Unveiling Top Embedded Analytics Tools for Businesses
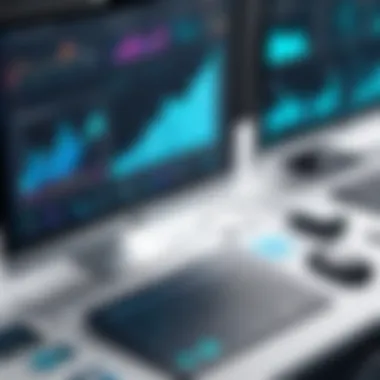
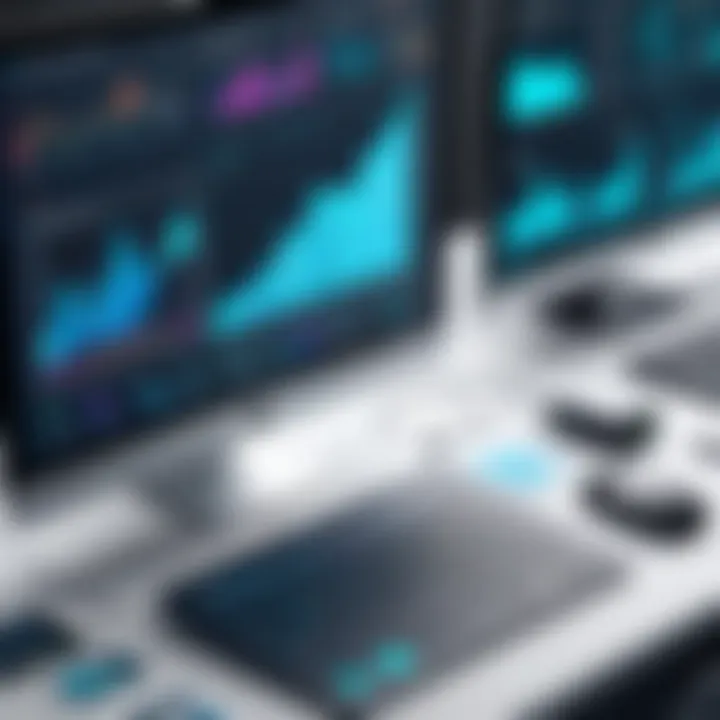
Intro
Embedded analytics tools serve a critical function in today’s data-driven organizations. They allow businesses to integrate analytical capabilities directly into applications without needing users to switch to separate data analysis platforms. This enables seamless data exploration and visualization, helping users make informed decisions quicker.
Understanding the landscape of embedded analytics tools is essential for both software developers and professionals in IT-related fields. This exploration will provide detailed insights into key features, usability, and specific use cases for these tools, which can elevate decision-making and operational efficiency in various settings.
Software Overview
Embedded analytics tools come with a variety of functionalities designed to enhance data accessibility and insight generation. The effectiveness of these solutions can be evaluated through various key features, followed by a look at system requirements necessary for optimal performance.
Key Features
Some of the principal features that define the best embedded analytics tools include:
- Data Visualization: Effective tools offer rich visualization options to represent data clearly. Users can create dashboards, charts, and graphs that transform raw data into understandable insights.
- Integration Capabilities: The ability to easily integrate with existing software systems is a critical feature. This allows data to be pulled directly from various sources, making analytics more fluid and accessible.
- Customizability: Users should have the flexibility to tailor the analytics experience according to their requirements. Custom dashboards and user interfaces can significantly enhance user engagement.
- Real-time Analytics: Timeliness is crucial in data analysis, making real-time data processing a must-have. This allows users to react quickly to trends or issues as they arise.
System Requirements
When selecting an embedded analytics tool, understanding its system requirements is vital. Common requirements usually include:
- A robust data source, which could be a SQL database, NoSQL database, or even cloud storage solutions.
- Sufficient hardware capabilities, particularly for on-premise solutions, to handle data processing loads adequately.
- Compatibility with existing APIs and technologies to facilitate smooth integration.
- Support for various file formats and data types to ensure versatility in data handling.
