The Evolution of Open Source Forecasting Software
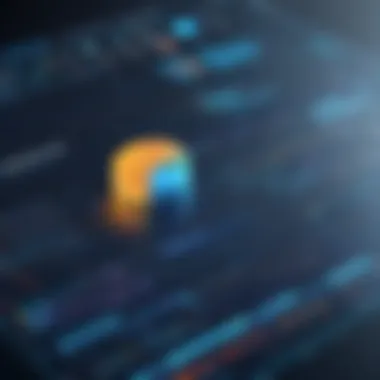
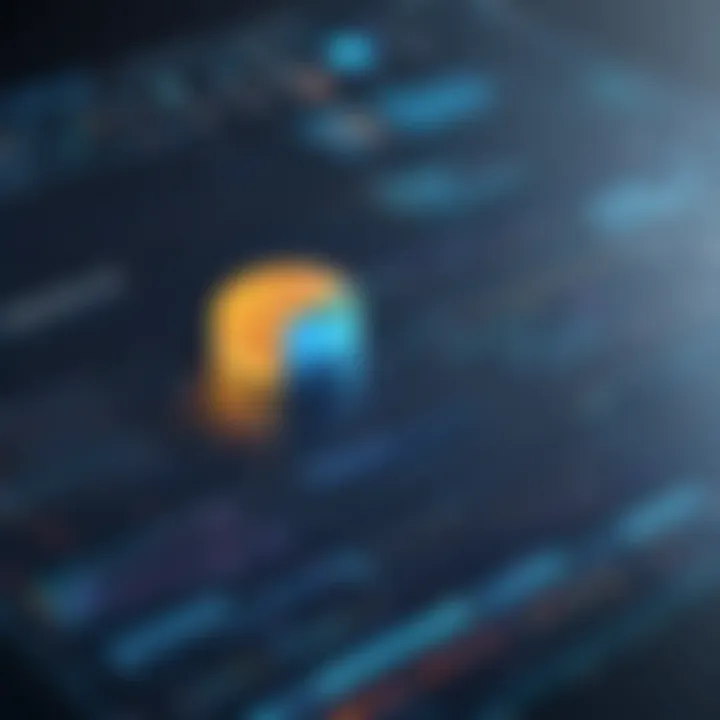
Intro
Open source forecasting software has started to shape the landscape of analytics in profound ways. In a world increasingly reliant on data-driven decisions, the importance of accurate forecasting cannot be overstated. The evolution of these tools has been influenced by various factors, such as technological advances and community engagement. By grasping the journey of open source forecasting software, both developers and professionals are better positioned to tap into its potential benefits.
Software Overview
Key Features
Open source forecasting software typically possesses several key features that set it apart from proprietary alternatives. Some of these features include:
- Flexibility: Users can modify the source code to fit specific needs, allowing for greater customization.
- Community Support: These platforms often benefit from active communities. Gain access to shared knowledge, troubleshooting, and enhancements.
- Cost Efficiency: As the name suggests, open source tools usually come with no licensing fees. This aspect makes them attractive to organizations of all sizes.
- Interoperability: Many open source forecasting solutions are designed to work well with existing systems, which aids in seamless integration.
System Requirements
To effectively use open source forecasting software, understanding system requirements is crucial. While the specifics may vary by platform, general needs often include:
- Operating system: Linux, macOS, and Windows are commonly supported.
- Memory: A minimum of 4 GB of RAM is advisable, although larger datasets may require more capacity.
- Processor: Dual-core or higher recommended for optimal performance.
- Storage: Sufficient disk space is necessary to accommodate datasets and software installations.
In-Depth Analysis
Performance and Usability
When considering open source forecasting tools, performance, and usability cannot be overlooked. While many users prioritize a robust feature set, ease of use is equally significant. Open source platforms often offer clean interfaces, which allow users to navigate and execute tasks without a steep learning curve. Performance benchmarks can vary, but some tools have proven effective for extensive data analyses, making them suitable for diverse industries.
Best Use Cases
Identifying appropriate use cases for open source forecasting software can enhance project outcomes. Some notable applications include:
- Financial Forecasting: Many financial institutions deploy these tools to predict market trends and manage risks effectively.
- Supply Chain Management: Businesses utilize forecasting software to improve inventory management and logistics.
- Demand Planning: Retailers can analyze sales data to better predict consumer demand patterns.
