SAS vs SPSS: Features, Applications, and Key Differences
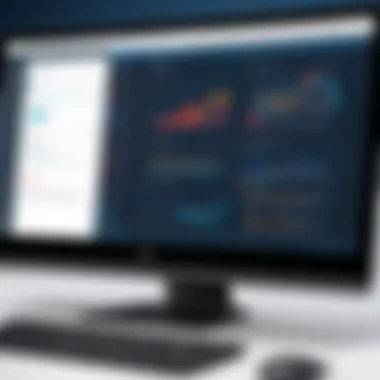
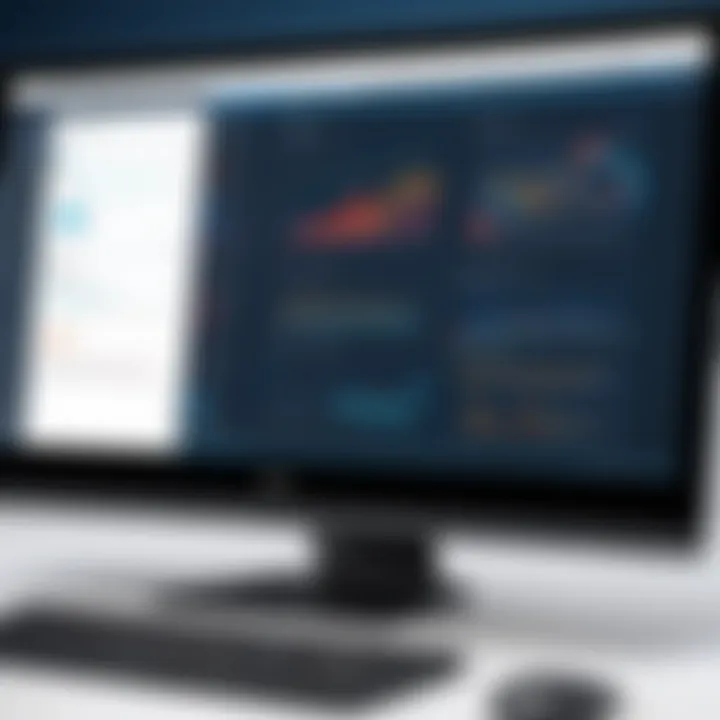
Intro
In the arena of statistical analysis and data management, SAS and SPSS stand out as prominent software solutions. Their applications span across various industries, facilitating decision-making processes through data-driven insights. Understanding the intricacies of these platforms is essential for software developers, IT professionals, and students alike.
This examination delves into the functionalities of SAS and SPSS, shedding light on their respective strengths and weaknesses. By exploring their key features, system requirements, performance, usability, and best use cases, users can discern which software aligns best with their specific analytical needs. As we navigate this exploration, it becomes clear that both SAS and SPSS play pivotal roles in the landscape of data analysis, each catering to different requirements and preferences.
Adopting a comprehensive approach to this discussion will ultimately assist users in making informed decisions, enhancing their data analysis capabilities, and optimizing their workflow.
Software Overview
Key Features
SAS offers a robust environment for data analytics. Its strengths lie in its advanced data manipulation techniques and extensive statistical procedures. Features like predictive analytics, data mining, and statistical analysis are core components of SAS, making it a favored choice for large-scale data operations.
On the other hand, SPSS is renowned for its user-friendly interface, which is particularly attractive to those less familiar with programming. SPSS excels in survey analysis, providing features that simplify complex statistical procedures. Its strong focus on data visualization and easy-to-use tools make it accessible for beginners.
System Requirements
Both SAS and SPSS have specific system requirements to ensure optimal performance.
- For SAS, the requirements depend on the specific version being used, but typical specifications include:
- SPSS requirements also vary with the edition. Common specifications include:
- Windows 10 or a compatible OS
- Minimum 4 GB of RAM (8 GB recommended)
- A multi-core processor for efficient data processing
- 64-bit operating system
- Minimum 4 GB of RAM (8 GB recommended)
- At least 1 GHz processor
Ensuring that your system meets these specifications can prevent issues during data analysis.
