Mastering A/B Testing Statistics for Better Insights
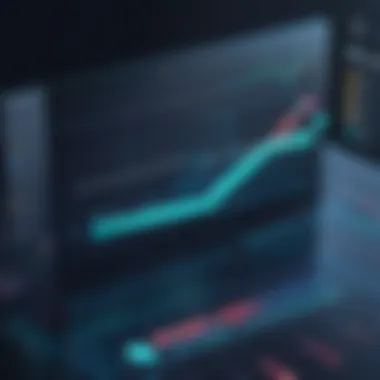
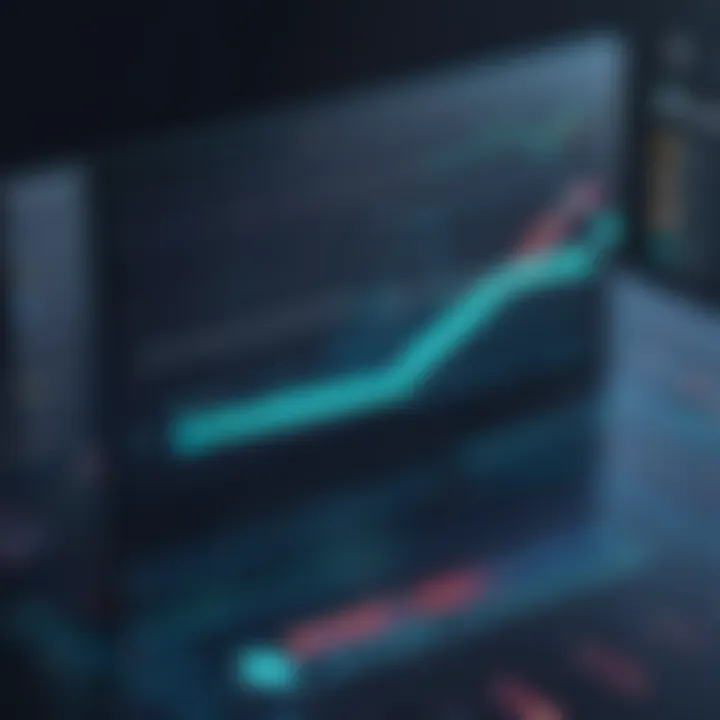
Intro
A/B testing is a powerful methodology used in various fields, especially marketing and product development. It allows professionals to compare two versions of a single variable to determine which one performs better. By examining user responses to different scenarios, businesses can make informed decisions that ultimately enhance user experiences and improve overall results. Understanding the statistics behind A/B testing is crucial for accurate interpretation and implementation.
In the realm of data-driven decision making, A/B testing stands out as a fundamental tool. Through robust statistical analysis, one can extract meaningful insights from controlled experiments. This article aims to dissect the intricate layers of A/B testing statistics. The focus will be on methodologies, statistical principles, interpretations, and common pitfalls to enhance the effectiveness of A/B testing.
The relevance of A/B testing is not just confined to academic theories; it has real-world applications that can lead to increased engagement and revenue. As we delve deeper, we will unravel how these statistics drive successful strategies in various industries. Let's investigate this essential aspect of modern analytics.
Preface to A/B Testing
A/B testing plays a crucial role in various fields, especially in marketing and product development. The essence of A/B testing is to compare two versions of a certain variable to determine which performs better. This can range from different webpage designs to variations in marketing emails. Understanding A/B testing allows professionals to make informed decisions backed by data, thus improving user engagement and conversion rates.
The benefits of A/B testing are numerous. It enables businesses to optimize their strategies by providing concrete evidence of what works and what does not. Moreover, it fosters a culture of continuous improvement. In an age where user experience is paramount, A/B testing serves as a valuable tool for data-driven decision making. However, it also requires careful consideration. Factors such as sample size, test duration, and the choice of metrics can significantly influence outcomes.
In this segment, we explore what A/B testing encompasses and why its historical development is essential to understanding its current applications.