"The true strength of open source forecasting software lies in its adaptability to a variety of sectors and applications."
By understanding the features, performance, and best use cases of open source forecasting software, professionals in IT-related fields will be better equipped to make informed decisions. Evaluating specific tools against distinct requirements is essential to leveraging their full potential.
Prelims to Open Source Forecasting Software
In recent years, open source forecasting software has garnered significant attention in various fields, from finance to healthcare. The importance of this topic lies in its ability to provide accessible tools that can adapt to different environments and needs. Utilizing open source solutions enhances transparency and fosters innovation through community collaboration.
Definition and Overview
Open source forecasting software refers to tools available to the public, allowing users to view, modify, and distribute the code. This model promotes a collaborative environment where developers and analysts can contribute to the tool's evolution. Such software encompasses a range of functionalities, including data analysis, predictive modeling, and visualization. By providing cost-effective solutions, open source software democratizes access to sophisticated forecasting models that were once only available through proprietary products. Users can benefit from collective insights and continuously improving algorithms, catering to various industries and applications.
Historical Context
The journey of open source forecasting software can be traced back several decades. Initially, most forecasting tools were closed-source, requiring substantial investments. However, the rise of the internet facilitated collaboration among developers and analysts, leading to the emergence of several open source projects. Influential programming languages like R and Python have played a pivotal role in this evolution, as they offer libraries and frameworks specifically designed for statistical analysis and machine learning. Today, open source project repositories, such as those on GitHub, provide ample resources for developers, enabling a culture of sharing and learning that continues to grow.
"Open source is a development methodology, not a business model." - Karl Fogel
This quote underscores the essence of open source forecasting tools; they prioritize collective advancement over profit. Overall, the evolution of these tools showcases an ongoing shift toward community-driven development and the increasing recognition of the vital role forecasts play in decision-making processes.
The Importance of Forecasting
Forecasting is crucial in today's data-driven world. It provides organizations with the ability to predict future trends based on historical data. This practice allows for informed decision-making, helping companies to mitigate risks, allocate resources effectively, and identify new opportunities. In the context of open source forecasting software, understanding the importance of forecasting is not only beneficial but essential.
Applications Across Industries
Forecasting spans a range of industries, from finance to retail. In finance, firms use forecasting to enhance investment strategies. They analyze trends to anticipate market movements. Retailers apply forecasting to manage inventory. Accurate forecasts help avoid overstock and stockouts, optimizing sales and serving customers better.
In healthcare, forecasting facilitates resource allocation. Hospitals estimate patient admissions, ensuring adequate staff and equipment. Similarly, in manufacturing, forecasting predicts demand, enabling efficient production schedules and reducing waste. With the flexibility of open source solutions, industries can tailor forecasting models to meet specific needs, enhancing their effectiveness.
Relevant industries utilizing forecasting include:
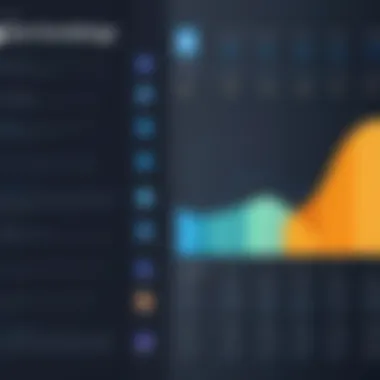
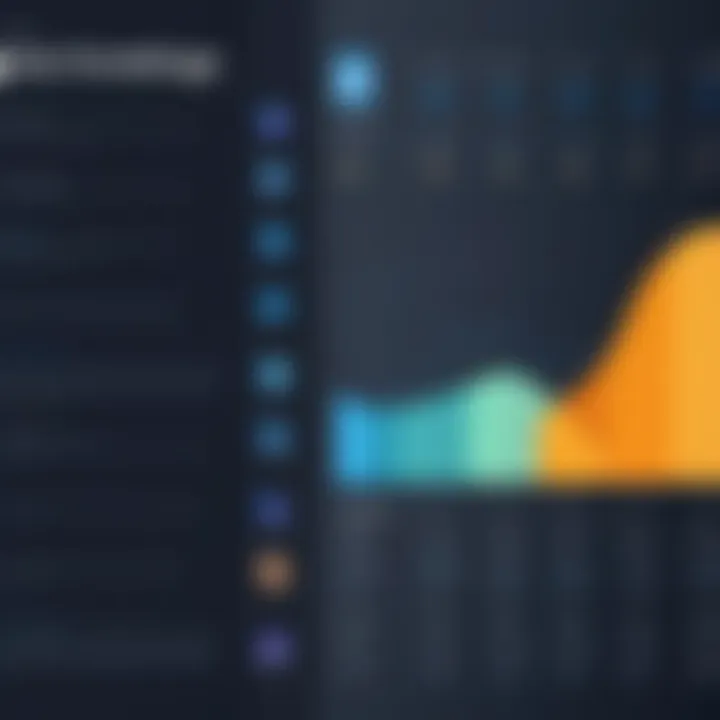
- Finance
- Retail
- Healthcare
- Manufacturing
- Transportation
Enhancing Decision-Making Processes
Effective decision-making relies heavily on reliable data and accurate predictions. Forecasting provides the insights necessary for sound business strategies. Organizations equipped with forecasting tools can assess multiple scenarios. By understanding potential outcomes, they can choose paths aligned with their goals. Open source software amplifies this capability through customization and community support.