In-Depth Analysis
A thorough understanding of the performance and usability of embedded analytics tools, along with best use cases, will further illuminate their value.
Performance and Usability
Performance metrics for embedded analytics tools often focus on speed and reliability. Users benefit from platforms that maintain fast load times without compromising data accuracy. Usability is also critical; a well-designed interface should facilitate easy navigation and allow users to derive insights with minimal learning curve.
Best Use Cases
The application of embedded analytics tools varies across industries and functions, including:
- Business Intelligence: Organizations utilize these tools for deeper market analysis, competitive intelligence, and strategic planning.
- Customer Experience: Businesses improve customer interactions through data analysis, identifying trends to tailor services accordingly.
- Financial Analysis: Financial institutions use embedded analytics for real-time insights on transaction data, credit scoring, and risk assessment.
"Embedded analytics can transform the way organizations approach data by integrating insights directly into business workflows, thus facilitating quicker decision-making processes."
Ultimately, the exploration of embedded analytics tools presents a compelling case for their incorporation in modern data strategies. Understanding their key features, system requirements, performance, and usability is essential to harness their full potential.
Prologue to Embedded Analytics
Embedded analytics represent a significant advancement in how organizations process and analyze data. By integrating analytical capabilities directly into applications, businesses can empower users to make informed decisions without needing to switch between different platforms. This seamless integration not only enhances efficiency but also improves the overall user experience.
Defining Embedded Analytics
Embedded analytics refers to the integration of data analysis capabilities into an application or software. This allows users to access insights without needing separate analytics tools. The core components include data visualization, reporting tools, and dashboards that display real-time data—all embedded within the user interface of the host application.
In essence, embedded analytics transforms raw data into actionable insights. Users can gain a deeper understanding of their data contextually, which in turn leads to better decision-making.
Importance in Modern Business
The role of embedded analytics in today’s business landscape is increasingly critical. Companies are inundated with data, making it imperative to derive meaningful insights efficiently.
- Enhanced Decision-Making: Embedded analytics allows decision-makers to analyze data as it happens. This immediacy ensures that businesses can respond to trends and issues in real-time.
- Increased Data Utilization: Many organizations struggle to leverage their data fully. By embedding analytics within existing applications, employees are more likely to engage with and utilize data.
- Cost-Effective Solutions: Developing stand-alone analytical tools can be expensive and time-consuming. Embedded analytics offer a cost-effective alternative by utilizing existing platforms and infrastructure.
- Fostering a Data-Driven Culture: Organizations that adopt embedded analytics tend to cultivate a culture that values data-driven decision-making. Employees at all levels become more adept at interpreting data, which enhances overall productivity and innovation.
"Embedded analytics empower users by providing immediate insights, fostering an organizational culture that values data-driven decisions."
Key Features of Embedded Analytics Tools
Embedded analytics tools have become essential in modern data-driven businesses. They enable organizations to integrate advanced analytical capabilities into their applications and workflows. This section will provide a detailed discussion of the critical features that determine the effectiveness of these tools. Understanding these features can help software developers and IT professionals make informed choices when selecting the right embedded analytics solution for their needs.
Data Visualization Capabilities
Data visualization is a fundamental aspect of embedded analytics tools. Users depend on clear and informative visual representations of complex data sets to make quick decisions. Effective data visualization capabilities allow users to explore data through interactive charts, graphs, and dashboards. The right tool will provide customizable visual options to cater to different data types and user preferences.