Definition and Purpose
A/B testing, often referred to as split testing, is a method used to compare two versions of a webpage, app feature, or other elements. By having users interact with both variants—A and B—businesses can gather data on user preferences and behaviors. Its primary purpose is to identify which version yields better results based on predetermined metrics such as click-through rates, conversion rates, and engagement levels.
The straightforward nature of A/B testing makes it accessible and practical. Different hypotheses can be tested without requiring extensive changes to existing systems, allowing companies to incrementally improve their offerings. Furthermore, A/B testing can inform a variety of business decisions, from marketing strategies to product features, proving its versatility in application.
Historical Context
The concept of A/B testing dates back several decades. It saw early adoption in fields like clinical trials and experimental psychology, where it was essential to ascertain effective treatments or behavioral strategies. The rise of the internet and digital marketing in the late 1990s gave A/B testing a new dimension, particularly in web design and advertising.
Notably, companies like Google and Facebook popularized A/B testing in the tech industry, demonstrating its value in making real-time improvements to user experiences. They provided case studies that showcased how small changes could significantly affect user behavior and, consequently, revenue growth. Today, A/B testing is an indispensable aspect of digital strategy across various sectors, as organizations seek to leverage data to drive success.
Understanding the roots and evolution of A/B testing equips professionals with insights not only into its methods but also its transformative power in decision-making processes.
Mathematics Behind A/B Testing
The mathematics behind A/B testing forms a cornerstone for drawing significant conclusions from tests. Understanding the underlying statistical principles enables better design, execution, and interpretation of the results. A proper grasp of these concepts ensures that business stakeholders can make informed decisions that lead to data-driven improvements.
Basic Statistical Concepts
Population vs. Sample
In A/B testing, differentiating between population and sample is critical. The population represents the entire group from which data might be collected. This could be all users visiting a website. In contrast, the sample is a smaller, manageable subset of the population chosen to participate in the test. The ideal sample should reflect the characteristics of the larger population. A primary aspect of using a sample is that it allows for efficiency in analysis while maintaining enough accuracy to generalize findings. A sample is often the chosen way due to practical limitations, such as time and resources. However, a unique feature to consider is that if the sample is biased, it can skew results. Hence, ensuring that the sample selection process is representative is a crucial point of concern.
Random Sampling
Random sampling is a method that helps mitigate the risks of bias when selecting samples. Its main characteristic lies in the fact that each individual in the population has an equal chance of being chosen for the test. By employing random sampling techniques, researchers can enhance the reliability of their findings. A unique advantage of random sampling is the reduction of variability within the sample. It often results in more accurate estimates of the population parameters. However, the challenge arises when random samples are hard to achieve – particularly in situations where data is limited or hard to access. Only gathering a non-representative sample can impede the quality of results and lead to improper conclusions.
Hypothesis Testing
Null Hypothesis
The null hypothesis is a fundamental concept in hypothesis testing, which serves as a starting point for statistical analysis. It typically states there is no effect or difference between the groups being tested. This hypothesis helps researchers to establish a baseline to compare against assuming conditions remain unchanged. Its importance lies in providing a prevalent framework from which to assess evidence against it. While it is easy to formulate, rejecting the null hypothesis requires careful calculation of statistical tests. A potential downside is that an improper formulation of the null hypothesis can lead to misleading outcomes.
Alternative Hypothesis
In contrast, the alternative hypothesis proposes that there is a measurable effect or difference. It represents what the researcher aims to prove. Understanding the alternative hypothesis is crucial as it guides the direction of the testing. It is beneficial in that it allows exploration beyond mere confirmation of the null. The distinctive feature of this hypothesis is that it often drives experiments based on desired outcomes. However, formulating an alternative hypothesis can sometimes lead to over-specification, making it complex to validate without sufficient evidence.
"Statistical principles guide the correct design and interpretation of A/B tests. Knowing what to measure is as critical as knowing how to measure it."