Incorporating forecasting in decision-making processes allows organizations to:
- Identify trends and patterns in data
- Analyze the impact of different variables
- Simulate potential future events
This analytical approach not only improves immediate decisions but also builds a foundation for long-term planning. Consequently, businesses can adapt to changes in the market more readily, maintaining a competitive edge.
"Forecasting is not about predicting the future; it is about making informed choices based on careful analysis of data."
The implications of forecasting extend into all corners of an organization. As such, the emphasis on utilizing open source forecasting software cannot be overstated. Its role in enhancing decision-making processes is vital, facilitating responses to challenges and opportunities alike.
Key Features of Open Source Forecasting Software
Open source forecasting software brings unique characteristics that enhance its utility and appeal. Understanding these key features is crucial for users, as they determine how well the software can meet specific demands. In this section, we delve into the essential attributes, which include customizability and flexibility, community support and collaboration, and cost-effectiveness. Each element plays an influential role in making open source tools viable choices in various industries.
Customizability and Flexibility
The ability to customize and adapt software to meet specific needs is one of the standout features of open source forecasting tools. Users can modify the source code, creating tailored solutions that reflect particular analytical requirements.
- Adaptation to Specific Models: In many cases, businesses have unique forecasting models that do not fit neatly into standard solutions. Open source software allows developers to tweak algorithms or incorporate additional variables.
- Integration Capabilities: These tools often integrate well with existing systems and databases, ensuring a seamless data flow. This capability is critical for organizations that rely on diverse data sources.
Through the lens of flexibility, organizations gain the ability to adjust and scale their tools without being locked into rigid frameworks. This adaptability can be pivotal in fast-evolving markets where rapid response is necessary.
Community Support and Collaboration
Another significant advantage of open source forecasting software is the robust community that supports it. Users can tap into a wealth of collective knowledge and expertise.
- Access to Resources: Many open source projects have documentation, forums, and collaboration spaces where users can ask questions and share solutions. This sense of community is invaluable, especially for less experienced users.
- Continuous Development: The collaborative nature of open source projects means that software is often under continuous improvement. Contributions come from users and developers who identify, report, and fix bugs, or introduce new features.
This community-driven support structure fosters innovation and allows users to benefit from shared advancements in technology.
Cost-Effectiveness
Cost is a pivotal consideration for any software solution. Open source forecasting software is generally more cost-effective than proprietary alternatives, making it attractive for various organizations.
- No Licensing Fees: Users do not have to pay hefty licensing fees for access. This aspect allows organizations to allocate resources to other critical areas, such as staff training or additional computing resources.
- Reduction in Vendor Lock-In: Organizations using open source tools have more freedom. They can shift development efforts in-house or choose from various service providers without being chained to a single vendor’s ecosystem.
Popular Open Source Forecasting Software
Open source forecasting software plays a significant role in today's analytics ecosystem. It allows users to access powerful tools for predictive analytics without incurring high licensing fees. This section highlights some of the most widely used open source forecasting software, underscoring their functionalities, advantages, and the contexts in which they excel. Each software serves different needs, making it essential to understand their respective capabilities as they can cater to various user requirements.
R
R is a widely recognized programming language that is particularly well-suited for statistical analysis and forecasting. With its comprehensive ecosystem that includes packages like and , R allows users to implement both basic and sophisticated forecasting techniques.