Important aspects of data visualization include:
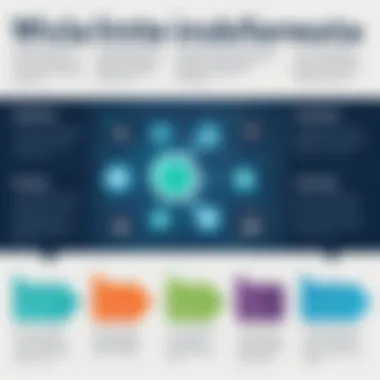
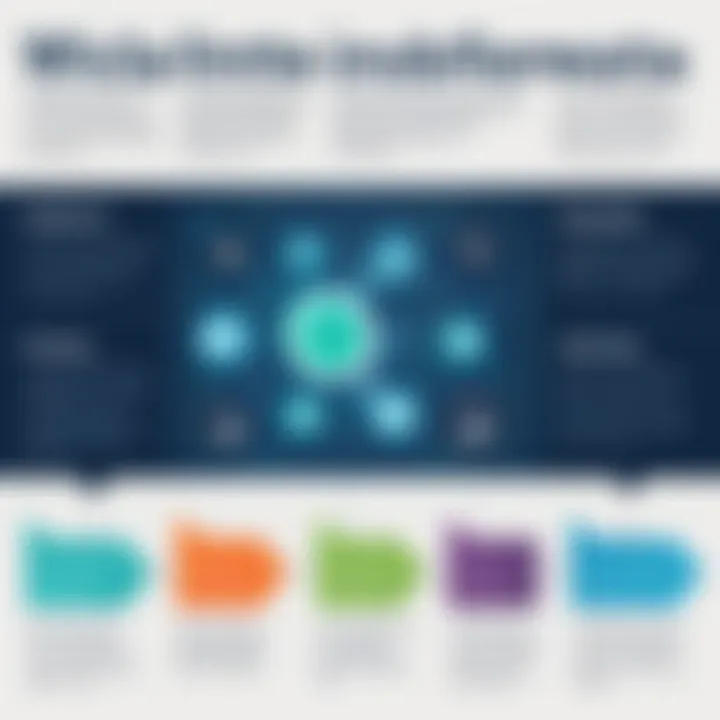
- Interactivity: Users should be able to manipulate data views for deeper insights.
- Customization: Users must tailor visualizations to align with their business context or branding.
- Clarity: Visuals should convey information succinctly without overwhelming the user.
Integration with Existing Systems
For embedded analytics tools to function effectively, they must integrate seamlessly with existing systems and data sources. This integration ensures that users can access real-time data across different platforms without encountering barriers. An effective embedded analytics solution will support integration with various data storage like databases, cloud services, or business applications. Considerations for integration include:
- API Accessibility: The tool should offer well-documented APIs for smooth integration.
- Compatibility: Ensure the analytics tools can connect with current data architectures.
- Data Synchronization: Real-time data updates are critical for accurate decision-making.
User Experience and Interface Design
An intuitive user experience is important for any embedded analytics tool. Professionals in the IT field or software development expect tools that are easy to use to minimize in-house training requirements. A well-designed interface can provide users with faster access to the information they need, improving overall productivity.
Key elements of user experience include:
- Navigation Ease: Navigation should be straightforward, allowing users to find relevant insights quickly.
- Responsive Design: The interface should be functional across various devices, from desktops to mobile.
- User Support: Adequate help resources or tutorials ensure users don’t get stuck while using the tools.
Real-Time Data Processing
The ability to process data in real-time is a game-changer for embedded analytics. Organizations strive for up-to-date insights to make timely decisions, especially in fast-paced industries. Real-time processing allows for immediate data updates and responsiveness to changing conditions or trends.
Key features to look for include:
- Streaming Data: Support for ingesting streaming data can enhance the relevance of insights.
- Alert Systems: Automated alerts can notify users when data meets specific conditions, prompting quick action.
- Scalability: Ensure the tool can handle increased data volumes without affecting performance.
The effectiveness of embedded analytics tools is determined significantly by their core features. Choosing tools with strong data visualization, integration capabilities, user-friendly designs, and real-time processing can yield substantial benefits for any organization.
In summary, the key features of embedded analytics tools play a crucial role in their effectiveness. Data visualization capabilities, seamless integration with existing systems, user experience, and real-time data processing are vital to enhance decision-making. By focusing on these aspects, developers and IT professionals can select tools that align with their specific business needs.