In summary, the mathematics behind A/B testing provides a structured approach to making comparisons. Understanding population and sample dynamics alongside hypothesis testing is vital for valid results. Consequently, solid mathematical grounding offers a pathway to more effective A/B testing methodologies.
Designing an A/B Test
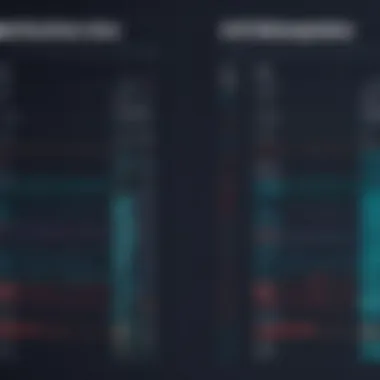
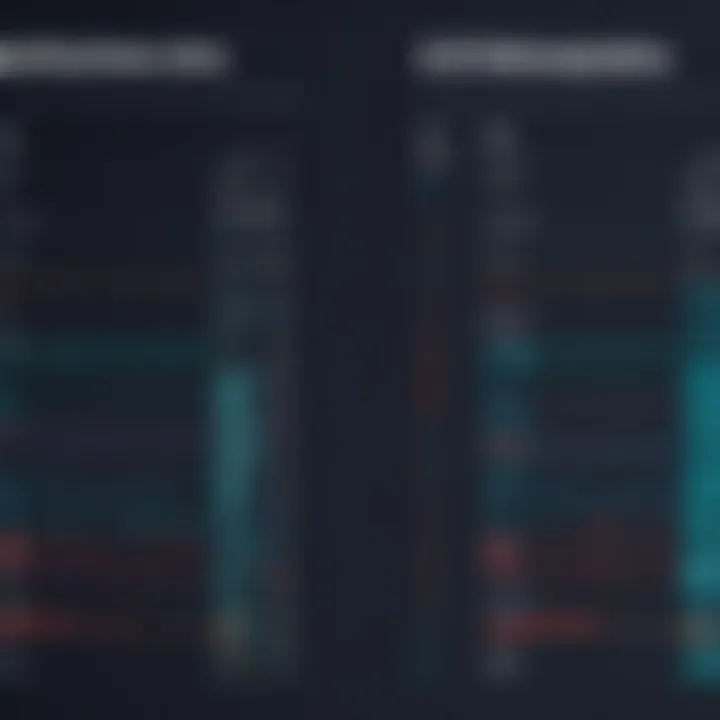
Designing an A/B test is a critical step in the A/B testing process. It involves thoughtful planning and a clear understanding of why the test is being conducted. This design is where the foundation for reliable results is laid. A well-designed test can lead to actionable insights, while a poorly designed one may yield misleading findings and wasted resources.
Identifying Objectives
The first task in designing an effective A/B test is to identify the objectives. These objectives specify what the test seeks to achieve. It can be improving website conversion rates, enhancing user engagement, or increasing sales. Each objective should be specific, measurable, and relevant to the business goals. Here are important points to consider:
- Specificity: Avoid generic goals. Instead of aiming to "increase traffic", focus on "raising monthly sign-ups by 20%".
- Measurement: Decide how success will be measured. Use metrics like conversion rate, click-through rate, or customer satisfaction scores.
- Alignment: Ensure that the objectives align with broader business strategies. This ensures that the findings from the test can be appropriately integrated into future campaigns.
"Defining clear objectives is the most important step in A/B testing. Without them, any insights gained may not support strategic decisions effectively."
Choosing Variables to Test
After identifying objectives, the next step is to choose the variables to test. Variables should be relevant to the objectives set earlier. Testing multiple variables at once can lead to confusion, making it hard to discern what influenced the results. Here are some factors to remember when selecting variables:
- Limit to One Variable: Each test should focus on a single variable to yield clear results. Testing a new design alongside a pricing change can muddle the outcomes.