Users appreciate the flexibility of R for various data types, whether time series or multivariate data. Its strong visualization capabilities enable users to generate meaningful plots to interpret data outcomes better. For many data scientists, R has become the go-to choice due to the extensive community support and resources available online.
Python Libraries
Python, as a programming language, provides several libraries specifically tailored for forecasting tasks. Libraries such as , , and excel in statistical modeling and machine learning. These tools allow users to easily implement predictive models with minimal setup time.
The integration capabilities of Python with other data processing platforms enhance its appeal. For example, combining Python with Pandas for data manipulation and Matplotlib for visualization forms a robust ecosystem. The synergy between these libraries makes Python a strong candidate for developers seeking a comprehensive forecasting solution.
Apache PredictionIO
Apache PredictionIO is an open-source machine learning server that offers a scalable solution for building predictive engines. It allows developers to create customized forecasting applications using a variety of machine learning algorithms. Its architecture supports both batch and real-time data processing, making it suitable for dynamic environments.
PredictionIO stands out due to its integration capabilities with various data sources and deployment environments. Users can leverage the extensive documentation and user community to fine-tune their applications for optimal results.
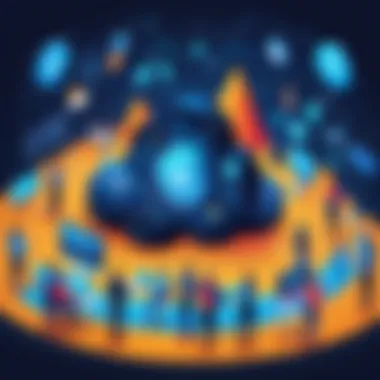
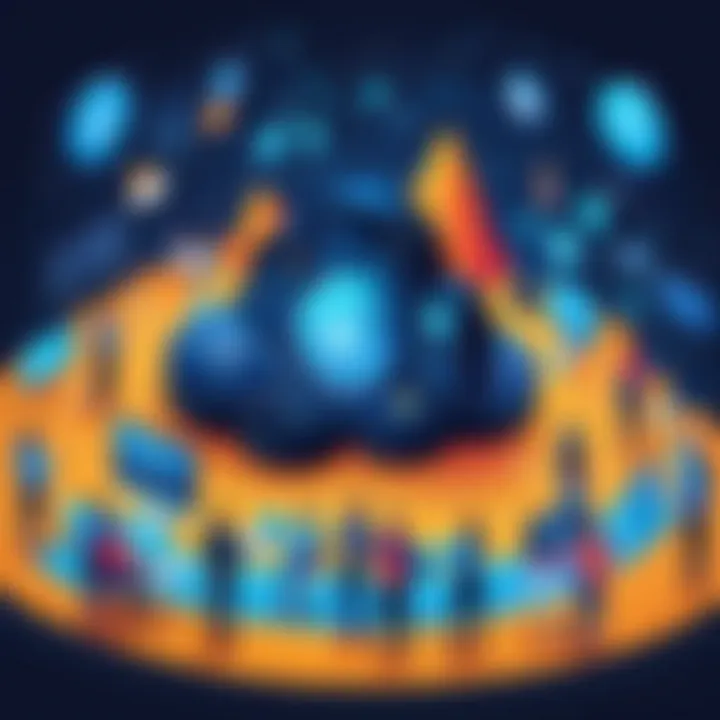
TensorFlow Forecasting
TensorFlow, primarily known for deep learning, also offers robust functionality for time series forecasting. Its framework allows users to build complex models that can capture patterns in large datasets. TensorFlow Forecasting provides user-friendly APIs that facilitate modeling and forecasting tasks.
The advantage of TensorFlow lies in its ability to handle vast amounts of data efficiently, making it ideal for applications in sectors like finance and retail. Users can implement state-of-the-art models, such as recurrent neural networks, to analyze time series data with precision. Furthermore, the strong developer community around TensorFlow ensures continual advancements and offers support for new users.
The choice of open source forecasting software significantly affects the quality of analysis and outcomes in various sectors. Understanding each software’s strengths can lead to more informed decisions in adopting the right tools for specific needs.
In summary, selecting the proper open source forecasting software depends on the user’s specific requirements. R, Python libraries, Apache PredictionIO, and TensorFlow provide diverse functionalities suited to different analytical tasks. Understanding these tools helps in optimizing forecast accuracy and overall business strategy.