Top Embedded Analytics Tools Overview
Embedded analytics tools have become essential for businesses aiming to make informed decisions based on their data. This section explores notable tools that stand out in the current market. It highlights their features, capabilities, and how they fit different business needs. Understanding these tools goes beyond just knowing their names; it involves recognizing their specific functionalities that enhance user experiences and aid in decision-making.
These tools enable businesses to integrate analytics directly into their applications. By doing so, they allow users to gain insights without switching to separate systems. This practice improves workflows, enhances data visibility, and fosters a data-driven culture within organizations.
When considering the right tool for a specific business, various aspects are crucial. This includes data visualization capabilities, integration with existing systems, and user experience. Each embedded analytics tool comes with unique strengths that can either align or misalign with an organization's needs.
"The right embedded analytics tool can significantly transform how a business interacts with its data, ultimately driving better outcomes."
Tool A: Overview and Features
Tool A, known as Tableau, is recognized for its robust data visualization capabilities. Its user-friendly interface allows professionals to create visually compelling dashboards with ease. Tableau supports numerous data sources, making integration simple and efficient for users in various industries. The ability to connect real-time data feeds is one of its most important features. This allows businesses to respond to changes promptly, leading to better decision-making. Moreover, Tableau’s community support provides additional resources for users seeking to enhance their analytical skills.
Tool B: Overview and Features
Power BI is another powerful embedded analytics solution. It excels in integration capabilities with Microsoft products. Its seamless synchronization with tools like Excel and Azure allows users to analyze data where they are already working. Power BI’s strong reporting features provide interactive visualizations that cater to diverse user levels, from beginner to advanced analysts. Additionally, its mobile compatibility ensures that teams can access their reports from anywhere, which is increasingly vital in today's remote work environment.
Tool C: Overview and Features
Looker offers a unique approach to embedded analytics by prioritizing data exploration and customization. With its flexible data modeling capabilities, Looker allows businesses to define metrics that are relevant to their specific needs. This flexibility promotes deeper data analysis and encourages user engagement. Looker is ideal for organizations looking to create tailored reporting solutions. Furthermore, its application programming interface (API) capabilities enhance the ability to embed analytics directly into other applications, thereby providing seamless experiences for users.
In summary, the landscape of embedded analytics tools like Tableau, Power BI, and Looker showcases a variety of approaches to data analysis and visualization. Each tool provides distinct advantages, making it crucial for organizations to assess their specific requirements before selecting a tool that best aligns with their strategies.
Comparison of Leading Embedded Analytics Tools
In the realm of embedded analytics, choosing the right tool can significantly influence how effectively a business uses data. The comparison of leading embedded analytics tools provides insights into what each option can bring to the table, helping professionals make informed decisions. When businesses integrate these tools, they enhance their overall data-driven strategies and facilitate better decision-making.
Understanding the differences between tools is essential as they each have unique features which may cater to different needs. This section highlights key elements such as functionality, integration capabilities, and pricing structures that one must consider. These comparisons are not merely academic; they have practical implications for IT departments, project managers, and end-users alike.
Functionality Comparison
When assessing functionality, it is crucial to look at the specific features that each embedded analytics tool offers. Some tools provide robust data visualization options, while others may excel in statistical analysis. Key aspects to compare include:
- Data Visualization Tools: Some platforms like Tableau and Power BI offer extensive libraries of charts and graphs. These enhance user engagement with intuitive interfaces.
- Reporting and Dashboards: Tools such as Looker and QlikView present customizable dashboards that can be tailored to various departmental needs.
- Data Processing Capacities: Evaluate how well each tool handles large datasets and real-time processing. Tools that can handle vast amounts of data without compromising speed are often more desirable.
- User-Friendliness: An accessible and clean user interface can drastically increase adoption rates within teams. Tools like Domo focus on ease of use to encourage widespread engagement.
Integration Capabilities Comparison
Integration capabilities hold significant weight when evaluating embedded analytics tools. Businesses frequently operate within diverse technology ecosystems, which necessitates seamless interoperability. Important points to consider include:
- APIs and SDKs: Many leading tools offer Application Programming Interfaces (APIs) and Software Development Kits (SDKs) to facilitate integration with existing software. For instance, Sisense provides flexible APIs for deeper customization.