- Prioritize High-Impact Areas: Choose aspects that have significant potential to affect user behavior. This might include headlines, call-to-action buttons, or even the layout of the product page.
- Operational Feasibility: Ensure that the changes can be implemented without significant disruption. If a change requires excessive resources or time, it may not be worth testing.
By approaching the design phase attentively, you can set the stage for generating meaningful data from A/B tests. This approach fosters an environment where informed decisions can be made based on solid evidence.
Statistical Significance in A/B Testing
Statistical significance is a central concept in A/B testing. It helps to determine whether the result of an A/B test is likely due to chance or represents a true effect. Understanding the significance helps businesses make informed decisions based on the data. When a result is statistically significant, it indicates confidence that the observed differences between variations are not simply random fluctuations. Therefore, grasping statistical significance is not just a theoretical exercise; it has practical implications for risk management and resource allocation.
Key elements to consider include the choice of significance level, typically set at 0.05. This level implies a 5% risk of concluding that a difference exists when there is none. Thus, a low p-value indicates strong evidence against the null hypothesis, which generally posits that there is no difference between the test groups. Companies need to balance the risks of Type I and Type II errors, leading to improved design for better decision-making.
Benefits of Statistical Significance
- It allows for reliable conclusions about user behavior.
- It minimizes the chances of flawed decision-making based on biased sample variance.
- It facilitates communication of results among stakeholders who may not be statistically inclined.
While determining statistical significance is vital, nuances surround it. Factors like sample size, variability in data, and duration of the test also significantly impact results. Running tests long enough for accurate data can prevent rushed conclusions. If done thoughtfully, it enhances overall business performance by fostering data-driven outcomes.
Analyzing A/B Test Results
A/B test results analysis is a crucial stage in the testing process. It allows decision-makers to gauge the effectiveness of various variables tested during an experiment. The outcome influences selections ranging from design choices to content variations in marketing campaigns. Analyzing these results directly impacts business strategies, making it essential for organizations looking to enhance their user experience and optimize conversion rates. With precise analysis, companies can identify trends and make data-driven decisions that lead to growth and success.
Interpreting Data Outputs
Interpreting data outputs involves translating raw data from A/B tests into actionable insights. It's important to understand what the metrics signify. Often, A/B tests will generate metrics such as conversions, engagement rates, or clicks. These numbers alone do not tell the full story. One must look beyond the basic statistics to understand their implications.
A key aspect to consider is statistical significance. When evaluating results, a p-value helps determine if the observed differences between groups are genuinely meaningful or if they could occur by chance. Generally, a p-value below 0.05 indicates that the results are statistically significant.
Another important measure is the confidence interval. This statistic provides a range within which the true effect of a variable might lie. A narrower interval suggests a more precise estimate. When analyzing data outputs, professionals must take both significance and confidence into account to make informed conclusions.
Outcomes should also be segmented for deeper insight. By breaking down results by demographics or behaviors, businesses can identify patterns that are not immediately obvious.
"Data analysis is not just about numbers; it’s about understanding what those numbers mean for the business."
Visualization Techniques
Visualization techniques play a significant role in interpreting A/B test results. They transform raw data into visual formats that facilitate easier comprehension. Visuals such as graphs, charts, and histograms can reveal trends and inform stakeholders quickly.
Some common visualization methods include:
- Bar Charts: Useful for comparing conversion rates between different variations.
- Line Graphs: Effective for showing changes over time, like daily conversion rates during the test period.
- Heatmaps: Help to identify user interaction flows and where users click most frequently.
Using software like Google Analytics or Tableau can simplify this process, as they provide tools to create dynamic visualizations from your A/B test data.
Crafting clear visualizations allows teams to communicate findings more effectively to non-technical stakeholders. Thus, conveying what the metrics mean in an accessible way becomes easier. Proper visualization of results strengthens the foundation for informed decision-making and strategic planning.