In-Depth Analysis
Performance and Usability
When discussing performance, SAS is known for handling large datasets more efficiently than many alternatives. Its capacity to process huge volumes of data without significant lag has made it the go-to tool for big data analytics.
Conversely, SPSS focuses on usability. Its interface simplifies navigation and analysis, which is crucial for rapid insights without extensive programming knowledge. This makes SPSS appealing for researchers in social sciences and academia, where the ability to quickly interpret results is vital.
Best Use Cases
Identifying ideal scenarios for each software enhances decision-making:
- SAS is often used in:
- SPSS is typically favored in:
- Health analytics for patient data analysis
- Financial institutions for risk management and fraud detection
- Marketing analysis to evaluate customer behavior
- Academic research, particularly in psychology and social sciences
- Market research for survey data analysis
- Quality control in manufacturing organizations
SAS and SPSS serve distinct needs within the analytics domain. Choosing the right software hinges on understanding your specific requirements and the nature of your data.
Prolusion to Statistical Software
In todayโs data-driven world, statistical software plays a crucial role in transforming raw data into actionable insights. With a wide range of applications across various industries, these tools are essential for professionals seeking to analyze and interpret data. This article focuses on two leading software solutions: SAS and SPSS. Understanding these tools will help users make informed decisions about which one best fits their needs for statistical analysis and data management.
Statistical software enables users to perform complex data analyses without needing extensive programming knowledge. Their user-friendly interfaces and robust functionalities allow for detailed exploration of data, facilitating everything from simple descriptive statistics to advanced predictive modeling. In this context, we will delve into the specific roles and capabilities of SAS and SPSS, setting the stage for a deeper understanding of their applications and differences.
The Role of Statistical Software
Statistical software serves varied purposes, which include:
- Data Analysis: The primary function of statistical software is to analyze data sets comprehensively. This ensures that users can derive significant insights relevant to their research or business objectives.
- Data Management: Beyond analysis, managing data efficiently is vital. Software like SAS and SPSS offers capabilities for cleaning, organizing, and maintaining data integrity across different formats.
- Reporting and Visualization: Effective communication of findings is essential. These tools often include features for generating reports and creating visualizations, making data more accessible to stakeholders.
The increasing complexity and volume of data necessitate sophisticated statistical tools. Thus, understanding the functionalities and limitations of each software program is essential for anyone engaged in data-intensive fields.
Overview of SAS and SPSS
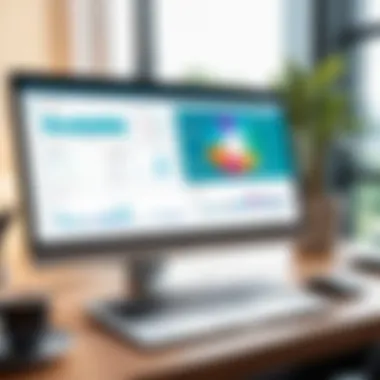
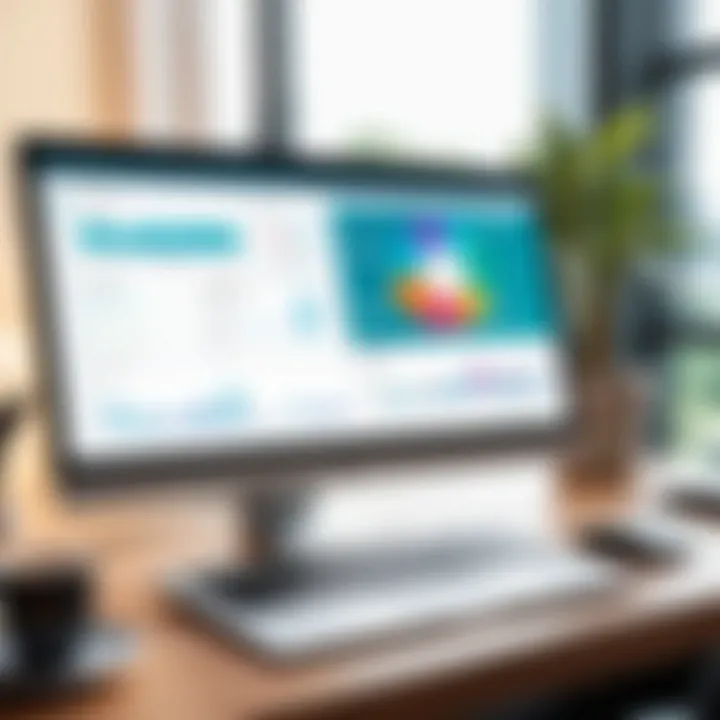
SAS (Statistical Analysis System) and SPSS (Statistical Package for the Social Sciences) are two of the most reputable tools in the domain of statistical analysis. Their features align with different user needs, making it crucial to understand what each offers.