Evaluating Open Source Options
Evaluating open source forecasting software is a critical step for organizations aiming to leverage these tools effectively. This evaluation ensures that the chosen software aligns with specific project requirements and integrates seamlessly into existing workflows. Understanding the importance of careful consideration can lead to more accurate forecasts and better overall results.
Criteria for Selection
When selecting open source forecasting software, several criteria should be systematically analyzed:
- Functionality: The software must meet functional needs, addressing the specific forecasting challenges faced. Consider whether it supports various models and algorithms relevant to your datasets.
- Performance: Analyze the efficiency and speed of the software. Performance can significantly impact productivity and the time taken to derive insights.
- Community and Support: Look into the community around the software. A vibrant community often facilitates quicker problem resolution and enhances collaborative efforts.
- Compatibility: Ensure the chosen software can integrate with existing systems. Compatibility issues can lead to data silos and inefficiencies.
- Security: Evaluate the security features of the software. Understanding how it handles data protection is vital, especially with sensitive information.
User Experience and Interface
The usability of open source forecasting tools greatly affects user engagement and data quality. A well-designed user interface can simplify the forecasting process:
- Intuitive Design: Software should offer an easy-to-navigate interface, allowing users to focus on analyzing data rather than struggling with technology.
- Documentation: Quality documentation is essential. It helps new users understand how to utilize features effectively and troubleshoot when necessary.
- Visualization Tools: Effective visualizations aid in interpreting data. The presence of built-in charts and graphs can enhance the overall experience.
- Customization Options: Users should be able to tailor the interface to suit their needs. This flexibility is crucial for teams working on diverse projects.
The right evaluation process allows organizations to mitigate risks and enhance their forecasting capability.
By meticulously considering these criteria, organizations position themselves to select the most appropriate open source forecasting software, ultimately leading to improved analytical outcomes.
Challenges with Open Source Forecasting Software
Open source forecasting software comes with noteworthy advantages, but it also possesses distinct challenges. Understanding these obstacles is crucial for those who are serious about leveraging such systems. Overcoming these challenges can determine the effectiveness and efficiency of the software in real-world applications. Here are the central elements to consider:
Integration with Existing Systems
Integrating open source forecasting software into existing systems can emerge as a complex task. Organizations often operate with a diverse set of tools and platforms. The challenge lies in ensuring compatibility. Open source solutions may not always directly align with proprietary systems, leading to potential issues.
- Data Silos: If data is stored in disparate locations, merging it can be cumbersome.
- Workflow Disruptions: Integrating new tools can disrupt established workflows, which may hinder productivity temporarily.
- API Limitations: Open source software may not offer robust APIs, complicating the integration process.
Thus, careful planning is necessary before initiating implementation. Robust documentation from the developers of the open source software may facilitate smoother integration processes.
Learning Curve and Technical Skills
Another prominent issue is the learning curve associated with open source forecasting software. While being cost-effective and customizable, these platforms can require significant technical know-how. Not all organizations have staff equipped with the relevant skills.
- Skill Gaps: Employees may require training sessions to adapt to new open source tools, leading to initial productivity drops.
- Resource Allocation: The need for additional resources for training can strain small teams.
- Adaptability: Ensuring team members are adaptable to frequent updates and changes within the software can also be challenging.
Organizations might need to invest in training or hire new talent to address these gaps. Creating a supportive learning environment can help bridge the necessary skill sets.
Maintenance and Upgrades
Maintenance and upgrades present their own set of challenges in open source forecasting software. Unlike commercial solutions that often come with dedicated support teams, open source tools typically depend on community-driven updates and management.
- Inconsistency in Support: While community support can be valuable, it may lack consistency. Not all issues may receive prompt attention.
- Security Risks: Regular updates are necessary to safeguard against vulnerabilities. Organizations need a strategy to stay current with patches and new releases.
- Customization Costs: Custom modifications, while advantageous, can complicate maintenance. Keeping track of unique changes can be burdensome.
Thus, it is beneficial for organizations to develop a solid maintenance plan. This would ensure continuous integration of new features while keeping the system secure.
The key to successful open source forecasting is recognizing these challenges and actively working to mitigate them.