Common Pitfalls in A/B Testing
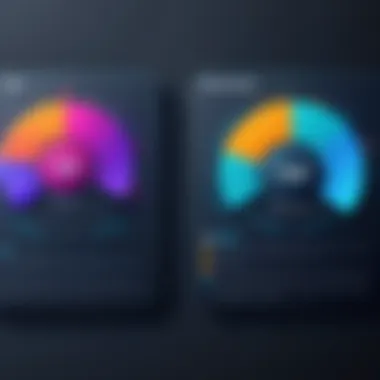
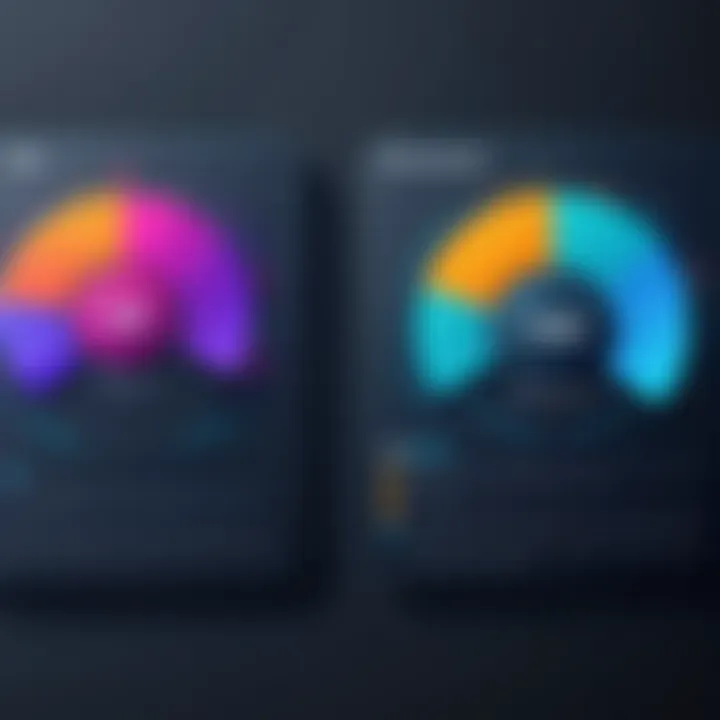
Understanding the common pitfalls in A/B testing is crucial for any professional engaged in data-driven decision-making. A/B testing, while a powerful tool, can lead to erroneous conclusions if not executed correctly. By being aware of potential missteps, individuals can enhance their testing outcomes and derive meaningful insights.
Sampling Bias
Sampling bias occurs when the sample selected for the A/B test does not accurately represent the entire population. This bias can significantly skew results, leading to false interpretations. For instance, if a website tests a new design only on frequent visitors, the feedback might not reflect the views of new visitors who may interact differently with the site.
To mitigate sampling bias, it's essential to employ random sampling techniques. Each user should have an equal chance of being placed in either the control or experimental group. This ensures that the findings can be generalized across the intended population. Here are key points to consider:
- Diverse User Base: Ensure the sample accurately reflects various user segments.
- Date Selection: Be cautious about specific dates; sudden spikes in traffic may misrepresent user behavior.
- User Interaction: A comprehensive tracking system can help understand interactions from different demographics.
"Sampling bias can tarnish the integrity of your test results, proving the importance of representation in your data collection methods."
Multiple Testing Issues
Conducting multiple A/B tests simultaneously can lead to increased chances of false positives, also known as Type I errors. When performing numerous tests on the same dataset, the likelihood that at least one of the tests will indicate a statistically significant result simply due to random chance also increases.
To tackle this issue, implementing correction methods, like the Bonferroni correction, is advised. This method adjusts the significance level based on the number of comparisons made. Below are considerations to keep in mind:
- Predefine Tests: Clearly define which tests to run before analyzing the data to avoid unnecessary comparisons.
- Use Correction Techniques: Apply statistical techniques to adjust for multiple tests.
- Analyze in Phases: Instead of simultaneous tests, consider running tests sequentially to better manage data interpretation.
