provide necessary insights and data for informed trading decisions. New advancements in software and analytical methods continue to emerge, catering to changing market needs.
Significant trends in the evolution of market analysis tools include:
- Real-Time Data Processing: Enhanced technology allows for the real-time processing of data from various sources, enabling traders to make quick decisions based on the latest market information.
- Advanced Visualization Techniques: Modern tools offer improved visualization features, making complex data sets easier to interpret. These visualizations facilitate faster understanding and strategic planning.
- Cross-Platform Integrations: Increasingly, market analysis tools are integrating with multiple trading platforms, providing seamless transitions between research and execution phases.
Key considerations include:
- User Experience: The efficacy of market analysis tools depends on accessibility and ease of use. Tools must cater to both experienced traders and beginners.
- Customization Options: Users now seek tools that allow for personalized metrics and analysis specific to their trading styles.
- Subscription Costs: As tools develop, pricing models can vary. Stakeholders need to evaluate cost against the potential benefits gained from using these advanced tools.
"Future-proofing trading operations means embracing advanced tools that fit your decision-making process."
Choosing an Automatic Trade System
The decision to choose an automatic trade system holds significant weight for investors and traders engaged in the complex world of financial markets. This choice can influence not only trading outcomes but also the efficiency of the operations and the overall trading experience. Automatic trade systems can vary widely in their functionalities, approaches, and underlying technologies. Thus, it is crucial for users to approach this decision with a clear understanding of their own needs and the options available.
When considering an automatic trade system, several specific elements become paramount. First, one must evaluate the objectives of trading. Are the goals consistent, or do they fluctuate depending on market conditions? Understanding objectives will inform the selection of a system that aligns with one's trading strategy.
Next, risk tolerance cannot be overlooked. Different systems may employ various levels of risk, which can materially affect the investment outcome. High-frequency trading might yield impressive returns but at greater risk exposure; meanwhile, a more conservative swing trading system could provide steadier returns with fewer surprises.
Another critical aspect to consider is the technology that underpins the chosen automatic trading system. Systems that leverage updated algorithms and data analytics capabilities tend to offer superior performance. One should examine how the system integrates with market data sources to ensure optimal decision-making.
Finally, system support and community feedback play essential roles in making a sound choice. User experiences, both positive and negative, provide insights into potential system performance and reliability. Engaging with users through online forums, such as
Reddit, often reveals these experiences and can guide potential users toward a suitable platform.
Factors to Consider
When selecting a trading system, several factors warrant attention:
- Algorithm Quality: A well-designed algorithm not only analyzes market data but also adapts to changing conditions. A robust system learns over time, which is crucial for maintaining competitive advantages.
- Ease of Use: The platform's interface should be user-friendly. Complex configurations could lead to user mistakes, affecting trading outcomes negatively.
- Costs and Fees: Understanding the fee structures involved with the system is important. Subscription fees, commissions, and any other costs should be transparently communicated by the provider.
- Regulatory Compliance: Ensure that the system adheres to applicable regulations within your jurisdiction, minimizing legal risks.
In essence, thorough research and a thoughtful evaluation of these factors can help in making an informed selection.
Top Automatic Trading Platforms
There are several automatic trading platforms that stand out in the current market:
- MetaTrader 4: Widely known for its ease of use and extensive community support, making it a top choice for many traders.
- NinjaTrader: This platform provides advanced charting capabilities and is favored by many algorithmic traders.
- Zerodha: Known in India for its low brokerage charges, it provides a simple interface for projects on algorithmic trading.
- TradeStation: Offers powerful trading tools and a solid array of features suitable for more advanced traders.
When evaluating these platforms, consider testing their demo accounts before the actual trading begins. This allows you to gauge the systems effectively without incurring financial risk. Utilizing community insights and systematic testing enhances your chances of successful trading in an automatic experience.
The End
The conclusion of this article underscores the significance of automation in trading systems, providing a thoughtful synthesis of the insights gained throughout our discussion. As financial markets evolve rapidly, understanding automatic trade systems becomes crucial for professionals involved in trading, software development, and market analysis. The importance lies not only in recognizing the mechanics but also in comprehending the broader implications these systems have on investing strategies and market trends.
Summary of Key Insights
The exploration of automatic trading systems reveals several key insights that define their role in modern finance:
- Efficiency in Execution: Automatic trading systems enable rapid execution of trades, far surpassing human capabilities in terms of speed. This capacity is paramount in capitalizing on fleeting market opportunities.
- Data-Driven Decision Making: These systems rely on robust data analysis, drawing from diverse sources to inform trading strategies. The integration of advanced algorithms enhances the predictive accuracy significantly.
- Risk Management: Users of automatic trading systems can implement sophisticated risk management strategies that help mitigate potential losses. This aspect is essential in today's volatile market environments.
- Regulatory and Ethical Considerations: As automatic trading continues to grow, understanding the regulatory landscape becomes vital. Compliance with regulations ensures that systems operate within legal frameworks, preserving market integrity.
Overall, these insights highlight the transformative impact that automatic trade systems have on the trading landscape and the necessity of adapting to these changes.
The Future of Trading Automation
Looking toward the future, trading automation is set to undergo profound changes that will reshape its functionalities and usability. Key trends to watch include:
- AI Integration: As artificial intelligence technologies advance, expect significant enhancements in predictive analytics and decision-making capabilities within trading systems. Machine learning algorithms will likely become central to refining trading strategies, enabling systems to learn from past performance dynamically.
- Increased Personalization: The future may see more personalized trading solutions that cater to individual trader preferences and risk profiles. Tailoured strategies could enhance user engagement and satisfaction.
- Expansion into New Markets: Automation will not be limited to traditional financial markets. Emerging sectors, such as cryptocurrency and foreign exchange, will likely see more sophisticated automated trading tools in use.
In summary, automatic trade systems represent a pivotal element of the financial trading ecosystem. As technology advances, their influence will only grow, making it essential for investors and professionals alike to stay informed and adaptable.