Case Studies of Successful Implementations
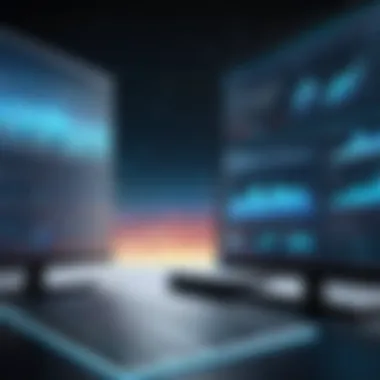
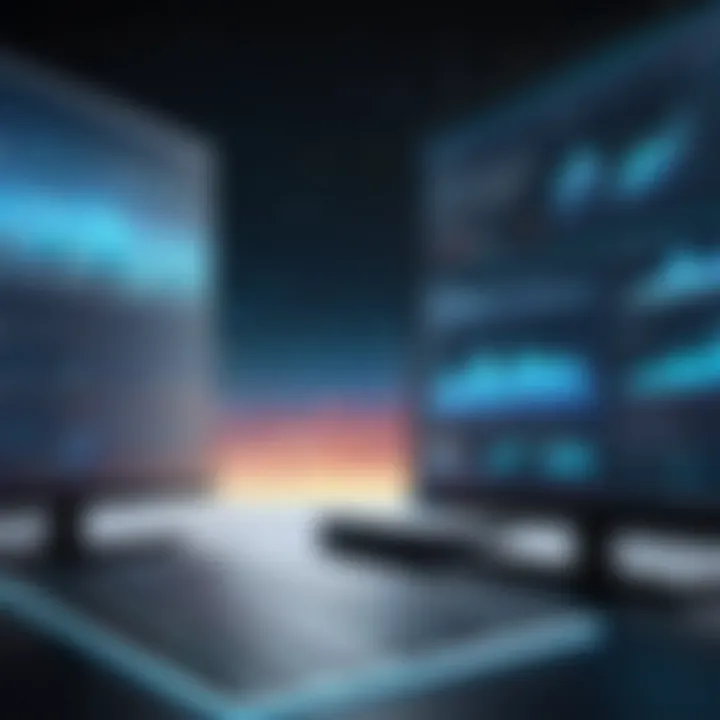
Case studies play a crucial role in understanding the impact and efficacy of open source forecasting software. They not only provide evidence of real-world applications but also illustrate how different industries have leveraged these tools to achieve measurable outcomes. Through detailed examples, organizations can assess the effectiveness of these solutions, learning from others’ experiences—both successes and challenges. This section delves into industry-specific applications of open source forecasting software and presents a comparative analysis of results, aiding in the decision-making process for future users.
Industry-Specific Applications
Open source forecasting software has found its place across various sectors, demonstrating adaptability and robustness in different contexts. Examples include:
- Retail Industry: Companies like Target have employed R and Python libraries for demand forecasting. These tools have enhanced their ability to predict customer needs, manage stock levels, and optimize supply chains, resulting in increased profitability and reduced waste.
- Healthcare Sector: The public health department in a major city used Apache PredictionIO to track disease outbreaks by forecasting the spread of illnesses. This implementation allowed for timely interventions, ultimately saving lives and resources.
- Finance Industry: Banks and financial institutions have utilized TensorFlow Forecasting to enhance risk assessment models. By integrating these models, they can better predict market trends and make informed investment decisions, fostering more stable financial practices.
By exploring these applications, we can understand how different organizations tailored solutions to fit their specific needs. Often, the flexibility of open source software enables them to customize the tools according to their operational requirements, fostering innovation at all levels.
Comparative Analysis of Results
A rigorous comparative analysis reveals how the implementation of open source forecasting software has led to varying degrees of success. Key metrics for assessment typically include:
- Accuracy of Predictions: Many organizations report significant improvements in their forecasting accuracy. For instance, a retail company noted a 20% increase in prediction accuracy after switching to open source tools.
- Cost Savings: Financial assessments have shown a considerable decrease in operational costs. Organizations leveraging open source software often find savings not only in software licenses but also in maintenance and customization expenses.
- User Engagement: Companies utilizing community-supported platforms report higher user satisfaction rates. This is partly due to the active discourse within communities, allowing for ongoing support and updates, making the transition smoother for users.
Comparing outcomes from diverse implementations helps illuminate best practices and highlight potential pitfalls in the adoption process. Many organizations acknowledge the steep learning curve; however, the long-term gains frequently outweigh initial challenges.
"Case studies provide a roadmap for decision-makers, illustrating what strategies lead to successes and how to avoid common errors. Understanding these lessons can dramatically inform future implementations."
With this comprehensive exploration of case studies, organizations can better appreciate the practical benefits and implications of adopting open source forecasting software.