By understanding these common pitfalls, professionals can refine their A/B testing strategies, leading to more reliable results and actionable insights.
Best Practices for A/B Testing
Implementing A/B testing presents a unique opportunity for professionals to derive insights and optimize performance across various digital interfaces. Understanding best practices is crucial because these guidelines not only enhance the validity of results, but they also ensure that conclusions drawn from the testing are sound and actionable. Adopting best practices in A/B testing can drastically elevate both user experience and business efficiency.
Establishing Clear Metrics
Clear metrics are the foundation upon which A/B tests are built. Having well-defined metrics allows you to measure success accurately. Without proper metrics, it becomes challenging to determine if changes yield positive results.
Metrics should align directly with your objectives. Here are some key metrics to consider:
- Conversion Rate: Measures the percentage of users who complete the desired action, such as making a purchase or signing up for a newsletter.
- Click-Through Rate (CTR): Indicates how many users click on a specific link or call-to-action compared to how many viewed it, reflecting engagement level.
- Bounce Rate: Measures the proportion of visitors who leave after viewing only one page. A high bounce rate may indicate issues with the content or layout.
When you clearly define which metrics to track, you minimize ambiguity. Clear metrics will guide the test design and strategy effectively, making it easier to evaluate results. Additionally, clearly stating your success criteria beforehand ensures that you maintain focus throughout the testing period.
Duration and Sample Size Considerations
Deciding on the duration and sample size of your A/B tests is critical for obtaining reliable results. Testing for too short a period or with too small a sample can lead to misleading conclusions. Conversely, unnecessarily extending a test can waste resources and cause decision-making delays.
- Sample Size: A larger sample size typically provides more reliable data. Tools such as calculators can help determine the appropriate sample size based on expected conversion rates and desired significance levels.
- Duration: The test duration should avoid any skewed results due to external factors. For instance, testing over a weekend might not be representative of a weekday’s performance. It's best to run tests across multiple cycles, including weekdays and weekends, to capture a more holistic view of user behavior.
- Utilize tools that can automate and integrate with your analytics platforms. Examples like Google Optimize or Optimizely provide insights that recommend sample sizes and testing durations based on your data.
Ultimately, establishing a suitable duration and sample size for A/B testing allows for a more effective analysis, leading to actionable insights that can significantly improve performance.
Real-World Applications of A/B Testing
The significance of A/B testing extends far beyond theoretical frameworks. It has been effectively integrated into various industries, demonstrating its power in making informed decisions based on actual data. Organizations leverage A/B testing to optimize platforms, user interactions, and marketing strategies. This methodology offers specific benefits and considerations that are crucial for professionals in fields like software development, marketing, and user experience design.
A/B testing allows for real-time experimentation, making it possible to validate assumptions and evaluate different approaches before wide-scale implementation. It is a method that supports evidence-based decision-making. This is particularly valuable in a landscape saturated with competing ideas and techniques. By applying A/B testing, organizations can significantly reduce the risk that comes with changes, ensuring they invest resources into the most effective strategies.
E-Commerce Optimization
The e-commerce landscape thrives on constant innovation and improvement. In this context, A/B testing plays a pivotal role in enhancing user experience. Online retailers often face the challenge of balancing aesthetics and functionality. Testing can guide these decisions.
For instance, changes to the layout of a product page can dramatically affect conversion rates. An updated design might look better but may also deter potential buyers if they find it less intuitive. Conversely, a simpler design might enhance navigation, leading to more sales.
Using A/B testing, e-commerce platforms can experiment with:
- Product Page Layout: Adjusting images, descriptions, or buttons to see what engages users more effectively.
- Checkout Process: Testing multiple steps within the process to reduce cart abandonment rates.
- Pricing Strategies: Experimenting with various price points to determine what maximizes revenue.
Through these methods, e-commerce companies can create a tailored experience that resonates with their customers, ultimately leading to better retention rates and increased sales.