- Data Source Compatibility: The ability to connect with multiple data sources—like databases, cloud services, or third-party applications—expands a tool's usability. Tools that support popular data services like Salesforce or AWS typically receive higher evaluations.
- Real-Time Data Access: Users should consider how well a tool integrates with real-time data inputs. For instance, an analytics tool that continually refreshes data displays ensures users are always working with the latest information.
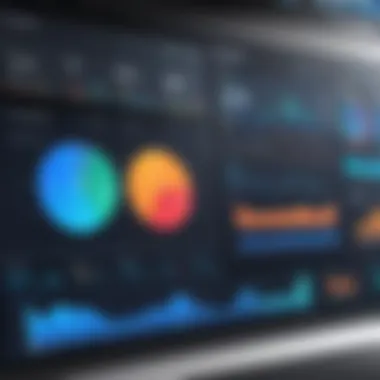
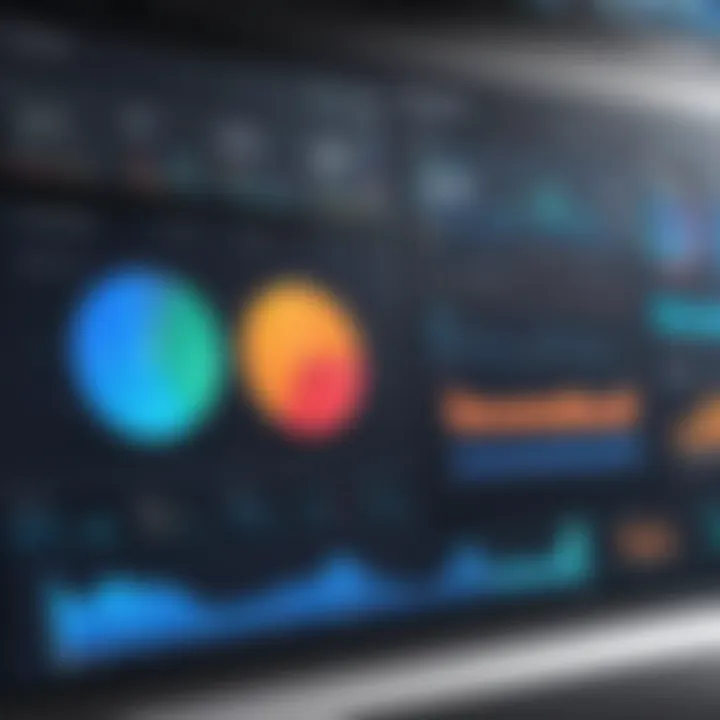
Effective integration enhances a team's capability to combine analytics with current operational processes, thus making the transition smoother.
Pricing Structures Analysis
An often overlooked aspect is the pricing structure of embedded analytics tools. Pricing varies widely based on features, user limits, and additional needs. Here are essential factors to examine:
- Subscription Models: Some tools operate on a subscription basis, catering to small teams while providing scalable options for larger enterprises. For example, Google Data Studio is free, while others, like Microsoft Power BI, have tiered pricing.
- Customization Costs: Some tools charge extra for custom features or additional service upgrades. Understanding these potential costs upfront can prevent budget overruns.
- Training and Support Fees: Effective onboarding and technical support are vital for successful implementation. Tools that include customer support often justify higher price tags.
- Total Cost of Ownership: It's key to consider not just the initial costs but ongoing expenses such as licensing renewals or additional features. Conducting a thorough analysis of total costs helps inform better budgeting decisions.
A well-rounded comparison of pricing structures provides transparency for procurement decisions, ultimately enabling organizations to choose tools that align with their financial capabilities while delivering optimal value.
"The right analytics tool is more than just a cost; it's an investment in your organization's data strategy."
Conducting a careful comparison across functionality, integration, and pricing ensures that the selected embedded analytics tool will align with both immediate needs and long-term goals.