Future Trends in Open Source Forecasting
The continuous advancements in technology propel the evolution of open source forecasting. There are several key factors shaping future trends in this field. Understanding these developments is crucial for software developers, professionals in IT-related fields, and students who rely on accurate predictions in various sectors.
One notable trend is the increasing integration of cloud computing. With this shift, open source forecasting software becomes more accessible and scalable. Users can harness the power of high-performance computing capabilities without significant hardware investments. This marks a significant change. It allows smaller companies to leverage sophisticated forecasting tools.
Another important aspect is the emphasis on interoperability. As organizations adopt multiple tools for their operational needs, open source forecasting software must communicate seamlessly with existing systems. This interconnectedness not only enables better data exchange but also enhances the overall workflow. Developers are encouraged to ensure their tools can integrate with popular platforms and databases.
Additionally, the rise of big data continues to influence forecasting methodologies. The ability to analyze vast amounts of data enables more accurate projections. Open source software must adapt to accommodate these data sets. This requires compatible frameworks and efficient algorithms for data processing.
"The future of open source forecasting lies not only in better accuracy and speed but also in fostering a collaborative ecosystem that enhances functionality across platforms."
Emerging Technologies and Methodologies
Emerging technologies are reshaping open source forecasting in profound ways. The proliferation of machine learning and advanced statistical approaches stands out as a pivotal development. These methodologies allow for more refined predictions by identifying patterns in historical data that were previously unnoticed.
Open source platforms are increasingly incorporating these techniques. For example, users can implement algorithms from programming languages like R and Python. These tools offer capabilities for time series forecasting and regression analysis, which improve accuracy.
Another significant trend is the increasing adoption of real-time data analytics. The capability to process and analyze data as it becomes available enhances forecasting agility. Professionals can respond more quickly to changes in the market or other variables. By incorporating real-time analytics, developers can create more dynamic and resilient forecasting tools.
The Role of Artificial Intelligence
Artificial Intelligence (AI) is revolutionizing open source forecasting. The algorithms driven by AI can learn from past data and adapt over time. This self-learning ability leads to increasingly sophisticated predictive models.
AI's influence extends to automation, where routine data processing tasks can be streamlined. This not only saves time but also reduces the margin for human error. For organizations, this results in improved decision-making based on more reliable data analysis.
Moreover, AI allows for enhanced user interaction with forecasting tools. With the introduction of natural language processing interfaces, users can query systems in plain language. This democratizes access to forecasting insights, making it easier for non-technical users to engage with complex data analysis.
The End
Open source forecasting software has shifted the landscape of analytics and decision-making across various domains. Its evolution has been marked by the integration of community-driven innovations and the introduction of advanced methodologies. These elements not only enhance forecasting models but also democratize the access to predictive analytics tools. The importance of the topic is accentuated when considering how businesses and organizations leverage these tools to make informed decisions, streamline operations, and improve outcomes.
The benefits of open source forecasting software are clear. It promotes cost efficiency, adaptability, and ongoing development due to community collaboration. It also opens avenues for customization, enabling organizations to tailor solutions to their specific needs. However, as organizations implement such systems, they must consider various factors. These include the integration process, user skill levels, and long-term maintenance.
As we move forward, understanding the dynamics of open source forecasting software will be crucial for professionals aiming to harness analytics effectively.
Recap of Key Points
- Historical Growth: Open source software has evolved significantly, enabling diverse forecasting capabilities.
- Flexibility and Customization: Users can modify software to suit their particular requirements.
- Community Support: Strong user communities contribute to continuous improvement and knowledge sharing.
- Challenges: Organizations face integration, technical skill gaps, and maintenance issues.
Final Thoughts on Adoption Strategies
For a successful adoption of open source forecasting software, an organization should follow certain strategies specifically:
- Assess Needs: Before selecting a tool, it's vital to clearly define forecasting requirements. Understanding the specific use cases will guide appropriate software selection.
- Encourage Training: Investment in training for users ensures that they feel confident working with open source tools. This can mitigate challenges posed by the learning curve.
- Evaluate Community Engagement: Active involvement in the community can provide insights into best practices and solutions for common issues.
- Plan for Maintenance: Have a maintenance plan in place, considering both software updates and user training needs post-implementation.
The emphasis on these strategies will support organizations in fully leveraging the power of open source forecasting software, leading to better analytical outcomes and strategic initiatives.