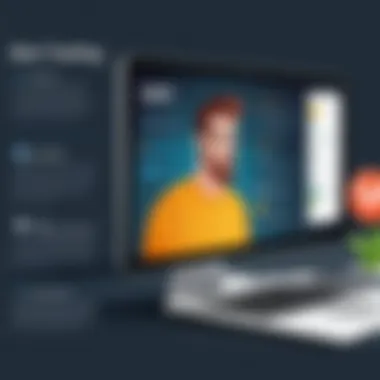
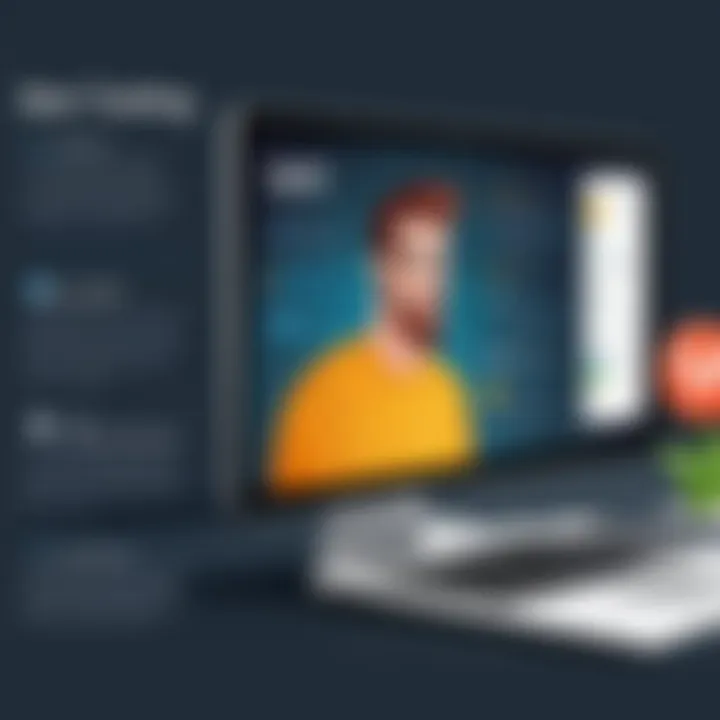
Marketing Campaign Enhancements
In marketing, the effectiveness of campaigns can be difficult to quantify. A/B testing offers marketers the ability to analyze different messaging strategies. This assists in optimizing engagement rates.
Emails, advertisements, and landing pages are common areas where A/B testing is utilized. Marketers might test:
- Subject Lines in Emails: Crafting different subject lines to see which generates higher open rates.
- Ad Copy Variations: Changing headlines or calls to action to improve click-through rates.
- Landing Page Designs: Modifying elements to determine which version encourages more conversions.
By effectively implementing A/B testing, marketers can identify the most compelling elements that drive user actions. This results in campaigns that not only reach a wider audience but also prompt more interactions and conversions.
"A/B testing is not just about the test; it's about understanding what resonates with your audience and why."
Tools for A/B Testing Analysis
A/B testing analysis relies heavily on the right tools. These tools equip professionals with the necessary capabilities to conduct tests effectively. Utilizing proper tools can provide precision, save time, and yield better insights. As the field of A/B testing evolves, integrated solutions that combine various functionalities have emerged, offering marked advantages.
The importance of tools in A/B testing analysis includes:
- Efficiency: Good software can automate various aspects of testing, from segmentation to reporting. This allows teams to focus on analyzing results rather than processing data.
- Ease of Use: User-friendly interfaces ensure that even those with minimal statistical knowledge can conduct proper experiments.
- Collaboration Features: Many modern tools enable multiple stakeholders to collaborate efficiently in real-time, fostering an environment of shared knowledge and collective decision-making.
When weighing options, it's essential to consider sample size, statistical tests available, and the integration capabilities with other analytics platforms.
Software Solutions Overview
Several software solutions can facilitate A/B testing. These tools provide diverse features that address different aspects of A/B testing.