Use Cases for Embedded Analytics
Embedded analytics tools deliver significant benefits across diverse industries. By integrating analytics directly into business applications, organizations enhance decision-making capabilities, leveraging data to drive operational efficiency and provide valuable insights. Understanding the specific use cases for these tools is crucial. This section will outline key areas where embedded analytics proves beneficial while providing considerations for implementation.
Business Intelligence Applications
One of the primary use cases for embedded analytics is within business intelligence applications. Organizations are increasingly reliant on data-driven decisions. Embedded analytics enables businesses to visualize performance metrics and key performance indicators within the very tools employees use daily.
Consider a company utilizing platforms like Tableau or Power BI. When analytics become part of these systems, teams can easily access real-time data without switching applications. This internalization simplifies the decision-making process. Detailed dashboards provide clarity on business trends, customer preferences, and market shifts.
Specific benefits include:
- Informed Decisions: Easy access to data helps regulators and managers make quicker, more informed choices.
- Increased Engagement: Users become more engaged with data when it is relevant to their role and work context.
Thus, integrated analytics should be a priority for organizations aiming to optimize their business intelligence strategies.
Operational Efficiency Monitoring
Embedded analytics also plays a critical role in operational efficiency monitoring. In industries where efficiency is paramount, organizations use analytics to gain insights into processes, workflows, and resource utilization. This function helps identify bottlenecks or inefficiencies in operations.
Tools like Microsoft Dynamics or Salesforce provide integrated analytics capabilities that allow users to monitor key operational metrics in real time. For example, a manufacturing company can assess production line efficiency and make adjustments based on live data.
The advantages of this approach include:
- Timely Interventions: Real-time monitoring allows for immediate action on identified issues, minimizing downtime.
- Resource Allocation: Organizations can more effectively allocate resources based on empirical data, reducing waste and improving productivity.
In this case, embedded analytics tackles operational challenges head-on, fostering a more responsive and efficient organization.
Customer Insights and Engagement
Lastly, embedded analytics greatly enhances customer insights and engagement. Companies can analyze customer behavior, preferences, and interactions within their platforms. This integration enables a more personalized experience, critical in today’s competitive landscape.
For instance, tools such as Google Data Studio can be embedded into customer relationship management systems to track customer engagement metrics and provide actionable insights. Understanding customers allows organizations to tailor products and services effectively.
Key points to consider include:
- Targeted Marketing: Analyzing customer data leads to more targeted marketing efforts and improved service offerings.
- Enhanced Customer Experience: Companies that understand their customers can provide a better overall experience, resulting in higher retention and satisfaction rates.
Challenges in Implementing Embedded Analytics
Implementing embedded analytics presents various challenges that organizations must navigate. These obstacles can hinder effective deployment and utilization, making it crucial to address them thoughtfully. Recognizing these challenges allows businesses to strategize and build solutions that enhance decision-making and data-driven processes. This section looks at the critical elements related to technical barriers, integration concerns, and user adoption.
Technical Barriers and Integration Issues
Technical barriers can arise when integrating embedded analytics tools with existing systems. Many organizations have legacy systems that may not be compatible with the latest analytics software. This leads to difficulties in data integration and may require significant resource investment to update or replace outdated technologies.
Additionally, data silos can complicate how information flows within an organization. When data resides in separate locations or databases, it becomes challenging to aggregate, analyze, and visualize it effectively. Without seamless integration, the potential benefits of embedded analytics are compromised.