- SAS is known for its powerful data processing capabilities and versatility in handling large data sets. It is commonly used in industries such as healthcare, finance, and retail, where complex data manipulation is essential. The ability to automate tasks within SAS also enhances productivity for its users.
- SPSS is renowned for its user-friendly interface, which appeals to a broader audience, including social scientists and researchers. It specializes in survey analysis and is widely used in academic settings and market research. SPSS provides users with many built-in statistical tests, which makes it approachable for beginners.
Both SAS and SPSS contribute significantly to the fields of data analysis and statistical modeling, yet they cater to slightly different user requirements. Understanding their respective strengths and limitations will guide users in making choices that align with their analytical needs.
What is SAS?
Understanding SAS is crucial for professionals seeking to analyze complex data sets effectively. SAS, or Statistical Analysis System, is a robust software suite developed for advanced analytics, business intelligence, data management, and predictive analytics. The ability of SAS to handle vast amounts of data makes it essential in various industries such as finance, healthcare, and retail. This section will delve into SAS's historical background, core features, and its diverse applications.
Historical Background
SAS was developed in the 1970s at North Carolina State University. It initially aimed to assist agricultural research in analyzing data. Over the years, it has evolved significantly. Now, it serves as a comprehensive tool for statistical analysis across many fields. As businesses began to recognize the value of data, SAS expanded its functionalities to keep pace with growing demands. The strong foothold SAS holds in the market results from its continuous updates and its focus on user needs.
Core Features of SAS
Data Management
Data Management in SAS is one of its most essential features. It allows users to manipulate and cleanse data efficiently. This includes importing, exporting, and transforming data from various sources. A key characteristic of this functionality is its ability to handle large datasets with ease. This makes SAS a popular choice for professionals needing to maintain data integrity over extensive processes. The unique feature of its data step language provides precise control to manipulate data, which is a significant advantage in complex analyses.
Statistical Analysis
Statistical Analysis in SAS extends beyond basic statistics. It includes a wide range of advanced methods such as regression modeling, multivariate analysis, and time series analysis. SAS supports rigorous statistical techniques, which are beneficial to users who demand accuracy. Its unique feature is the vast library of procedures that cater to various statistical needs, which can also be a disadvantage as mastering these procedures requires considerable time and expertise.
Reporting and Visualization
Reporting and visualization capabilities in SAS are designed to present analysis outcomes in a clear format. Users can create detailed reports, dashboards, and graphs. This is especially important in a business context where stakeholders may require visual summaries of data. The key characteristic is that SAS allows for customizable reporting options, which makes it attractive to users looking to tailor their outputs. However, the learning curve for creating complex visualizations might deter some beginners.
Applications of SAS
Banking and Financial Services
In Banking and Financial Services, SAS plays a pivotal role in risk management and fraud detection. Financial institutions use SAS for precise modeling and predictive analytics. A significant aspect of its application in this field is its compliance with stringent financial regulations. Its ability to produce accurate reports and forecasts makes SAS indispensable for financial analysts. However, reliance on SAS requires professional training to utilize its capabilities effectively.