Popular options include:
- Optimizely: This platform offers robust A/B testing capabilities. It allows users to run targeted tests based on user demographics and behaviors.
- VWO (Visual Website Optimizer): This tool combines A/B testing with heatmaps and user recordings. This comprehensive approach helps in understanding user interactions.
- Google Optimize: A free tool that's tightly integrated with Google Analytics. It is suitable for teams looking for an effortless entry into A/B testing.
- Adobe Target: With capabilities for personalization, Adobe Target provides a powerful suite for sophisticated experiments involving multiple variables.
Choosing the right tool requires understanding the specific needs of your project. Each software has unique strengths that may suit different testing scenarios.
Integrating Analytics Tools
Integrating analytics tools with A/B testing platforms is vital. This synergy not only enhances the efficiency of data collection but also improves the accuracy of reporting. Good integration allows for a seamless flow of information, providing a clearer picture of user behavior.
Considerations for integration include:
- Data Consistency: Ensure that data collected aligns with other analytics tools. This will help in maintaining accurate insights.
- Tracking Capabilities: Select tools capable of tracking user interactions effectively. This is crucial for understanding test performance accurately.
- Flexible APIs: Using tools with robust APIs enables better customization and allows the integration of different data sources.
- Data Visualization: Integration should also facilitate clearer data presentation, making it easier to understand and act on test results.
Often, organizations will find value in tools that offer pre-built integrations with commonly used analytics platforms, which can save time and reduce errors in data handling.
Ending
A conclusion in any study or examination plays a vital role in synthesizing the findings presented throughout the article. In the context of A/B testing statistics, this section serves several important functions. It allows for a summary of the key takeaways, which helps reinforce the core messages and insights the audience should remember. By distilling complex information into essential points, the conclusion aids in ensuring that the valuable lessons learned are not lost.
Furthermore, the conclusion enables readers to reflect upon the significance of A/B testing in their own practices. It is not merely about presenting results; rather, it highlights how A/B testing can facilitate data-driven decision-making. In today’s competitive environment, understanding how to effectively interpret test results can drive significant business outcomes. A well-crafted conclusion leaves readers with a clear understanding of why they should value A/B testing as a tool for optimization and improvement in their respective fields.
Summarizing Key Takeaways
Key takeaways serve as a foundation for understanding the entire article on A/B testing statistics. Here are some key points to consider:
- A/B Testing Basics: It is essential to grasp both the definition and purpose of A/B testing to recognize its role in user experience enhancement.
- Statistical Principles: Understanding concepts such as p-values and confidence intervals is crucial for analyzing results correctly.
- Common Pitfalls: Awareness of issues like sampling bias and multiple testing is key to ensuring valid results.
- Best Practices: Establishing metrics and determining appropriate duration for tests can optimize outcomes significantly.
"Understanding the nuances of A/B testing is paramount for any professional looking to leverage data effectively."
These points encapsulate the importance of A/B testing while providing foundational knowledge for effective application.
Future Directions in A/B Testing
The field of A/B testing is evolving rapidly, and several trends are shaping its future. Here are some potential developments to anticipate:
- Automated Testing Solutions: With advancements in machine learning, automated A/B testing tools will become more prevalent. These solutions may allow for faster iterations and more sophisticated analysis.
- Integration with AI: The integration of artificial intelligence could enhance the personalization of tests, allowing for more tailored experiences for users based on their behavior.
- Enhanced Data Privacy: As data privacy regulations become stricter, A/B testing methodologies will need to adapt, ensuring compliance while still providing insightful results.
- Cross-Platform Testing: As users engage across multiple devices, the focus on cross-platform testing will likely increase, necessitating more comprehensive test designs.
These forthcoming changes in A/B testing indicate that staying informed and adaptable will be critical for those in the field. As technology continues to evolve, professionals will need to embrace new approaches within A/B testing to ensure their strategies remain effective and relevant.