Strategies to mitigate these technical issues include:
- Conducting a thorough assessment of existing infrastructure
- Identifying integration points early in the project
- Leveraging APIs and middleware solutions for smoother transitions
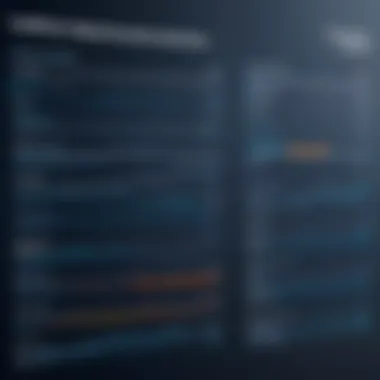
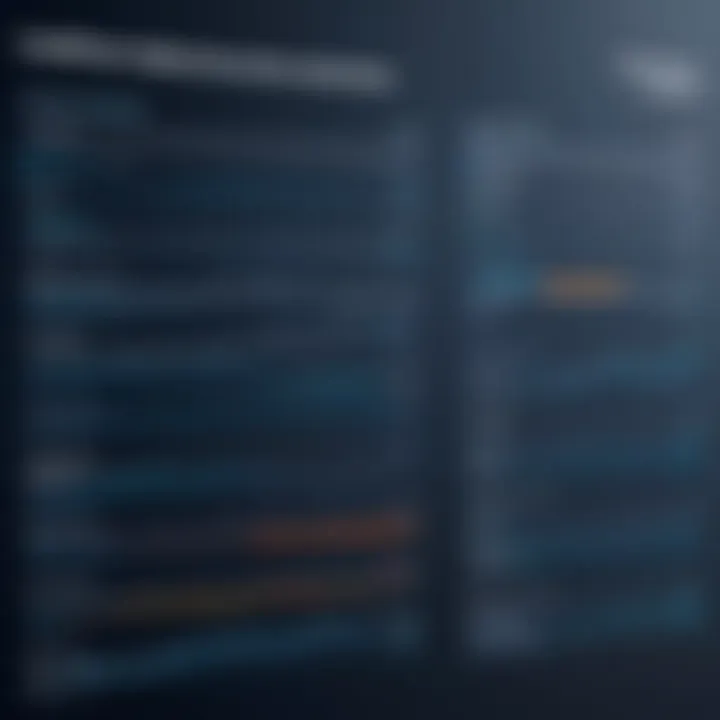
It is important to collaborate with IT teams and data engineers to ensure the selected analytics tools can communicate effectively with legacy systems. This can reduce the likelihood of future integration issues and promote better data flow.
User Adoption Concerns
Even with the right tools in place, user adoption remains a significant hurdle for many organizations. Employee reluctance to embrace new technology can stem from fear of the unknown or lack of training. If users do not fully understand how to utilize the embedded analytics features, they may revert to familiar methods, thus missing out on insights and efficiencies.
Key considerations for fostering user adoption include:
- Providing comprehensive training programs
- Ensuring clear communication of the benefits of using embedded analytics
- Encouraging feedback and making adjustments based on user experiences
Promoting a culture of data-driven decision making is vital. Organizations should promote success stories and demonstrate the practical benefits of adopting these analytical tools. By emphasizing how these tools can improve workflows and outcomes, organizations can alleviate fear and encourage greater user engagement.
Successful implementation of embedded analytics often hinges on addressing technical barriers and fostering user engagement.
Best Practices for Maximizing Embedded Analytics Effectiveness
In the realm of embedded analytics, maximizing effectiveness requires a strategic approach. Implementing best practices can significantly enhance data utilization, decision-making, and overall user experience. The focus here is on how to tailor analytics solutions to meet specific business needs and the necessity of fostering continuous learning and adaptation. These two core areas are pivotal in ensuring that embedded analytics fully serves its purpose within any organization.
Tailoring Solutions to Business Needs
Every organization is unique, with distinct challenges and objectives. Therefore, it is crucial to tailor embedded analytics solutions to meet these specific needs. Here are key considerations to ensure effective tailoring:
- Understand the Stakeholders: Identify who will use the analytics and their requirements. Sales teams may need different insights compared to marketing or operational teams.
- Define Clear Objectives: Outline the precise goals of embedding analytics. Are you looking to improve customer insights, enhance operational efficiency, or drive sales growth?
- Select the Right Tools: Not all tools fit every use case. Evaluate tools based on their ability to deliver relevant data visualizations and insights suited to your business context.
- User Customization Options: Providing users with options to customize dashboards or reports increases engagement and makes the analytics more relevant to their daily tasks.