Healthcare
In the Healthcare sector, SAS supports drug development, patient analysis, and operational efficiency. It helps organizations analyze patient data to improve health outcomes. The capacity of SAS to handle various data types from clinical trials or electronic health records is a hallmark feature. However, the high costs associated with SAS licenses could be a barrier for some small healthcare entities.
Retail and Marketing
SAS is also essential in Retail and Marketing, particularly for customer segmentation and demand forecasting. Retailers use SAS to analyze shopping patterns and optimize inventory levels. A key characteristic of SAS in this sector is its ability to integrate customer data from multiple channels. This integration provides retailers valuable insights into consumer behavior. Still, the complexity of SAS may limit its use to more data-savvy professionals in marketing departments.
What is SPSS?
SPSS, short for Statistical Package for the Social Sciences, is a powerful statistical software widely used for data analysis and management. Its importance lies in its ability to provide a comprehensive platform that caters to both novice and advanced users in various fields. This section explains SPSS's role in statistical analysis, touching on its history, core features, applications, and benefits.
Historical Background
SPSS was developed in 1968 by Norman H. Nie, C. Hadlai Hull, and William G. Franklin. Originally aimed at social scientists, it has since evolved into a versatile tool used by professionals across various disciplines. The acquisition by IBM in 2009 further cemented its standing in the industry, enabling integration with other IBM products and expanding its functionalities. The historical evolution provides users insight into its robust development and adaptability in response to changing data needs.
Core Features of SPSS
Data Analysis
Data analysis in SPSS is one of its most significant aspects. The software offers a user-friendly interface that simplifies complex statistical processes. Users can perform various analyses, from descriptive statistics to more intricate inferential tests. A key characteristic is its ability to handle large datasets with ease, which enhances its appeal among professionals. The unique feature of SPSS's data analysis capability is the extensive range of statistical functions available, though some advanced analyses may require additional training or experience to interpret accurately.
Survey Analysis
Survey analysis is another critical function of SPSS. This feature allows users to input, manage, and interpret survey data effectively. SPSS provides specialized tools for transforming raw survey results into actionable insights. The software's interactive capabilities make it a popular choice for researchers. However, users must be meticulous in data entry to avoid errors, as inaccuracies can lead to flawed conclusions.
Reporting Capabilities
SPSS excels in reporting capabilities, enabling users to generate detailed reports based on analyses. Users can create easily interpretable output tables and visualizations. These reports are essential for conveying complex information in a straightforward manner. A key characteristic is the automated syntax generation, which facilitates reproducibility. Yet, while SPSS is effective in producing professional reports, users need to understand the data context to create meaningful visualizations.
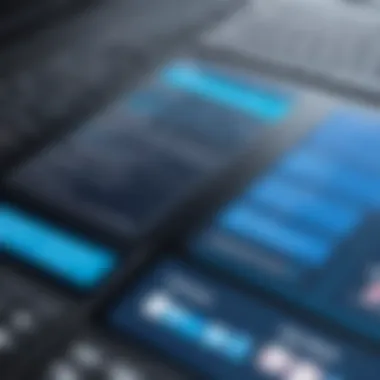
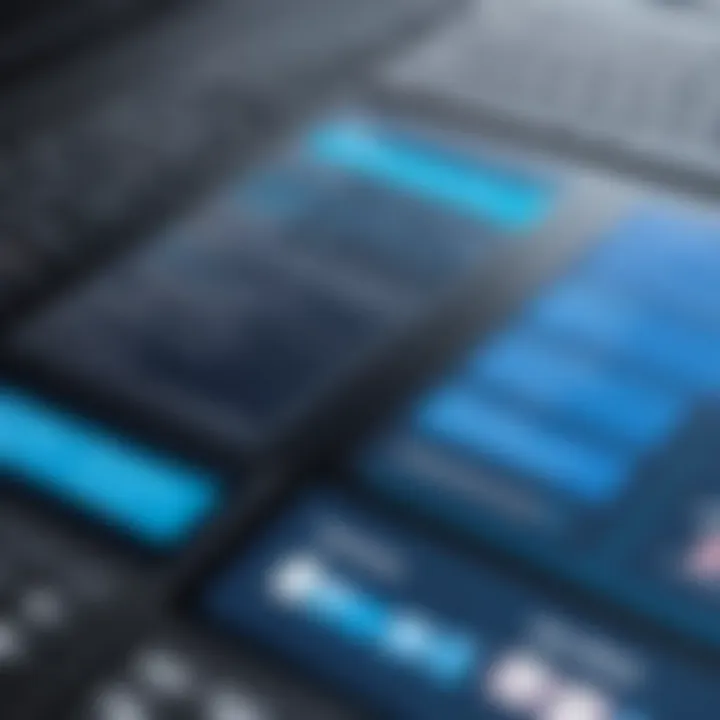
Applications of SPSS
Academia