Focusing on these aspects makes it easier to adapt the embedded analytics effectively, leading to a more impactful integration into existing workflows. The better the analytics align with actual business processes, the higher the likelihood of user adoption and satisfaction.
Continuous Learning and Adaptation
The world of data analysis is constantly evolving. Therefore, it is essential for organizations to embrace a culture of continuous learning and adaptation regarding embedded analytics. Some useful practices include:
- Regular Training Sessions: Conduct scheduled training for users to learn about new features or updates in the embedded analytics tools. This keeps the staff competent and confident in using the tools.
- Feedback Loops: Implement channels for users to provide feedback on their experiences. Gathering insights on what works and what doesn’t helps inform future improvement initiatives.
- Monitor Usage and Outcomes: Utilize data to understand how analytics tools are being used and the outcomes they produce. Adjust analytics strategies based on this data-driven insight.
- Stay Updated with Technology Trends: Keeping abreast of emerging technologies and trends in analytics will equip organizations with innovative ideas to improve their embedded analytics.
"Adapting your analytics strategy is not just about tools; it's about aligning with the dynamic nature of data."
Future Trends in Embedded Analytics
The landscape of embedded analytics is rapidly transforming. As businesses strive for more data-driven decision-making, understanding the future trends in this domain becomes crucial. These trends not only influence how organizations use analytics but also shape the tools that power these insights.
Artificial Intelligence and Machine Learning Integration
Artificial intelligence (AI) and machine learning (ML) are set to be game-changers in the realm of embedded analytics. The integration of AI and ML facilitates advanced data analytics capabilities that were not possible before. With these technologies, tools can analyze complex datasets and uncover patterns that might go unnoticed by human analysts.
Businesses can benefit from automated data preparation, anomaly detection, and predictive analysis, which enhance decision-making processes. For instance, organizations can leverage predictive analytics to forecast trends and behaviors, allowing them to adjust strategies proactively.
Moreover, AI can improve user experience through personalized analytics. By learning from user interactions, AI algorithms can tailor dashboard displays, recommend specific analyses, and simplify data navigation. This added layer of intelligence means that users—regardless of their technical expertise—can derive insights tailored to their needs.
The Role of Cloud Technologies
Cloud technologies have a significant impact on how embedded analytics tools evolve. The shift to cloud-based solutions enables organizations to access and analyze data from virtually anywhere. This flexibility is crucial in today’s remote work environment.
Cloud solutions often come with scalability benefits. Organizations can scale their analytics resources up or down based on demand, optimizing costs. Additionally, the cloud can facilitate real-time data processing and collaboration across teams, enhancing project outcomes.
Furthermore, cloud technologies support the integration of various data sources. Embedded analytics tools can pull information from CRM systems, marketing platforms, and other applications, providing a more holistic view of organizational performance.
End
The conclusion of this article is a pivotal element that encapsulates the key points discussed throughout. It emphasizes the relevance of embedded analytics tools in today’s data-driven landscape. Recognizing their importance aids businesses and professionals in making informed decisions, ultimately enhancing operational efficiency and strategic planning.
Summarizing Key Insights
In summary, embedded analytics tools have proven vital for organizations aiming to leverage data effectively. They bring together various functionalities, including real-time data processing, user-friendly interfaces, and excellent data visualization capabilities. Let’s highlight a few key insights:
- Integration: Most modern tools seamlessly mesh with existing systems, reducing the friction of adoption.
- User Experience: A good tool prioritizes an intuitive design, promoting user engagement and satisfaction.
- Cost vs. Value: It’s critical to assess pricing structures against the benefits offered, ensuring it aligns with business objectives.
Moreover, businesses benefit from tailored solutions that meet their unique requirements, providing a competitive edge in their respective markets.
Final Thoughts on Choosing Tools
When selecting embedded analytics tools, one must consider several factors. Clearly define your organizational needs before diving into available options. The choice should not only focus on features but also on ease of integration and support services.
- Assess the scalability of the tool. Will it grow with your needs?
- Evaluate user feedback and case studies related to the tool’s performance.
- Consider long-term vendor partnerships for ongoing support and updates.