In academia, SPSS is widely used for research and teaching purposes. Its ease of use makes it suitable for students and educators alike. The software supports a broad range of statistical tests necessary for academic research. Students can gain hands-on experience, preparing them for careers in data analysis. However, reliance solely on SPSS may limit exposure to other analytical tools, which can be a drawback for aspiring professionals.
Market Research
SPSS finds significant application in market research, where data-driven insights are crucial. Businesses utilize SPSS to analyze consumer behavior, preferences, and trends. Its features allow for in-depth segmentation and profiling, providing a competitive edge. Market analysts benefit from the softwareโs capabilities but should be aware of the rising need for integrating other data sources to create a comprehensive view of the market landscape.
Government Agencies
Government agencies leverage SPSS for data evaluation and policymaking. The software aids in analyzing census data, survey results, and various statistical reports. Its ability to handle complex datasets makes it an excellent choice for public sector projects. However, reliance on software alone must be balanced with statistical literacy among users to ensure accurate interpretations of results.
"SPSS empowers users by simplifying complex statistical tasks, making it accessible for various fields, from education to government."
Comparative Analysis of SAS and SPSS
Understanding the comparative analysis between SAS and SPSS is essential for users who seek to select the right tool for their data analysis needs. Each software has unique characteristics that can cater to different requirements in various workflows. This section aims to highlight significant elements that are crucial when comparing these two powerful statistical tools. The benefits and considerations surrounding user experience, statistical techniques, and overall flexibility are integral to informed decision-making.
User Interface Considerations
The user interface of any statistical software can greatly influence how efficiently users perform their analyses. SAS offers a more complex environment, which might be overwhelming for new users. It relies heavily on programming and scripting, making it better suited for experienced users who are comfortable with coding language. In contrast, SPSS provides a more intuitive graphical user interface, emphasizing point-and-click functionality. This design is particularly appealing to beginners or those less familiar with programming.
When evaluating user interfaces, you should consider the following:
- Learning Curve: SAS requires a steeper learning curve due to its reliance on coding. SPSS allows users to dive into analysis more quickly.
- Functionality Access: SAS offers a rich set of functionalities that can take time to learn, while SPSS allows for easier navigation through its functions.
Statistical Techniques Offered
Both SAS and SPSS provide a wide range of statistical techniques but differ in their focus and application. SAS is known for advanced statistical procedures, including predictive modeling and complex data manipulation. It encompasses functionalities that support vast data sets and intricate analyses, making it ideal for industries like finance and health care.
SPSS, on the other hand, is known for its strength in survey analysis and descriptive statistics. It provides an accessible approach to performing basic statistical tests with a straightforward methodology. Here are some techniques offered by each software:
- SAS: Regression analysis, time series analysis, and data mining.
- SPSS: T-tests, ANOVA, and factor analysis.
Understanding the specific statistical needs of your project will help guide this choice.
Customizability and Flexibility
Another critical aspect of the comparative analysis of SAS and SPSS is their customizability and flexibility. SAS excels in custom programming and automation of repetitive tasks. Users can easily develop customized scripts to meet specific analysis needs. This flexibility is vital for professional users who handle complex data scenarios regularly.
In contrast, while SPSS allows for some level of customization, it does not match the extent available in SAS. SPSS's focus remains on facilitating standard statistical analyses with less emphasis on programming. For users needing targeted analyses within a defined scope, SPSS offers sufficient flexibility without the need for extensive programming.
Thus, the choice between these two will often hinge on your familiarity with coding, the complexity of analyses required, and your specific application needs.
Strengths and Weaknesses
Understanding the strengths and weaknesses of SAS and SPSS is essential for users deciding which software to utilize for data analysis. Each platform possesses unique characteristics that cater to different needs and preferences. Evaluating these attributes helps users identify the best fit based on their specific projects, skills, and organizational requirements. From capabilities in advanced analytics to usability for beginners, the comparison between these two tools offers critical insight into their potential impact on any analytical endeavor.
Advantages of SAS
SAS has several strengths that make it a popular choice among data professionals.
- Comprehensive Data Management: SAS excels in data management capabilities, allowing users to efficiently manipulate and process massive datasets. This is particularly useful in industries like healthcare and finance where data is often voluminous and complex.
- Advanced Analytical Techniques: The software includes a wide range of statistical techniques, including predictive modeling, advanced analytics, and machine learning algorithms. Its robustness in these areas is a major advantage for users requiring deeper insights from their data.
- Customization Options: SAS is configurable, meaning users can tailor it to their specific needs. This flexibility supports the creation of custom scripts or applications, providing a higher degree of control over analyses and outputs.
- Scalability: SAS products can scale effectively to accommodate growing data sets and increasing analytical demands. This scalability makes it suitable for both small projects and large enterprise applications.
Disadvantages of SAS
Despite its strengths, SAS does have notable weaknesses.
- Cost: SAS licenses can be significantly expensive, making it less accessible for smaller organizations or individual researchers. Ongoing costs for maintenance and support further add to this burden.
- Steeper Learning Curve: New users may find SAS challenging to learn, especially if they do not have prior programming experience. Its use of proprietary syntax can be a barrier to entry.
- Limited Community Support: Compared to SPSS, SAS has a smaller community of users. This can make finding resources, tutorials, and support more difficult for those seeking help in unfamiliar areas.
Advantages of SPSS
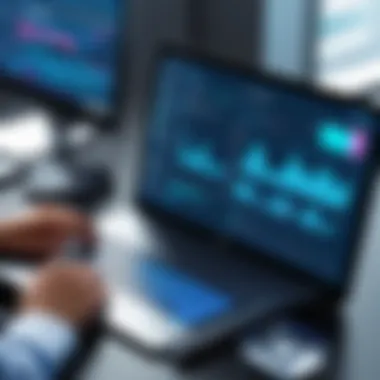
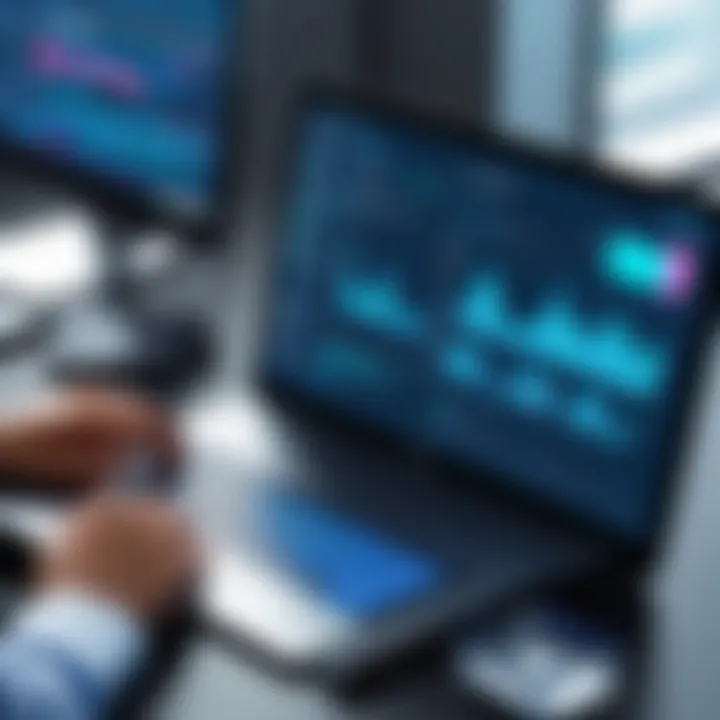
SPSS offers several advantages that appeal to a diverse user base, especially in academic and research settings.
- User-Friendly Interface: SPSS is well-known for its intuitive graphical user interface. This makes it accessible to beginners and non-technical users, allowing them to perform statistical analyses without deep programming knowledge.
- Strong Survey Analysis Capabilities: SPSS provides robust features for survey analysis, which is particularly valuable for market researchers and social scientists. Its tools for dealing with categorical data and complex survey designs are very helpful.
- Effective Reporting Tools: SPSS has effective reporting options that allow users to generate clear and comprehensive outputs. The built-in visualization features facilitate the communication of findings in a user-friendly manner.
- Active User Community: The SPSS user community is large and active. This results in a wealth of shared knowledge, resources, and forums, creating opportunities for users to engage and learn from one another.
Disadvantages of SPSS
However, SPSS also has its drawbacks that potential users should consider.
- Limited Advanced Analytical Techniques: SPSS may not offer the same depth of advanced analytics compared to SAS. Users looking for complex predictive modeling or tailored machine learning capabilities might find its options limiting.
- Customization Limitations: While SPSS allows for some extent of customization, it does not match SAS's flexibility. Users are often confined to the pre-built functionalities of the software, which can be restrictive for more advanced needs.
- Cost Considerations: Similar to SAS, SPSS is also expensive. Although it might be more accessible than SAS for some users, licensing can still pose significant challenges, especially in budget-conscious environments.
In summary, both SAS and SPSS bring valuable strengths and weaknesses to the table. Users must evaluate these aspects according to their unique requirements to make informed decisions regarding statistical software.
Choosing the Right Tool
Selecting between SAS and SPSS can significantly impact your data analysis journey. Understanding the key characteristics of each software is vital for effective decision-making. The right tool depends on various factors, such as the userโs experience level, specific project needs, and industry requirements. Having a clear view of these elements can help in maximizing efficiency and achieving desired outcomes in data analysis.
Considerations for Beginners
For individuals new to statistical software, the choice can be overwhelming. Beginners should prioritize ease of use when selecting a tool. SPSS is often viewed as more user-friendly due to its intuitive interface and menu-driven commands, making it a suitable option for those with limited statistical knowledge.
In contrast, SAS offers robust capabilities but may impose a steeper learning curve. However, that should not deter beginners from considering SAS if they have access to ample training resources. Additionally, learners should explore training materials and community forums. Online platforms such as Reddit and academic websites can provide valuable insights and guidance.
Some key factors for beginners include:
- Availability of training resources: Training courses, online tutorials, and user communities can facilitate learning.
- User support: Look for platforms that offer strong technical support and documentation.
- Software demos: Engaging with trial versions can help assess user experience without committing to a purchase.
Considerations for Advanced Users
Advanced users tend to look beyond the basics of ease and usability. They should consider the specific analytical needs of their projects when choosing between SAS and SPSS. For complex data analysis, SAS may be preferred due to its powerful statistical capabilities and comprehensive suite of features. It supports extensive programming options, which allows for custom procedures and extensive data manipulation.
On the other hand, SPSS is advantageous for its focus on survey data and social science applications. Users engaged in market research or academia might find its features beneficial for statistical testing and reporting.
For advanced users, important considerations may include:
- Compatibility with existing systems: Ensure that the chosen software integrates seamlessly with other tools in use.
- Customization capabilities: Advanced users might need tailored solutions to varying analytical needs.
- Cost-efficiency: Weigh the subscription costs against the advantages provided to determine the best fit.
In summary, selecting the right software tool hinges on understanding oneโs own proficiency level, the analytical demands of the project, and long-term goals in data management. Users of all levels must carefully consider these factors to choose the solution that aligns best with their needs.
Future Trends in Statistical Software
The realm of statistical software is undergoing significant transformations driven by technological advancements and evolving user needs. Understanding these trends is crucial for software developers, IT professionals, and students who want to stay ahead in data analysis and management. The importance of the future trends in statistical software lies in their potential to redefine how data is processed, analyzed, and interpreted.
Emerging Technologies
Emerging technologies are reshaping the landscape of statistical software. Data science is becoming increasingly reliant on machine learning algorithms and artificial intelligence models. These technologies enhance the capabilities of software like SAS and SPSS, allowing for more sophisticated analysis and predictive modeling.
Several noteworthy trends include the rise of cloud computing. Software tools are now often accessible via the cloud. This change has improved data sharing and collaboration among users. Users can access large datasets and leverage computational power without investing heavily in hardware. Additionally, the integration of automated machine learning is streamlining processes that require extensive data preprocessing or model selection. Tools are evolving to include features that automate routine tasks, allowing professionals to focus on interpreting results rather than just data preparation.
According to experts, the implementation of natural language processing is another exciting area. This feature will allow users to interact with software using everyday language. It simplifies the analysis process for beginners and makes data insights more accessible to a broader audience.
Integration with Big Data
The integration of statistical software with big data is another future trend. As organizations increasingly rely on large datasets, the ability to handle big data efficiently becomes essential. Statistical software must adapt to these changes, facilitating easier data access and processing.
SAS has developed features to handle vast amounts of data through advanced analytics tools. Its data management capabilities allow it to work seamlessly with large databases, making it suitable for industries like finance and healthcare. SPSS, though not initially designed for big data, is incorporating features to manage larger datasets effectively through integration with other tools and databases.
The use of technologies such as Hadoop and Apache Spark plays a significant role in this integration. Hadoop facilitates storage and processing of big data across distributed computing networks. Apache Spark, on the other hand, enhances data processing speeds significantly. The combination of these technologies with statistical software opens new avenues for real-time analysis and insight generation, which is critical for businesses looking for competitive advantages.
Culmination
In summarizing the comprehensive examination of SAS and SPSS software, it is essential to understand their significance in the realm of statistical analysis and data management. Both tools, while different in their approaches and applications, offer critical functionalities that cater to varying user needs. As professionals and students navigate the field of data analytics, recognizing when to use SAS or SPSS can lead to more effective data-driven decision-making.
Summarizing Key Insights
The exploration of SAS and SPSS reveals several key insights:
- Diverse Applications: SAS excels in fields such as healthcare and finance, where complex data management and sophisticated analytics are vital. On the other hand, SPSS is favored in academia and market research for its ease of use and robust survey analysis capabilities.
- Strengths and Weaknesses: Each software has unique strengths; SAS is known for its advanced statistical techniques and customization options, while SPSS is recognized for its user-friendly interface and reporting capabilities. However, both also have their drawbacks, such as cost and learning curves that vary significantly.
- User Considerations: The choice between SAS and SPSS should consider the userโs skill level, specific data analysis needs, and industry requirements. Beginners might find SPSS more approachable, while advanced users may appreciate the depth of SAS.
Ultimately, your choice will impact the quality of data insights derived from these powerful tools. The future trends discussed earlier, particularly in emerging technologies and integration with big data, highlight the necessity for ongoing education and adaptability in using these software solutions. As technology continues to evolve, staying informed on enhancements in both SAS and SPSS will empower users to harness the full potential of their data analysis capabilities.